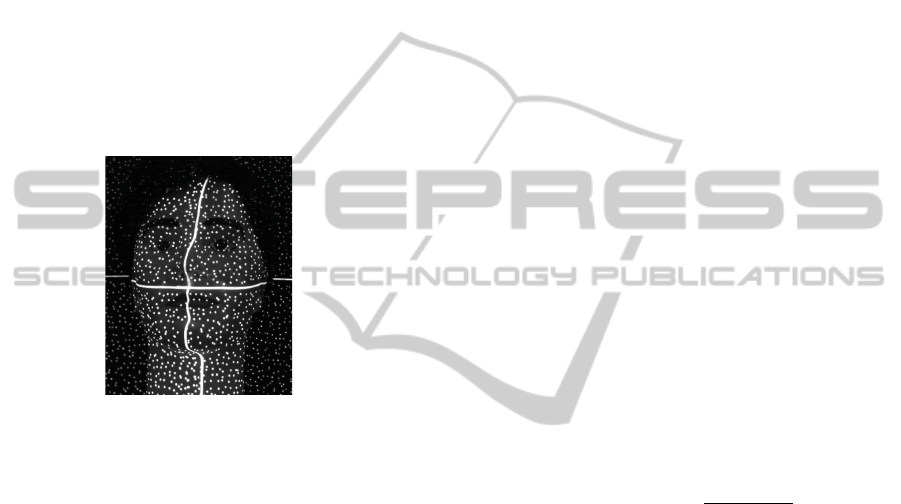
surface of interest there is only one consistent and
physical depth value on which every stereo pair in
the multi-camera setup must agree, and this per-
mits the identification of correspondingspots between
multiple images. The multi-camera setup in this
work comprises 4 cameras, which are calibrated us-
ing Bouguet’s Camera Calibration Toolbox for Mat-
lab (Bouguet, 2010).
Spot Pattern Extraction: A simple, single pattern
composed of centered horizontal and vertical stripes,
spanning from one border to the other, and binary dots
is projected on the object of interest, as shown in Fig-
ure 1. The stripes delineate quadrant sets of dots al-
lowing each quadrant to be processed separately and
inhibiting every dot matching error from propagating
into other quadrants.
Figure 1: Projection of the light pattern onto the object of
interest, as acquired from one of the cameras.
The spot pattern is extracted by binarising the im-
ages of the object and the projected light pattern, and
filling any holes in the spots. The centroid of each ex-
tracted spot is then found and used to represent the ap-
proximate location of each projected spot. This yields
the images f
(u)
i
for any spot quadrant, where camera
index i = 1, . . . , n, and superscript u denotes unrecti-
fied images.
Spot Matching: In order to generate a sparse re-
construction of the object of interest, corresponding
spots between multiple stereo pairs need to be iden-
tified. Contrary to the active techniques reviewed
in Section 1, which rely on coded patterns to iden-
tify correct pixel correspondences, the proposed algo-
rithm exploits the redundant data in multiple stereo
images to match each spot in the uncoded binary pat-
tern. This ensures that the method is unaffected by
the underlying surface colour and is not susceptible
to surface depth discontinuities. The spot matching
procedurefirst involves identifying candidate matches
and then identifies the most likely match on the basis
of range consistency.
In order to identify the possible matching spots in
the pattern, the binary images are first rectified with
respect to a reference camera i according to (Fusiello
et al., 2000), in order to reduce the search problem
for correspondences to a 1-dimensional search along
epipolar lines. This yields the pairs of images, f
(r(i, j))
i
and f
(r(i, j))
j
for cameras indexed i and j respectively,
where j = 1, . . . , n, j 6= i, with a particular rectifica-
tion r(i, j) between camera pair i, j. The spots in im-
ages f
(r(i, j))
i
and f
(r(i, j))
j
are also arbitrarily assigned a
unique index value k
(i)
and k
( j)
respectively, for iden-
tification purposes.
Now, consider the spot indexed k
(i)
. Its true match
must theoretically lie on the epipolar line in image
f
(r(i, j))
j
. Therefore, the indices of the spots residing
on the epipolar line are included in the set, C
j
(k
(i)
) =
{k
( j)
1
, k
( j)
2
, . . . , k
( j)
N
} = {k
( j)
η
, 1 ≤ η ≤ N}, of candidate
matching dots for the spot with index, k
(i)
. In addi-
tion, spots residing on a number of rows above and
below the epipolar line are also included in the set of
candidate matches, since the estimation of each spot
location may shift the true match off the epipolar line.
Now, each candidate match is assigned a score and
a depth value. The score value was chosen to be a lin-
early decreasing function of distance from the epipo-
lar line. The depth value Z
(r(i, j))
(k
(i)
;k
( j)
η
) is calcu-
lated by triangulation between the reference spot with
index value, k
(i)
, and each candidate match, k
( j)
η
, in
C
j
(k
(i)
) as described by Equation 1.
Z
(r(i, j))
(k
(i)
;k
( j)
η
) =
B
i, j
F
(r(i, j))
d(k
(i)
, k
( j)
η
)
(1)
where, η = 1, . . . , N, B
i, j
denotes the baseline length
of the stereo pair (i, j), F
(r(i, j))
denotes the common
focal length of cameras i and j, and d(k
(i)
, k
( j)
η
) is the
disparity value in pixel units between spot index k
(i)
in f
(r(i, j))
i
and the candidate matching spot index k
( j)
η
in f
(r(i, j))
j
.
Since the depth value of the true match must be
consistent between all stereo pairs, this true match can
be identified by seeking that candidate match that has
the same depth value in all stereo pairs. However,
any inaccuracies in the camera calibration parameters
and the inaccurate approximation of each spot loca-
tion may cause the depth values of the true match
to vary by some value between different stereo pairs.
To counteract this discrepancy and identify the true
match, a weighted histogram of the depth values of
each candidate match is generated, where each can-
didate match is weighted by its score value forming a
histogram such as shown in Figure 2. A separate map-
ping table is also used to retain the relationship be-
ICINCO 2011 - 8th International Conference on Informatics in Control, Automation and Robotics
318