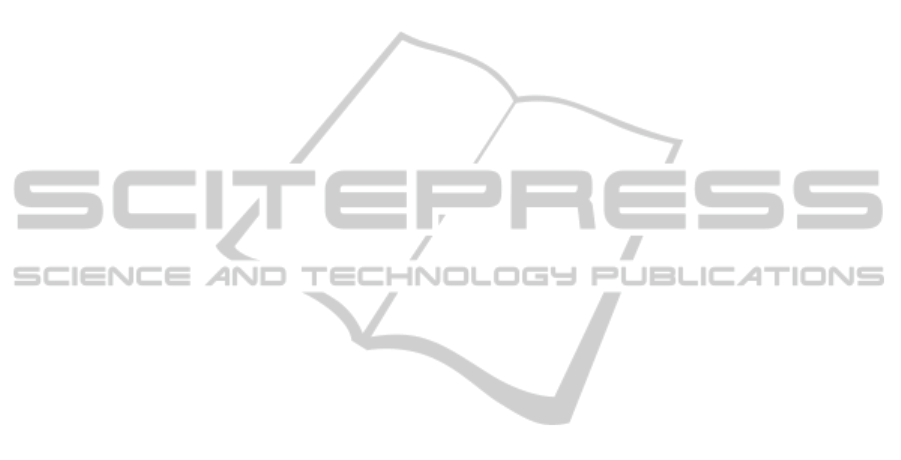
We are in an era where the competition is so strong.
As technology grows exponentially, both the
patients and the doctors would like to use the
technology to cure the patients efficiently and in a
short period of time. Hence, a HCS would like to
attract and enrol more patients and the doctors to its
organization. One of the ways of doing this is to
provide “quality” service at an affordable price and
still make profit. Note that a typical HCS consists of
many (major) groups such as (a) administrative; (b)
doctors who serve the patients insured through the
system; (c) hospitals that serve the patients covered
by the system and (d) laboratories that serve the
hospitals, doctors, and patients. It should be noted
that there may be interactions between these groups.
For example, some hospitals have their own
laboratories which serve the patients admitted to the
hospital either as in-patients or out-patients. Most
doctors visit hospitals to take care of not only their
own patients but also other patients who have been
admitted. We will not model that aspect in this
paper.
The study of such systems in which most of the
underlying variables are random falls in the area of
stochastic modelling and one can avail the tools
therein to study healthcare models. However,
tracking analytically the system performance
measures such as the mean waiting times and the
utilization factors is almost infeasible due to inherent
complexities and the significant interactions that are
present among various segments of a healthcare
system. An alternative approach to analytical
modelling is through simulation. While simulation in
healthcare has evolved at a slow pace (as compared
to other non-healthcare systems such as
manufacturing and telecommunications) over the
last three decades or so (see e.g., Jun, et al., 1999,
Baldwin, et al., 2004), it is recognized as an
important tool in solving problems arising in
healthcare systems. Most of the published papers
dealing with simulation in healthcare systems focus
on sub-systems such as emergency room, outpatient
clinics, etc. (Eldabi, et al., 2010, Gunal and Pidd,
2010). For latest developments in simulation and its
application to many fields including healthcare
systems we refer the reader to journals such as
Simulation Modelling Practice and Theory,
Simulation in Healthcare, Journal of Simulation, and
Proceedings of Winter Simulation Conference.
Thus, the objective of this paper is to provide
insights into how stochastic modelling can be
applied to a typical HCS at a macroscopic level so as
to help the management with aggregate planning. It
is our intent here to focus on the use of simulation to
identify bottlenecks that cause excessive delays in
patients receiving service, and areas of under
utilization of the resources, by looking at a HCS at a
macroscopic level. More specific ones (within this
type of HCS) requiring microscopic level modelling
will be addressed elsewhere.
2 MODEL DESCRIPTION
As indicated earlier a HCS is plagued with wastage,
underutilized resources, and excessive delays
experienced by patients. Whether one is dealing with
handling patients to go through diagnostic labs or to
process paperwork before (and after) the patients go
through diagnostics or the doctors to notify the
patients of the results, or the patients need to be
admitted in the hospitals, or the patients requiring
operations need to wait for beds, equipments,
personnel, delays are inevitable due to available
finite resources and the way they are allocated
among competing service providers. These delays
are further compounded by inherent randomness.
For example, the arrivals of the patients to service
providers are not deterministic. Different classes of
patients arrive and they have to be attended based on
their priorities. These are also random. The service
times are usually random and some patients may
have to go through the same service more than once
for reasons that cannot be anticipated. Thus, a
natural approach to solving such problems is the use
of stochastic modelling. While analytical modelling
is important, there are instances such as the current
study where one has to rely on simulation due to
complex nature of the model.
Patients are the central focus in any HCS and so
we start with assuming that patients arrive to a HCS
according to a Markovian arrival process (MAP)
with representation (D
0
, D
1
) of order m. Note that
the transitions corresponding to no arrivals are
governed by D
0
and the transitions corresponding to
arrivals are governed by D
1
. The underlying
continuous-time Markov chain (CTMC) has the
generator given by Q = D
0
+D
1
. This representation
of MAP is a special case of batch Markovian arrival
process (BMAP). This BMAP was originally
introduced by Neuts (1979) as a versatile Markovian
point process in 1979. MAP, a very versatile point
process used extensively in stochastic modelling,
includes several well-known point processes such as
Poisson, Erlang, and hyperexponential. For full
details on MAP and its applications to stochastic
models we refer to (Lucantoni, 1991, Chakravarthy,
2001, Chakravarthy, 2010). The fundamental rate
(the rate of arrivals per unit of time), λ, is given by
SIMULTECH 2011 - 1st International Conference on Simulation and Modeling Methodologies, Technologies and
Applications
110