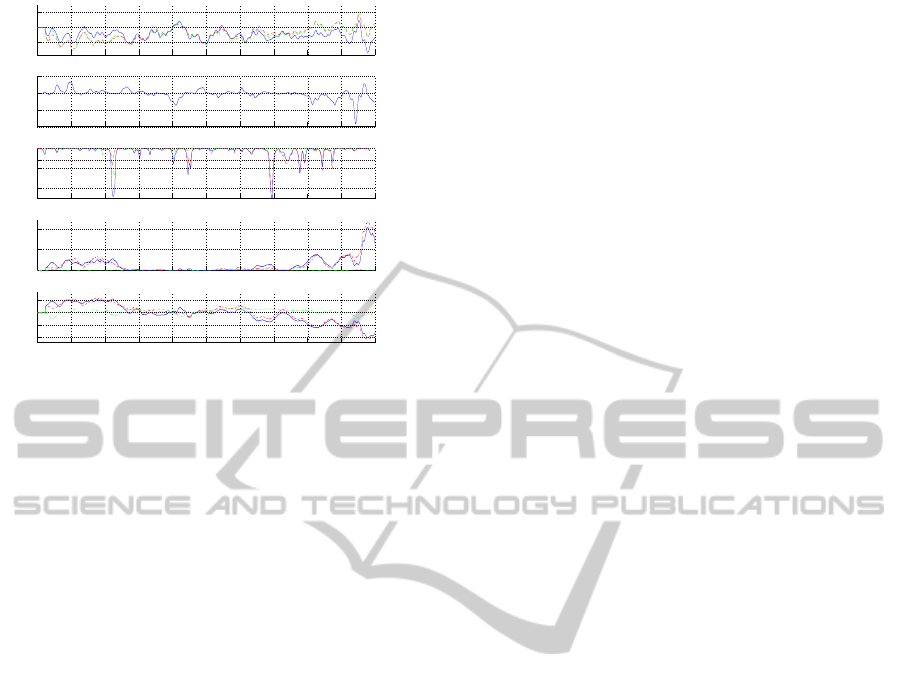
−0.1
0
0.1
(a)
Level mean [m]
−0.06
−0.03
0
0.03
(b)
d
Eu
0
0,5
1
(c)
K
i,j
0
0.005
0.01
(d)
ε
2
i,j
0 1 2 3 4 5 6 7 8 9 10
x 10
4
−0.1
−0.05
0
0.05
(e)
ε
i,j
Time [step]
Figure 7: (a) Measured levels x
c
(continuous line), x
a
(dot-
ted line) and x
f
(dashed-dotted line), (b) Euclidienne dis-
tance d
Eu
(M
e
,M
n
), (c) correlation indicators K
c,a
(continu-
ous line), K
c, f
(dashed line) and K
a, f
(dashed-dotted line),
(d) quadratic error indicators ε
2
c,a
(continuous line), ε
2
c, f
(dashed line) and ε
2
a, f
(dashed-dotted line), (e) error in-
dicators ε
c,a
(continuous line), ε
c, f
(dashed line) and ε
a, f
(dashed-dotted line), measured on 2006.
der to reach these objectives. The technique of super-
vision which is presented in this article is based on
the Pattern recognition AUDyC algorithm. It has the
advantage to limit physical knowledge of the system,
and aims to modelling the operating modes of dynam-
ical systems using only measured data. The charac-
teristics of the operating mode are updated in real-
time in order to follow the drifts due to sensor faults,
and detect setting errors and measurement or trans-
mission errors. The proposed technique is applied on
a real hydrographical system with presents the partic-
ularities to not being modelled according to classical
modelling methods. Fault indicators are determined
according to levels which are measured since 2006.
The first obtained results highlight the efficiency of
the proposed fault detection method. However, these
results have to be improved. The futur purposes con-
sist in proposing more pertinent fault indicators by
considering the measured upstream and downstream
in the Cuinchy-Fontinettes channel. It should be also
interesting to take into account the unknown inputs
which correspond to overflows in the channel. In fu-
ture works, a prognosis approach will be proposed to
predict the future state of the level sensors in order to
detect as soon as possible sensor faults. Finally, an
implementation of the proposed technique on the real
system may be considered at term.
REFERENCES
Akhenak, A., Chadli, M., Ragot, J., and Maquin, D. (2004).
State estimation of uncertain multiple model with un-
known inputs. In 43rd IEEE Conference on Decision
and Control, Atlantis, Bahamas, page 35633568.
Bedjaoui, N. and Weyer, E. (2010). Algorithms for leak de-
tection, estimation, isolation and localization in open
water channels. Control Engineering Practice, In
Press.
Chow, V. T., Maidment, D. R., and Mays, L. W. (1998).
Applied Hydrology. McGraw-Hill.
Eltoft, T. and de Figueiredo, R. (1998). A nez neural net-
work for cluster-detection-and-labeling. IEEE Trans.
Neural Networks, 9:1021–1035.
Frank, P. M., Ding, S. X., and KCipper-Seligcr, B. (2000).
Current developments in the theory of fdi. In SAFE-
PROCESS00, Budapest, Hungary, pages 16–27.
Gertler, J. (1998). Fault Detection and Diagnosis in Engi-
neering Systems. Dekker.
Hartert, L., Mouchaweh, M. S., and Billaudel, P. (2010).
Intelligent Industrial Systems: Modeling, Automation
and Adaptive Behavior. IGI.
Lecoeuche, S., Lurette, C., and Lalot, S. (2004). New su-
pervision architecture based on on-line modeling of
non-stationary data. Neural Computing and Applica-
tions Journal, 13:323–338.
Mouchaweh, M. S., Devillez, A., Lecolier, G., and Bil-
laudel, P. (2002). Recursive learning in real time using
fuzzy pattern matching. Mathematics and Computers
in Simulation, 60:209–216.
Su, M.-C. and Liu, Y.-C. (2005). A new approach to clus-
tering data with arbitrary shapes. Pattern Recognition,
38:1887–1901.
Traore, M., Duviella, E., and Lecoeuche, S. (2009). Dy-
namical clustering technique to estimate the proba-
bility of the failure occurrence of process subjected
to slow degradation. In ICINCO, Milan, Italy, pages
636–643.
Weihua, L., Harigopal, R., and Sirish, S. (2003). Subspace
identification of continuous time models for process
fault detection and isolation. Journal of Process Con-
trol, 13:407–421.
Xie, L., Soh, Y. C., and de Souzi, C. E. (1994). Ro-
bust kalman filtering for uncertain discrete-time sys-
tems. IEEE Transaction on Automatic Control, 93:131
01314.
SENSOR FAULT DETECTION IN A REAL HYDRAULIC SYSTEM USING A CLASSIFICATION APPROACH
387