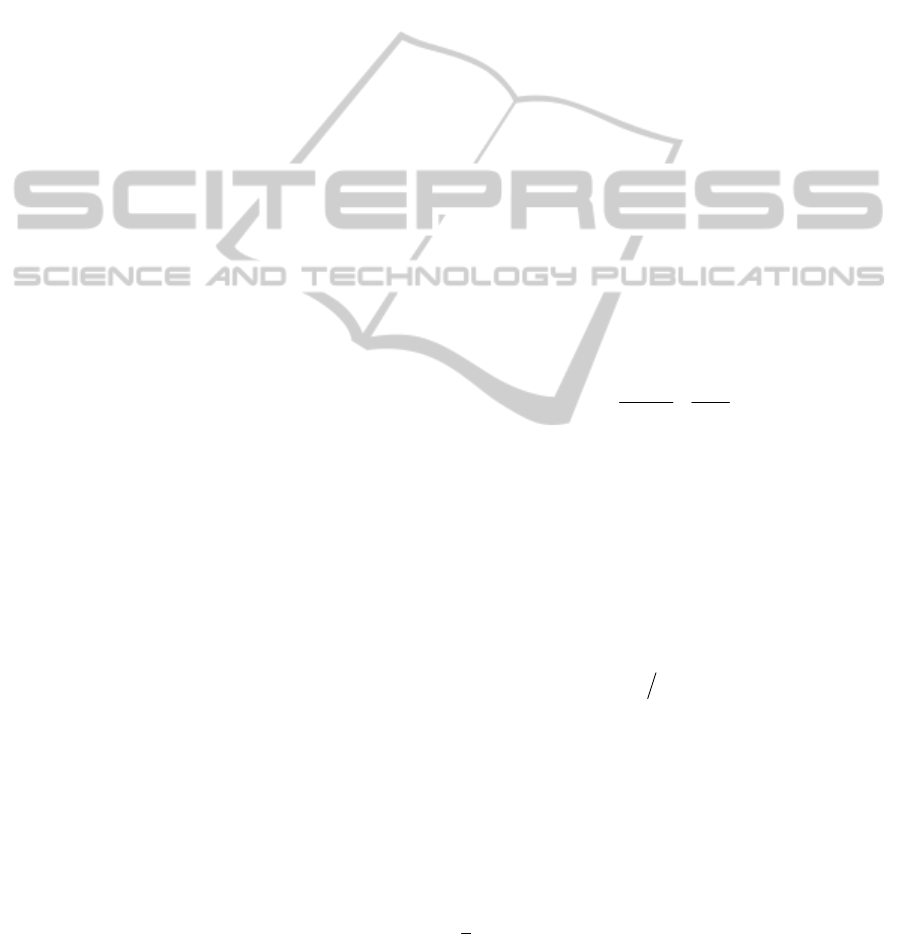
in section 4. Finally we offer some concluding
remarks.
2 THE MODEL
Consider a decentralized supply chain consists of a
risk-neutral supplier and a risk-averse manufacturer
with a fully-liquid electronic market. The supplier
sells an intermediate good via forward contract to
the manufacturer, who use the good as one to one
input to a product selling in the customer market
with revenue
p . The customer market faces a
stochastic demand
d . The supplier decides her
wholesale price w to the manufacturer for the
targeted period. In response to the offer, the
manufacturer must decide the contracted quantity
q .
The contracted quantity is shipped to the
manufacturer at the beginning of the period, and the
supplier will sell her remaining production quantity
(not ordered by the manufacturer) in the spot market
with a stochastic spot price
. Besides contract
procurement, the manufacturer can also purchase
from the spot market during the targeted period. At
the same time, he can sell his excessive inventory in
the spot market. We assume that once the targeted
period starts, the manufacturer cannot reorder from
the supplier for this period. The interactions between
the supplier and manufacturer form a Stackelberg
game, in which the supplier is the leader and the
manufacturer is the follower.
The customer demand
d and the spot price
are typically positively correlated (Litzenberger and
Rabinowitz. 1995, Seifert et al. 2004). That means
that if the customer demand is high, the spot price
usually goes up, and vice versa. For model
tractability, we assume that
d and
follow a
bivariate normal distribution with a correlation
coefficient
0
, i.e.
22
,,,,,.
dsd s
ds BN
Normal distribution assumption is commonly used
in the literature, e.g., Seifert et al. (2004) and Van
Mieghem (2007). To deal with a possible negative
value, we further assume that the standard deviations
of the normal random variables are relatively small
compared to their means. In reality, the spot price
and demand fluctuations in one period usually do
not exceed a certain level.
Let
and
m
be the supplier’s and the
manufacturer’s profits, respectively. Following to
Seifert et al. (2004)’s model, the manufacturer’s
profit function is
m
pd wq s d q s q d
The supplier’s profit includes the profits from the
forward contract and the online spot market. We
have
,
s
wq s Q q
where
Q
represents the supplier’s capacity. More
general model should include the production cost,
which will not influence our analysis.
While seeking profit maximization, the
manufacturer also needs to limit their risk exposures.
In this paper, we explicitly incorporate the player's
risk tolerances in the decision model. Let
k
measures his risk attitudes, and assume
0k
. Let
mm m
UE kVar
be the manufacturer's utility.
This mean-variance utility function has been widely
used to characterize decision makers' risk-averse
behaviors since the seminal work of Markowitz
(1959), and has been widely adopted in recent
operations management studies, e.g., Van Mieghem
(2007).
Lemma 1 (Seifert et al. 2004).
If
22
(,) , , , ,
dsds
ds BN
, then
*
2
2
sd
ds
ss
w
qp
k
Before the targeted period, the manufacturer
observes a customer demand forecast Fd
,
where
1
0,N
. We assume that the forecast
errors
is independent of
d
and s . Since this
paper mainly analyze the effect of forecast accuracy,
we assume that the distributions of underlying
customer demand
d and the spot price
s
are fixed
and only the level of noise in the manufacturer’s
forecast varies.
We denote
222
11d
a
as the demand
forecast accuracy of the manufacturer. The larger the
value of a , the less accurate the manufacturer’s
forecast. In the limiting case where
1a , the
forecast contains no valuable information about
demand and the posterior distribution is identical to
the prior. In the opposite limiting case where
0a
,
the forecast perfectly reveals the exact demand. For
simplicity, we only focus our discussion to
0,1a .
To simply the following analysis, we denote
1aa
, and define the demand conversion factor
and price conversion factor
as
/
d
and /
ds
, respectively. Then,
ICEIS 2011 - 13th International Conference on Enterprise Information Systems
508