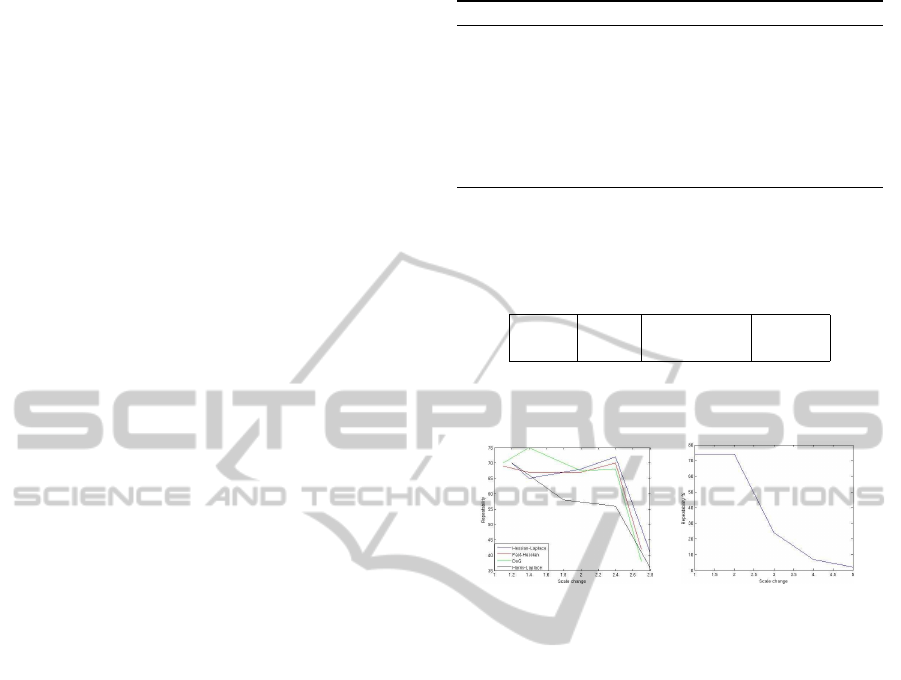
based method close to ours is presented in (Hahnel
and al, 2005); they also used a probabilistic sensor
model for their RFID reader; they associate the prob-
ability of tag detection with the relative position of
their tag. This model is used to map positions of pas-
sive RFID tags, considering the robot is located from
a previously learnt map through laser based SLAM.
Vorst and al (Vorst and Zell, 2010) have developped
a method of localisation on which the estimation is
based only on odometry and RFID measurements.
The technique requires no prior observation model
and makes no assumptions on the RFID setup. On the
other hand, using vision based approach, Zhou and
al (Zhou and al, 2007) proposed an indoor localization
method with modified active RFID tags, equipped
with LEDs which make the recognition much easier.
In (Ziparo and al, 2007), RFID tags are detected to
coordinate a team of robots for an exploration in un-
structured areas. In (Raoui and al, 2009), two strate-
gies are presented for metrical and topological navi-
gation with tags merged on shelves and on the ground.
Other researchers use vision based localization, but
the work of (Davison and al, 2007) is considered as a
turning point in the monoSLAM based navigation.
The fusion of many types of sensor data makes
more accurate the robot position. In (Deyle and
al, 2009), an RFID-enabled mobile manipulator can
grasp an object to which a self-adhesivepassive RFID
tag has been fixed; this new mode of perception pro-
duces a map of the spatial distribution of received sig-
nal strength indication for each of the tagged objects
in an environment.
3 GENERATING FEATURES
Our visual features (Raoui and al, 2009) are based on
the Harris detector because of its suitability in com-
putation time. We extend it to the colored images
by using the Gaussian color model(Geusebroek and
al, 2001). It is based on the second moment matrix
also called the auto correlation matrix. The detector
is made invariant to scale computing four characteris-
tic scales aroud each feature point, by the use of the
LoG operator (K.Mikolajzyk and C.Schmid, 2004).
The orientation for interest points is computed as
in (Lowe, 2004). It has good performances compar-
ing to other descriptors because it mixes localized in-
formation and the distribution of gradient related fea-
tures. Thus, for each scale the image is processed
to extract the orientation for the feature point and all
points around it ( 4 points). We concatenate these ori-
entations in the same descriptor. Then, we compute
the descriptor around each feature point (figure 2) by
Algorithm 1
1: for i← Feature Point-2 to Feature Point+2 do
2: box ← Create a box around a point i
3: result ← box * Gaussians(scales)
4: Compute norm(result)
5: V ← 4 highest values
6: Add V to the Feature Point
7: end for
using a set of 9 Gabor wavelets (Gabor, 1946). In or-
der to evaluate our detector and descriptor, we use the
repeatability score as suggested in (K.Mikolajzyk and
C.Schmid, 2004); a comparison is shown on figure 3.
x y σ r t
space scale orientation texture
Figure 2: Structure of our descriptor, 2 bin for space, 21 for
scale, 5 for orientation, 9 for texture.
Figure 3: Repeatability of Fast Hessian, DoG, Harris-
Laplace, Hessian-Laplace interest points detectors with re-
spect to scale(left).Repeatabilty of our detector descriptor
with respect to scale(right).
4 RFID-BASED ROBOT
LOCALIZATION
Our method for stochastic localization from RFID
Tags, is presented in (Duarte et al., 2010); it is based
on a particle filter in order to estimate the robot pose
from RFID observations. Such a filter represents the
state at step k by a random vector x
k
. Then a proba-
bility distribution over the position and orientation of
the robot x
k
, known as belief, Bel(x
k
) represents the
uncertainty of the state. In the particle filter scheme,
the belief function is represented by a set of n pairs
< x
k
i
,w
k
i
> defined by a position x and a weight w.
This weight is a measure of how much its related posi-
tion x represents the real robot position with respect to
the environment model. A function p(x
k
|x
k1
), known
as prediction function, and a correction function of w
k
must be defined. p(x
k
|x
k1
) models the dynamic of the
moving object, using here the odometry model. w
k
is
corrected depending on how much the actually sensed
data fit the position x
k
.
In order to maximize localization performances,
MOBILE ROBOT LOCALIZATION SCHEME BASED ON FUSION OF RFID AND VISUAL LANDMARKS
297