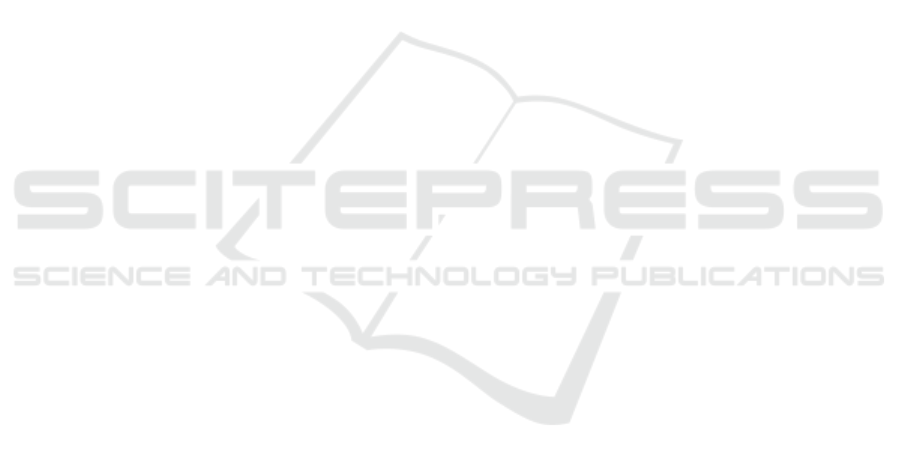
MUTUAL INTERDEPENDENCE OF STOCK MARKETS
BASED ON SUPPORT VECTOR MACHINE
Minghao Zhu and Jie Li
School of Economics and Management, Beijing Jiaotong University, Beijing, China
Keywords: Stock index, Mutual prediction, Nonlinear dependence, Support Vector Machine.
Abstract: China's market economy continues to advance, which makes the transparency of information of stock
market increasing, the information between the stock market flows faster, a variety of interactions between
the stocks increasingly significant. In this paper, support vector machine method is used to study the stock
market in the nonlinear discontinuous time series, through the establishment of different support vector
machine model, respectively to predict for the Shanghai A shares index, the Shenzhen A share index, the
Shanghai B share index and Shenzhen B share index, analyze their absolute error and relative error, it was
found there is a strong nonlinear interdependence in the same stock market and a strong dependence of
different securities markets, the Shanghai index has a larger effect compare to the Shenzhen index slightly.
1 INTRODUCTION
By studying the interaction between the predictive
ability of stock to analyze the interdependence of the
stock market, stock prediction is the use of mutual
support vector machines for the training data using
different indexes to predict changes in other stock
indices open space and trends, through analysis the
error between models to study the predicted
relationship between stock index, which can analyze
the dependence between the stock market, mainly to
describe the mutual coordination of the stock index
between the various elements of the situation is good
or bad, to measure the phase transition
characteristics and laws between the two indexes.
According to the basic principles of coordination
theory, the coordination degree determines which
sequence and structure the system will reach when it
comes to a critical region or the trend from
disorderly to orderly.
The main methods of researches between stocks
are stock co-integration (Daimin, 2002; Yu et al.,
2004; Guang and Yang, 2010; Xiyu and Yufang,
2004) and prediction
(Pi-e and Yanhua, 2000; Ping
et al., 2003; Xing et al., 2001; Yuchuan and
Zuoquan, 2007). Co-integration analysis is used to
study relationship and co-integration of the stock
index, futures, options, mutual stock fluctuations and
so on, by the methods of ADF, Johansen, Granger
Test, but all of these methods cannot describe the
nonlinear characteristics. There is a lot of studying
on a short-term or long-term prediction of a stock
index, but less about mutual prediction. Support
Vector Machine (SVM) theory is based on statistical
learning theory, and the approximately realize of the
minimization structural risk, the effective prediction
of stock index by the method of SVM regression
prediction gives powerful message about the overall
change in the stock market, which makes sense for
index prediction. The mutual authentication and
prediction of different stock index as the training
data gives us a new method to understand the
relevance and synergy of securities market change.
China's stock market is still in "weak efficient
market", which is not a simple linear and orderly
market; it is the financial market with complex
nonlinear characteristics. Not only investors is
irrationality with overreaction occurs or lack of
reflect, but also the market is often unstable. With
the internal features of nonlinear, discontinuous, the
time-series of the stock market and SVM methods
have similar characteristics. The paper establishes
SVM neural network model, with the Shanghai A
Share Index and Shenzhen A Share Index as the
training data, then it analyzes other indexes
(including the Shanghai A Shares Index and
Shenzhen A Shares Index) for regression predict. By
analyzing the predicted results and the forecast error,
we study the correlation and collaboration between
218
Zhu M. and Li J..
MUTUAL INTERDEPENDENCE OF STOCK MARKETS BASED ON SUPPORT VECTOR MACHINE.
DOI: 10.5220/0003552702180221
In Proceedings of the 13th International Conference on Enterprise Information Systems (ICEIS-2011), pages 218-221
ISBN: 978-989-8425-54-6
Copyright
c
ξ 2011 SCITEPRESS (Science and Technology Publications, Lda.)