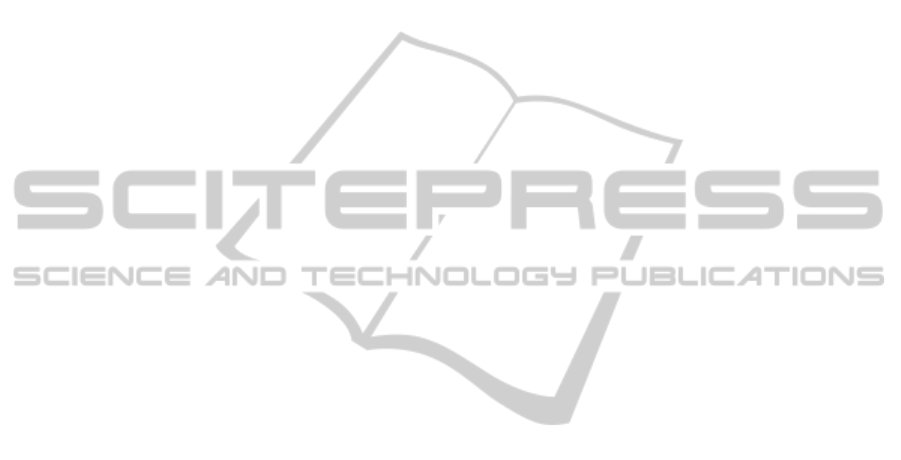
for an accurate foreground contour, a manually
obtained rough foreground region is used for each
frame in the evaluations, as shown in Fig. 4b.
Obviously, it is difficult to get accurate individual
detections based on the foreground area only.
The third row is the results based on shape cues
only. Most individuals can be located well based on
shape cues. However, cues based on shape may be
less reliable when the crowd is dense, the
background is complicated or other human shape-
like region appears. Hence, some false detections
may stay in the shape-based results, which are
indicated in green colour in Fig. 4c. The fourth row
is the results when motion consistency is also
considered. It can be seen that the false detections
indicated with the green colour in the third row have
been removed based on the coherent motion rule in
Fig. 4d. In the left column, three close persons have
got better detection results based on their different
trajectories. Similarly, in the middle column, a false
candidate covering two close persons has been
removed. Finally, the bottom row shows the results
when the upper-body rigid motion is also
considered. In the left column of Fig. 4e, a false
candidate with high trajectory variations in the upper
part has been removed. In addition, better human
size has been obtained for the persons indicated in
cyan colour in Fig. 4d.
5 CONCLUSIONS
In this paper, a method based on both shape and
motion features for crowd segmentation is presented.
The shape-based method has formulated the problem
into a feature point clustering process. Multi-frame
coherent motion of the feature points on a person is
used to enhance the segmentation performance.
Most feature points on the human upper-body are
moving together, which are used to get more
reasonable detections.
REFERENCES
Brostow, G. J. & Cipolla, R. 2006. Unsupervised Bayesian
Detection of Independent Motion in Crowds. IEEE
Conference on Computer Vision and Pattern
Recognition.
Dalal, N. & Triggs, B. 2005. Histograms of oriented
gradients for human detection. IEEE Conference on
Computer Vision and Pattern Recognition.
Dalal, N., Triggs, B. & Schmid, C. 2006 Human detection
using oriented histograms of flow and appearance.
European Conference on Computer Vision.
Hou, Y.-L. & Pang, G. K. H. 2009. Human Detection in a
Challenging Situation. IEEE International Conference
on Image Processing.
Hou, Y.-L. & Pang, G. K. H. 2010. Human Detection in
Crowded Scenes. IEEE International Conference on
Image Processing.
http://homepages.inf.ed.ac.uk/rbf/caviar/.
Lin, Z., Davis, L. S., Doermann, D. & Dementhon, D.
2007. Hierarchical Part-Template Matching for
Human Detection and Segmentation. IEEE
International Conference on Computer Vision.
Rabaud, V. & Belongie, S. 2006. Counting Crowded
Moving Objects. IEEE Conference on Computer
Vision and Pattern Recognition.
Rittscher, J., TU, P. H. & Krahnstoever, N. 2005.
Simultaneous estimation of segmentation and shape.
IEEE Conference on Computer Vision and Pattern
Recognition.
Shi, J. & Tomasi, C. 1994. Good features to track. IEEE
Conference on Computer Vision and Pattern
Recognition.
Tuzel, O., Porikli, F. & Meer, P. 2007. Human Detection
via Classification on Riemannian Manifolds. IEEE
Conference on Computer Vision and Pattern
Recognition.
Viola, P., Jones, M. J. & Snow, D. 2003. Detecting
pedestrians using patterns of motion and appearance.
IEEE International Conference on Computer Vision.
Wang, X., Han, T. X. & Yan, S. 2009. An HOG-LBP
Human Detector with Partial Occlusion Handling.
IEEE International Conference on Computer Vision.
Zhao, T. & Nevatia, R. 2004. Tracking multiple humans in
complex situations. IEEE Transactions on Pattern
Analysis and Machine Intelligence, 26, 1208-1221.
Zhao, T., Nevatia, R. & Wu, B. 2008. Segmentation and
Tracking of Multiple Humans in Crowded
Environments. IEEE Transactions on Pattern Analysis
and Machine Intelligence, 30, 1198-1211.
Zhu, Q., Yeh, M.-C., Cheng, K.-T. & Avidan, S. 2006.
Fast Human Detection Using a Cascade of Histograms
of Oriented Gradients. IEEE Conference on Computer
Vision and Pattern Recognition.
ICINCO 2011 - 8th International Conference on Informatics in Control, Automation and Robotics
178