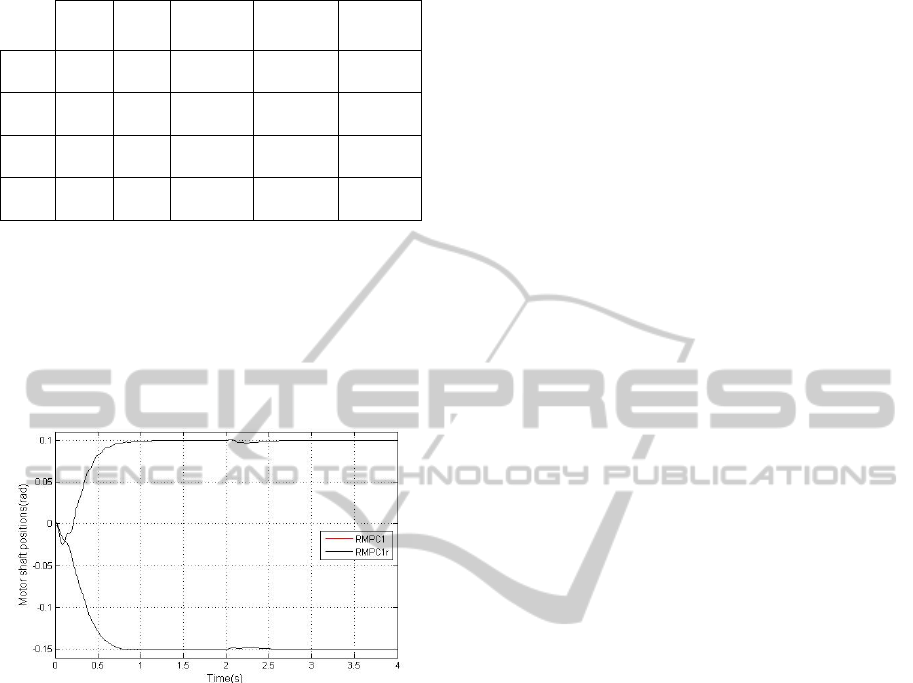
Table 1: Structured uncertainties robustness.
LQG MPC RMPC0 RMPC1
RMPC1r
Case 1
Case 2
Case 3
Case 4
Figure 12 illustrates the case where an
uncertainty of
%20 is considered on the motors
inertia:
mm
JJ %20 . Despite this uncertainty and
the nonlinearities of the system, the robustified
controller RMPC1 still stabilises the system.
Moreover, it can be observed that this property is
conserved even after the order reduction.
Figure 12: Output
m
q . Nonlinear model with
uncertainties of
mm
JJ %20
.
6 CONCLUSIONS
This paper proposes a comparison between
advanced control techniques for the control of the
angular position of a two axes model of a
cardiovascular robot, which is a strongly nonlinear
multivariable system. In order to improve the
controllers’ robustness, several layers of
robustification are further considered.
A linear quadratic controller (LQG) and a Model
Predictiv Control (MPC) law are first designed to
achieve similar level of performance for the time-
domain response. In a first step, additional
measurements of the joints accelerations are used in
order to increase the initial level of robustness of the
two controllers. Robust stability under unstructured
uncertainties is explicitly considered in the synthesis
of the robustified MPC controllers, while, for the
LQG controller, the robust stability under
unstructured uncertainties is verified a posteriori.
Simulation results show a trade-off between robust
stability and disturbances rejection.
The robustness towards the variation of some
parameters (i.e. structured uncertainties) is verified a
posteriori for all the considered controllers. An
interesting perspective is to take into account these
structured uncertainties during the synthesis of the
robustified MPC. A possibility is to consider a
polytopic uncertain domain around the nominal
model as in (Stoica et al., 2009) and to guarantee the
stability over the specified uncertain polytopic
domain solving a BMI (Biliniar Matrix Inequality)
optimisation problem.
REFERENCES
Al Assad, O., Godoy, E., Croulard, V., 2008. Macroscopic
drive chain efficiency modeling using state machines.
17th IFAC World Congress, Seoul, pp. 2294-2299.
Al Assad, O., Godoy, E., Croulard, V., 2007.
Irreversibility modeling applied to the control of
complex robotic drive chains. 4th ICINCO, Angers,
pp. 217-222.
Book, W. J., 1989. Modelling, design, and control of
flexible manipulators arms: status and trends. NASA
Conference on Space Telerobotics, vol. 3, pp. 11-24.
Boyd, S., Barratt, C., 1991. Linear controller design.
Limits of performance, Prentice Hall.
Boyd, S., Ghaoui, L. El., Feron, E., Balakrishnan, V.,
1994. Linear matrix inequalities in system and control
theory, SIAM Publications, Philadelphia.
Camacho, E. F., Bordons, C., 2004. Model predictive
control, Springer-Verlag. London, 2
nd
edition.
Clement, B., Duc, G., 2000. A multiobjective control via
Youla parameterization and LMI optimization:
application to a flexible arm, IFAC Symposium on
Robust Control and Design, Prague.
Doyle, J. C., Stein, G., 1979. Robustness with observers.
In IEEE Trans. Automatic Control, vol. AC 24, pp.
607-611.
Halalchi, H., Bara, G. I., Laroche E., 2010. LPV controller
design for robot manipulators based on augmented
LMI conditions with structural constraints, IFAC
Symposium on System, Structure and Control, Ancona,
Italy.
Hedjar R., Toumi, R., Boucher, P., Dumur, D., 2002.
Feedback nonlinear predictive control of rigid link
robot manipulators, IEEE ACC, Anchorage, AK,
USA, pp. 3594-3599.
Kučera, V., 1974. Closed loop stability of discrete linear
single variable systems, Kybernetika, vol. 10(2),
pp. 146-171.
Maalouf, A.I., 2006. Improving the robustness of a
parallel robot using Predictive Functional Control
ICINCO 2011 - 8th International Conference on Informatics in Control, Automation and Robotics
298