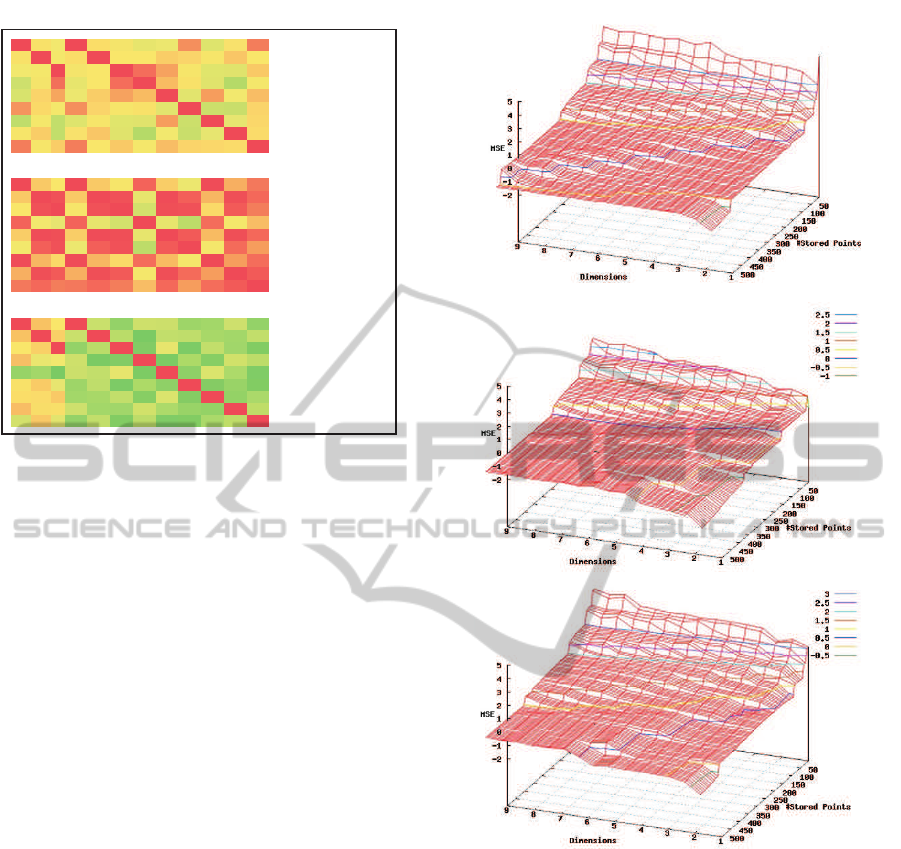
1,0 0,7 0,6 0,5 0,6 0,9 0,5 0,7 0,9
0,7 1,0 0,7 0,7 0,7 0,7 0,7 0,7 0,7
0,6 0,7 1,0 0,9 0,8 0,7 0,5 0,5 0,7
0,5 0,7 0,9 1,0 0,8 0,7 0,5 0,3 0,6
0,6 0,7 0,8 0,8 1,0 0,5 0,8 0,6 0,8
0,9 0,7 0,7 0,7 0,5 1,0 0,4 0,4 0,7
0,5 0,7 0,5 0,5 0,8 0,4 1,0 0,5 0,7
0,7 0,7 0,5 0,3 0,6 0,4 0,5 1,0 0,7
0,9 0,7 0,7 0,6 0,8 0,7 0,7 0,7 1,0
1,0 0,7 0,7 1,0 0,7 0,6 1,0 0,8 0,9
Dependent
0,7 1,0 1,0 0,5 1,0 1,0 0,8 1,0 0,9
0,7 1,0 1,0 0,4 1,0 1,0 0,7 1,0 0,9
1,0 0,5 0,4 1,0 0,5 0,3 0,9 0,6 0,8
0,7 1,0 1,0 0,5 1,0 1,0 0,8 1,0 0,9
0,6 1,0 1,0 0,3 1,0 1,0 0,6 0,9 0,8
1,0 0,8 0,7 0,9 0,8 0,6 1,0 0,8 0,9
0,8 1,0 1,0 0,6 1,0 0,9 0,8 1,0 1,0
0,9 0,9 0,9 0,8 0,9 0,8 0,9 1,0 1,0
1,0 0,4 0,1 0,4 -0,4 0,2 0,2 0,4 -0,1
Independent
0,4 1,0 0,3 0,0 -0,3 0,3 0,2 0,2 0,3
0,1 0,3 1,0 0,0 -0,5 0,0 0,3 0,2 0,0
0,4 0,0 0,0 1,0 0,0 0,2 0,1 0,4 -0,3
-0,4 -0,3 -0,5 0,0 1,0 0,1 -0,5 -0,2 0,0
0,2 0,3 0,0 0,2 0,1 1,0 0,0 -0,1 0,0
0,2 0,2 0,3 0,1 -0,5 0,0 1,0 0,1 0,2
0,4 0,2 0,2 0,4 -0,2 -0,1 0,1 1,0 -0,3
-0,1 0,3 0,0 -0,3 0,0 0,0 0,2 -0,3 1,0
1,0 0,7 0,6 0,5 0,6 0,9 0,5 0,7 0,9 SemiDependent
0,7 1,0 0,7 0,7 0,7 0,7 0,7 0,7 0,7
0,6 0,7 1,0 0,9 0,8 0,7 0,5 0,5 0,7
0,5 0,7 0,9 1,0 0,8 0,7 0,5 0,3 0,6
0,6 0,7 0,8 0,8 1,0 0,5 0,8 0,6 0,8
0,9 0,7 0,7 0,7 0,5 1,0 0,4 0,4 0,7
0,5 0,7 0,5 0,5 0,8 0,4 1,0 0,5 0,7
0,7 0,7 0,5 0,3 0,6 0,4 0,5 1,0 0,7
0,9 0,7 0,7 0,6 0,8 0,7 0,7 0,7 1,0
1,0 0,7 0,7 1,0 0,7 0,6 1,0 0,8 0,9
Dependent
0,7 1,0 1,0 0,5 1,0 1,0 0,8 1,0 0,9
0,7 1,0 1,0 0,4 1,0 1,0 0,7 1,0 0,9
1,0 0,5 0,4 1,0 0,5 0,3 0,9 0,6 0,8
0,7 1,0 1,0 0,5 1,0 1,0 0,8 1,0 0,9
0,6 1,0 1,0 0,3 1,0 1,0 0,6 0,9 0,8
1,0 0,8 0,7 0,9 0,8 0,6 1,0 0,8 0,9
0,8 1,0 1,0 0,6 1,0 0,9 0,8 1,0 1,0
0,9 0,9 0,9 0,8 0,9 0,8 0,9 1,0 1,0
1,0 0,4 0,1 0,4 0,4 0,2 0,2 0,4 0,1
Independent
0,4 1,0 0,3 0,0 0,3 0,3 0,2 0,2 0,3
0,1 0,3 1,0 0,0 0,5 0,0 0,3 0,2 0,0
0,4 0,0 0,0 1,0 0,0 0,2 0,1 0,4 0,3
0,4 0,3 0,5 0,0 1,0 0,1 0,5 0,2 0,0
0,2 0,3 0,0 0,2 0,1 1,0 0,0 0,1 0,0
0,2 0,2 0,3 0,1 0,5 0,0 1,0 0,1 0,2
0,4 0,2 0,2 0,4 0,2 0,1 0,1 1,0 0,3
0,1 0,3 0,0 0,3 0,0 0,0 0,2 0,3 1,0
Figure 5: Examples for the Spearman rank correlation for
each of the designed datasets shown in a correlation matrix.
Green colors depict a low correlation and red colors stand
for a high correlation between the parameter pairs.
effect the analysis was repeated with 100 different
datasets each.
The results for the compression of one dimension
is similar for all datasets.
From figure 6 it can be seen, that with increasing
dimensions the amount of PIPs required to achieve
the same goodness of fit is exponentially larger in the
case of the independent processes.
6 CONDITION MONITORING
CASE STUDY ON TWO REAL
DATASETS
Considering datasets from real systems it is important
to keep the findings of the previous section in mind.
It is crucial only to compress multivariate datasets if
the individual signals have significant stochastic de-
pendencies with each other. In case these stochas-
tic dependencies do not exist, it is possible to iso-
late groups of highly dependent signals. In this man-
ner a dataset can be split into a number of subset
datasets which each contain the required amount of
data. These groups of dependent signals are also very
beneficial for the application of a condition monitor-
ing software (Feller and Chevalier, 2010).
The multivariate extension of the perceptually im-
portant points algorithm was applied to two case study
datasets. The first dataset originates from a gas tur-
bine and the second comes from an agricultural ve-
hicle. The dataset from the gas turbine consists of
SemiDependent
Dependent
Independent
Figure 6: The three graphs show the logarithm of MSE ver-
sus the number of dimensions compressed and the number
of PIPs selected for linear approximation. The top most
graph results from the compression of the artificial dataset
2 which has a medium dependency between different di-
mensions. The center graph results from the third artifi-
cial dataset. Finally the bottom graph results from the first
dataset with the lowest dependency. The colored lines in
each graph depict levels of equal goodness of fit. For each
graph the same scales and view were used. To smooth out
any random effects the analysis was repeated 100 times and
the results were averaged.
5000 samples, each containing 120 different param-
eters. The parameters include mechanical and ther-
modynamical variables. The dataset from the agricul-
tural vehicle contains 3750 samples, each with 130
different parameters. The parameters primarily con-
tain mechanical variables, such as vibrations. Fig-
ure 8 summarizes the compression progress for both
OPTIMIZED STRATEGIES FOR ARCHIVING MULTI-DIMENSIONAL PROCESS DATA - Building a Fault-diagnosis
Database
391