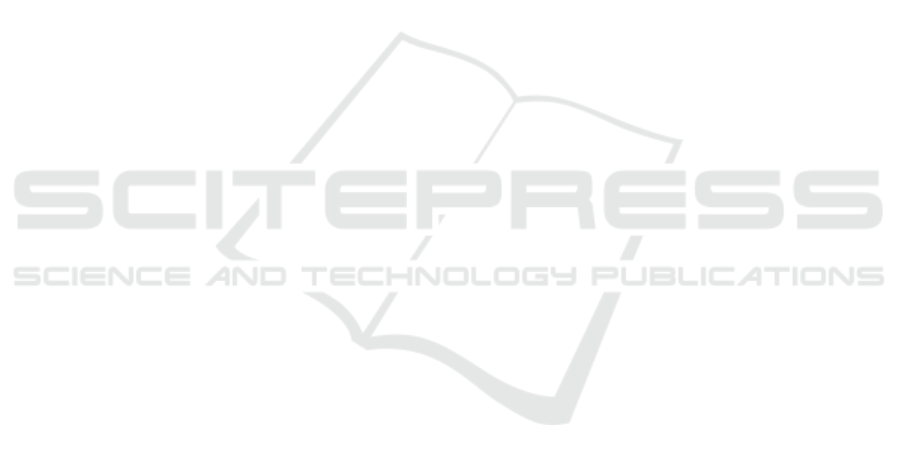
A WIDELY INFINITE PAST PREDICTION PROCEDURE
Jes´us Navarro-Moreno, Rosa M. Fern´andez-Alcal´a, Juan C. Ruiz-Molina and Antonia Oya
Department of Statistics and Operations Research, University of Ja´en, 23071 Ja´en, Spain
Keywords:
Prediction theory, Transfer function models, Widely linear processing.
Abstract:
Using a widely linear (WL) processing, a prediction algorithm has been designed for WL transfer function
models in terms of an infinite number of past observations. This algorithm leads to a suboptimum infinite
past predictor which approximates the optimal predictor based on a finite past information when the size of
the series goes to infinite. Hence, the applicability of our solution lies in those situations where the predictor
based on a finite past is difficult to obtain.
1 INTRODUCTION
Prediction based upon an infinite number of past ob-
servations is a problem of great relevance in statistical
communication theory. Specifically, in those situa-
tions where the predictor based on finite past is diffi-
cult to obtain because of the number of available ob-
servations is extremely large, infinite past prediction
problemsprovidefeasible recursivealgorithmsfor the
computation of a suboptimum estimate which approx-
imates the finite past predictor optimally [see, for ex-
ample, (Brockwell and Davis, 1991)].
In particular, this strategy has been widely used
in transfer function models (Box and Jenkins, 1970;
Brockwell and Davis, 1991). Transfer function mod-
els, also called dynamic regression models, are exten-
sions of familiar linear regression models which in-
clude not only information related to the past of the
time series of interest but also the present and past
values of other time series. Thus, the prediction of
the first time series may be considerably improved by
using information coming from the second.
On the other hand, the widely linear (WL) pro-
cessing has provided a new perspective for solving
several problems concerned with noncircular or im-
proper complex-valued time series. This approach,
based on the information supplied by both the sig-
nal and its conjugate, has shown its efficiency against
the conventional or strictly linear (SL) processing in
many areas of statistical signal processing such as
modeling and estimation, among others [see, e.g.,
(Mandic and Goh, 2009; Navarro-Moreno, 2008;
Navarro-Moreno et al., 2009; Picinbono and Cheva-
lier, 1995; Picinbono and Bondon, 1997)]. Indeed,
in the modeling field, WL systems appear to be more
suitable than SL systems in the representation of this
type of signal. In this framework, the WL finite past
prediction problem for WL ARMA models has been
studied in (Navarro-Moreno, 2008).
This paper tackles the WL infinite past prediction
problem for a more general WL system than the one
considered in (Navarro-Moreno, 2008). Specifically,
the time series of interest is assumed to be modeled
by a WL transfer function system and thus, following
a WL processing, a recursive prediction algorithm is
devised from the infinite past information supplied by
both the input and output of such a model. This al-
gorithm becomes an alternative approach to the WL
finite past prediction problem of this type of system
which, in general, is difficult to address. For this pur-
pose, we first introduce WL transfer function models
in Section 2. Next, the WL infinite past prediction
problem is addressed in Section 3. Finally, an illus-
trative example is developed in Section 4.
2 WL TRANSFER FUNCTION
MODELS
To start with, we introduce some important notations
that will be used throughout the paper.
The real part of a complex number will be denoted
by ℜ{ ·}, the transpose of a vector by (·)
′
, the complex
conjugate by (·)
∗
and the conjugate transpose by (·)
H
.
In general, we will consider the augmented version
X
t
= [X
t
, X
∗
t
]
′
of the complex-valued random process
X
t
.
441
Navarro-Moreno J., M. Fernández-Alcalá R., C. Ruiz-Molina J. and Oya A..
A WIDELY INFINITE PAST PREDICTION PROCEDURE.
DOI: 10.5220/0003544704410444
In Proceedings of the 8th International Conference on Informatics in Control, Automation and Robotics (ICINCO-2011), pages 441-444
ISBN: 978-989-8425-74-4
Copyright
c
2011 SCITEPRESS (Science and Technology Publications, Lda.)