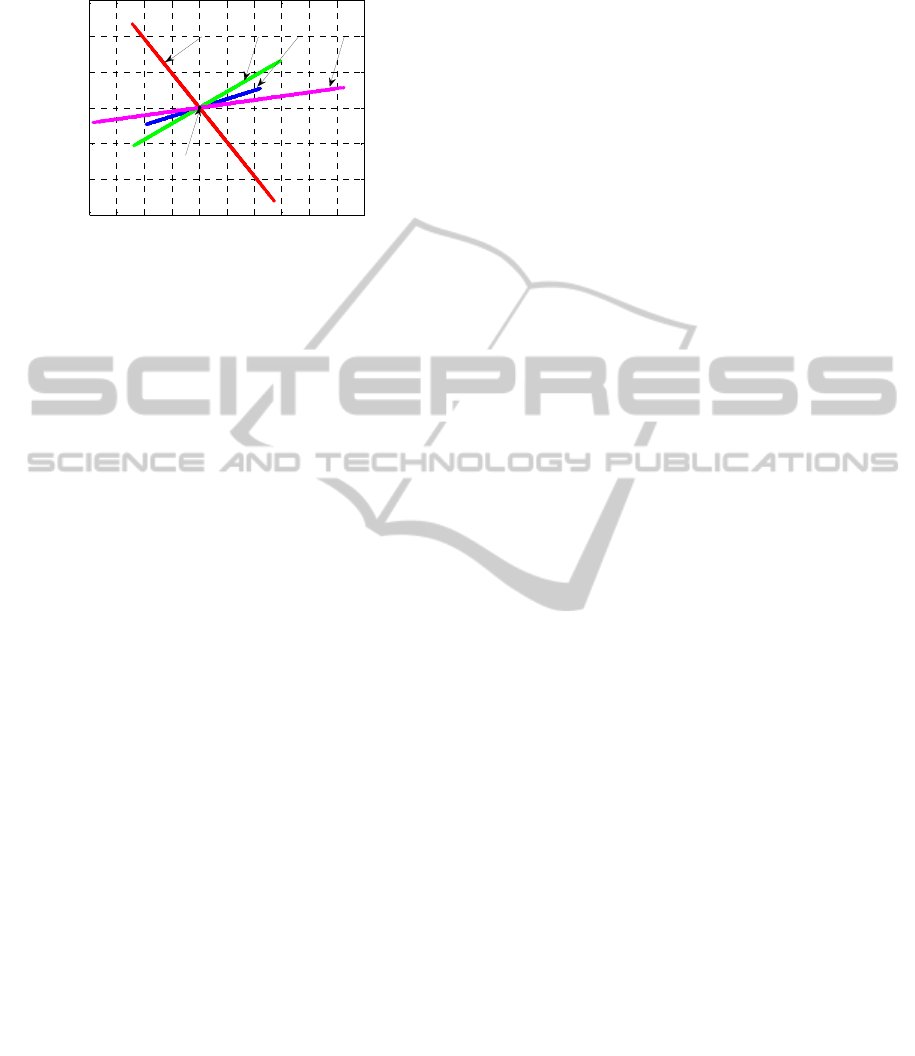
Figure 16: Variations of the rotor phase “a” current
residues versus time of the healthy and faulted WRIM.
Figure 17: Variations of electromagnetic torque residues
versus the shaft rotational speed residues of the WRIM.
4.3 Discussion
Several types of representations are used in the
signals processing domain, in particular for electrical
machines diagnosis. We can mention the temporal
representation (Fig. 8 to Fig.11, Fig. 15 and Fig. 17)
and the signal frequency analysis. Although they
have demonstrated their effectiveness, the state
variables representations between them also show
their advantages. They can be performed without
mathematical transformation (Fig. 12) and with
mathematical transformation (Fig. 13 and Fig. 14).
The latter representation type and the temporal
representation are confronted with the PCA method
application results (Fig. 15 to Fig. 17). Only the
simulation results with stator faults are presented
because the global behavior of the state variables in
both rotor and stator faults are almost similar.
For the temporal variations case, the rotor
currents (Fig. 9) and the shaft rotational speed (Fig.
10) are the variables which produce the most
information in presence of faults. The faults occur
on the rotor current frequency and the shaft
rotational speed magnitude.
Also, the electromagnetic torque variations
versus the shaft rotational speed clearly show the
WRIM operation zone in the presence of faults (Fig.
12). Contrary to this, the representations with
mathematical transformations (Fig. 13 and Fig. 14)
do not provide significant information due to the fact
that the stator currents remain almost unchanged in
the presence of faults (Fig. 8).
With PCA method application, all representation
types well show the differences between healthy and
faulted WRIM (Fig. 15 to Fig. 17). In the healthy
case, residues are zero. When faults appear, the
residue representations have an effective value with
an absolute value greater than zero.
In the figure 17, the healthy case is represented
by a point placed on the coordinate origins. Also,
one can show several right lines corresponding to
the faulted cases. This behavior is due to the
proportional characteristic of the considered faults.
PCA method proved so effective in electrical
machines faults detection. This requires a good
choice of the number of the principal components to
be retained so that information contained in residues
is relevant.
5 CONCLUSIONS
PCA method based on residues analysis has been
established and applied on WRIM diagnosis.
An accurate analytical model of the machine has
been proposed and simulated to performed the
healthy and faulted data for PCA approach need.
Several representations of nine state variables of
the machine have been analyzed. In the case of
temporal variation and without PCA, the rotor
current and the shaft rotational speed are the more
affected by the considered fault type. The
representations of the electromagnetic torque versus
the shaft rotational speed in both with and without
PCA approach show clearly the presence of faults.
Indeed, PCA method is interesting for all type of
representation compared to some other signal
processing types.
ACKNOWLEDGEMENTS
This research was supported by MADES/SCAC
Madagascar project. We are grateful for technical
and financial support.
REFERENCES
Wieczorek, M., Rosołowski, E., 2010. Modelling of
induction motor for simulation of internal faults,
Modern Electric Power Systems 2010, Wroclaw,
Poland MEPS'10 - paper P29.
Razik, H., 2002. Le contenu spectral du courant absorbé
par la machine asynchrone en cas de défaillance, un
état de l’art, La revue 3EI, n°29, pp. 48 - 52.
Chia-Chou, Y. & al., 2008. A Reconfigurable Motor for
Experimental Emulation of Stator Winding Interturn
and Broken Bar Faults in Polyphase Induction
Machines, IEEE transactions on energy conversion,
vol.23, n°4, pp. 1005-1014.
-0.4 -0.3 -0.2 -0.1 0 0.1 0.2 0.3 0.4 0.5 0.6
-0.4
-0.2
0
0.2
0.4
0.6
Electromagnetic torque
Shaft rotational speed
Healthy
40%10%20% 30%
ICINCO 2011 - 8th International Conference on Informatics in Control, Automation and Robotics
174