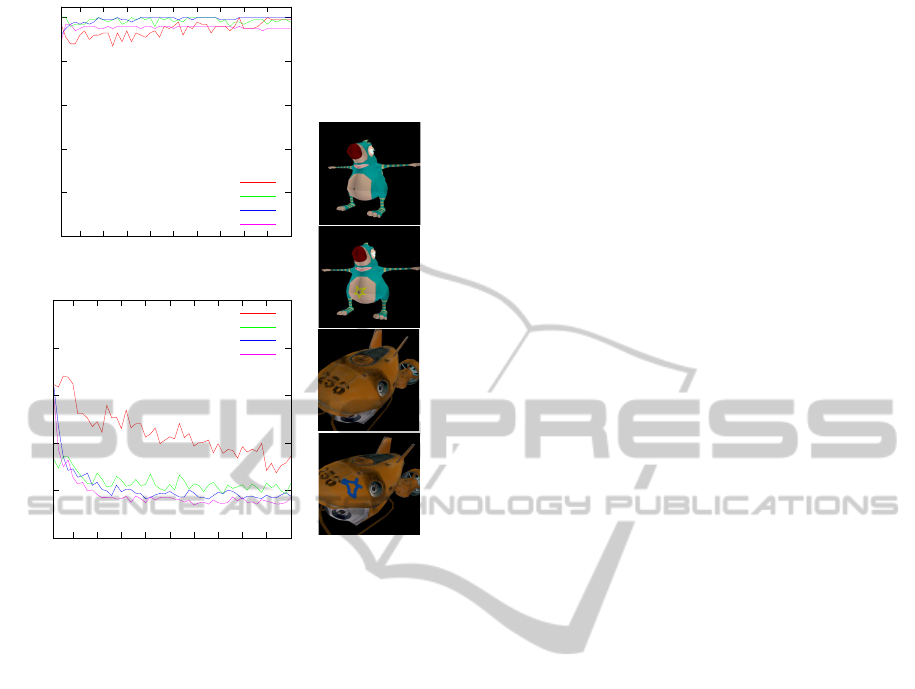
!
" !
# !
$ !
Figure 5: Example learning curves. The top graph shows
the averaged cumulative rewards during the course of 50
episodes. The bottom graph shows the averaged number of
steps it took the agent to reach the goal. The objects the
agent had to recognize are shown on the right. Additional
to the submarine models, two dragon models where used
which differ in the presence or absence of a yellow star sur-
rounding the belly button. The threshold values used for the
certainty score are given in the diagram legends. The results
were averaged over 100 runs.
5 CONCLUSIONS
We presented a hybrid learning system which con-
sists of two different machine learning components,
namely a reinforcement learning component and a be-
lief revision component. This system was applied to
an object recognition problem. We demonstrated in
a first experiment, that the agent is able to learn how
to access such views of an object that allow for a dis-
tinction of the object from a very similar but differ-
ent object. As an indicator for this ability we regard
the promising learning curves and decreasing episode
lengths depicted in Figure 5. In the currentstate of de-
velopment, our system, of course, still exhibits weak-
nesses. For example, the threshold-based goal state
identification is not robust enough to be universally
applicable. In particular, it turned out to depend on
the distance of the camera to the object. Summariz-
ing, we have reason to assume the general applicabil-
ity of our hybrid learning approach to object recogni-
tion tasks.
ACKNOWLEDGEMENTS
This research was funded by the German Research
Association (DFG) under Grant PE 887/3-3.
REFERENCES
Bay, H., Tuytelaars, T., and Van Gool, L. (2006). Surf:
Speeded up robust features. In 9th European Confer-
ence on Computer Vision, Graz Austria.
Deinzer, F., Denzler, J., Derichs, C., and Niemann, H.
(2006). Integrated viewpoint fusion and viewpoint se-
lection for optimal object recognition. In Chanteler,
M., Trucco, E., and Fisher, R., editors, British
Machine Vision Conference 2006, pages 287–296,
Malvern Worcs, UK. BMVA.
Gordon, I. and Lowe, D. G. (2006). What and where: 3d
object recognition with accurate pose. In Ponce, J.,
Hebert, M., Schmid, C., and Zisserman, A., editors,
Toward Category-Level Object Recognition. Springer-
Verlag.
H¨aming, K. and Peters, G. (2010). An alternative approach
to the revision of ordinal conditional functions in the
context of multi-valued logic. In 20th International
Conference on Artificial Neural Networks, Thessa-
loniki, Greece.
Murase, H. and Nayar, S. K. (1995). Visual learning and
recognition of 3-d objects from appearance. Int. J.
Comput. Vision, 14(1):5–24.
Peters, G. (2006). A Vision System for Interactive Object
Learning. In IEEE International Conference on Com-
puter Vision Systems (ICVS 2006), New York, USA.
Schiele, B. and Crowley, J. L. (1998). Transinformation
for active object recognition. In ICCV ’98: Proceed-
ings of the Sixth International Conference on Com-
puter Vision, page 249, Washington, DC, USA. IEEE
Computer Society.
Spohn, W. (2009). A survey of ranking theory. In Degrees
of Belief. Springer.
Sun, R., Merrill, E., and Peterson, T. (2001). From implicit
skills to explicit knowledge: A bottom-up model of
skill learning. In Cognitive Science, volume 25, pages
203–244.
Sutton, R. S. and Barto, A. G. (1998). Reinforcement Learn-
ing: An Introduction. MIT Press, Cambridge.
Viola, P. and Jones, M. (2001). Rapid object detection us-
ing a boosted cascade of simple features. Computer
Vision and Pattern Recognition, IEEE Computer So-
ciety Conference on, 1:511.
ICINCO 2011 - 8th International Conference on Informatics in Control, Automation and Robotics
332