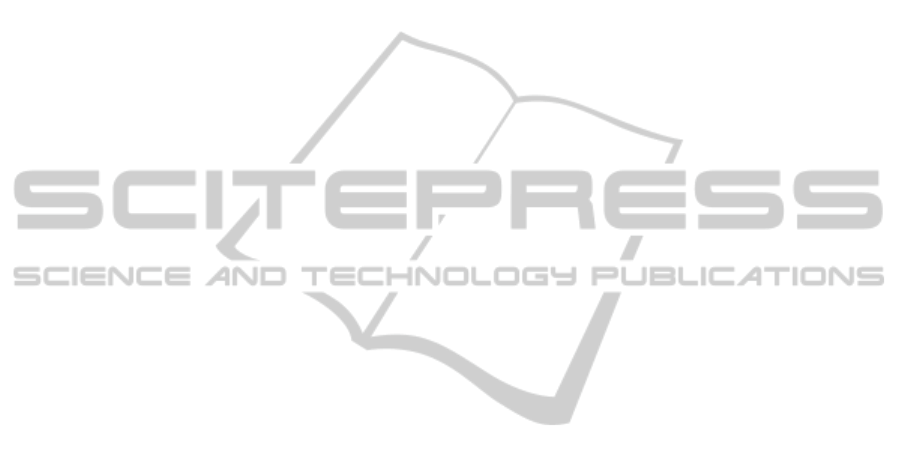
et al., 2008), a combination of SAC model follow-
ing and optimal H
∞
control is applied. The objec-
tive is to use SAC while satisfying some H
∞
-norm
bound γ. Sufficient conditions are derived for the sta-
bility and model following of the closed-loop dynam-
ics of the SAC scheme with disturbance attenuation
level γ. These sufficient conditions are expressed in
terms of Linear Matrix Inequalities (LMI), which can
be solved using Matlab’s LMI Toolbox (Gahinet et al.,
1995). A numerical flight control example is given
which illustrates the method.
1.1 Notation
Throughout the paper the superscript ‘T’ stands for
matrix transposition, R
n
denotes the n dimensional
Euclidean space, R
n×m
is the set of all n× m real ma-
trices, and the notation P > 0, for P ∈ R
n×n
, means
that P is symmetric and positive definite. T
zw
denotes
the transference from the exogenous disturbance w to
the objective function z, kT
zw
k
∞
is its H
∞
-norm and
kT
zw
k
2
is its H
2
-norm. col{a, b} stands for [a
T
b
T
]
T
and tr{H} denotes the trace of the matrix H.
2 PRELIMINARIES
2.1 Ideal Strictly Proper System and
Ideal Control
Consider the following continuous-time linear sys-
tem:
˙x
∗
(t) = Ax
∗
(t) + B
2
u
∗
(t), x
∗
(0) = 0
y
∗
(t) = C
2
x
∗
(t)
(1a,b)
where x
∗
(t) ∈ R
n
is the system state, y
∗
(t) ∈ R
l
is the
plant output which can be measured and u
∗
(t) ∈ R
m
is
the control input. A, B
2
and C
2
are constant matrices
of appropriate dimensions.
The output of the plant (1) is required to follow
the output of the asymptotically stable model:
˙x
m
(t) = A
m
x
m
(t) + B
m
u
m
(t), x
m
(0) = 0
y
m
(t) = C
m
x
m
(t)
(2a,b)
where x
m
(t) ∈ R
q
is the system state, y
m
(t) ∈ R
l
is
the plant output, u
m
(t) ∈ R
m
is the control input and
A
m
, B
m
and C
m
are constant matrices of appropriate
dimensions. The reference model (2) is used to define
the desired input-output behavior of the plant. It is
important to note that the dimension of the reference
model state may be less than the dimension of the
plant state. However, since y
∗
(t) is to track y
m
(t), the
number of the model outputs must be equal to number
of the plant outputs.
2.2 Hyper Minimum Phase Systems
Following (Fradkov, 2003), we introduce the follow-
ing notation:
δ(s) = det(sI
n
− A), G(s) = C
2
(sI
n
− A)
−1
B
2
.
Let T ∈ R
m×l
be off full row-rank and define
Ψ(s) = δ(s)det(TG(s)), Λ = TC
2
B
2
.
Definition 1. (Fradkov, 2003) The system (1) is
called minimum phase if the polynomial Ψ(s) is Hur-
witz (its zeros belong to the open left half-plane). It
is called Strictly Minimum Phase (SMP) if it is mini-
mum phase and detΛ 6= 0, and Hyper Minimum Phase
(HMP) if it is minimum phase and Λ > 0.
Remark 1. HMP is closely related to the ASPR for
square systems. For strictly proper square systems,
the conditions which define the ASPR property are
similar, and in such cases any minimum phase system
of m inputs and m outputs satisfying also C
2
B
2
> 0 is
ASPR (Kaufman et al., 1998).
Suppose that (1) closed with the feedback
u
∗
(t) = Ky
∗
(t) + v(t)
(3)
where K ∈ R
m×l
and v(t) is an auxiliary input. The
proof of the following Theorem can be found in
(Fradkov, 2003).
Theorem 1. The system (1) is strictly passifiable by
the output feedback (3) with fixed matrix K iff the sys-
tem (1) is HMP.
We will see in the sequel, as in (Kaufman et al.,
1998), that the HMP property allows applying a class
of direct adaptive controllers referred to as “simple
adaptive controllers”. In the sequel we assume that
the system (1) is HMP.
2.3 Perfect Following
Perfect Following (PF) is defined as following with
zero tracking error, namely
y
∗
(t) = y
m
(t)
The next lemma determines the relation that exists be-
tween the plant’s and the model’s state vectors.
Lemma 1. There exist F(t) ∈ R
n×q
and G(t) ∈
R
n×m
such that the trajectories of (1) are of the form:
x
∗
(t) = F(t)x
m
(t) + G(t)u
m
(t)
(4)
Proof: Equation (4) describes n equations with
n× (q + m) variables, thus the existence of F(t) and
G(t) is guaranteed for all 0 ≤ t < ∞. QED
ROBUST SIMPLE ADAPTIVE H8 MODEL FOLLOWING CONTROL DESIGN BY LMIS
339