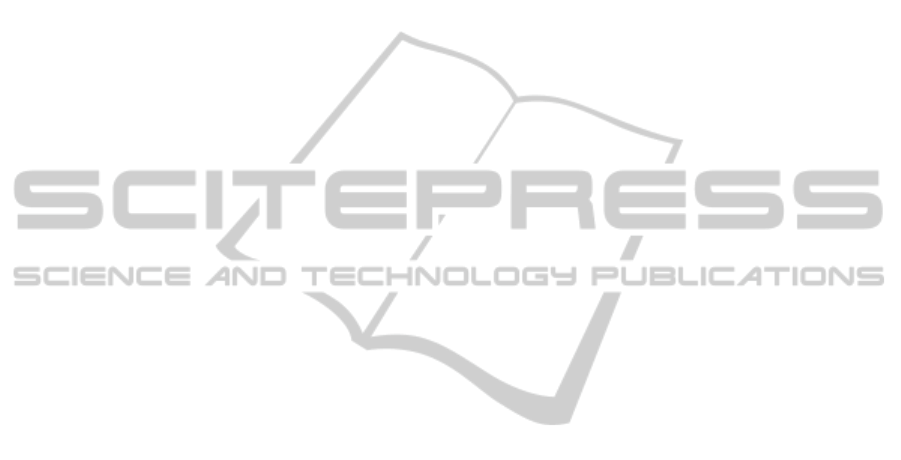
models. These entities, often called decisions
making units (DMU
s
), perform the same function by
trans- forming multiple inputs into multiple outputs.
A main advantage of DEA is that it does not require
any prior assumptions on the underlying functional
relationships between inputs and outputs (Seiford
and Thrall, 1990). It is therefore a nonparametric
approach. In addition, DEA is a data-driven frontier
analysis technique that floats a piecewise linear
surface to rest on top of the empirical observations
(Cooper et al., 2004).
Since the work by Charnes et al. (1978), DEA
has rapidly grown into an exciting and fruitful field,
in which operations research and management
science (OR/MS) researchers, economists, and
experts from various application areas have played
their respective roles (Førsund and Sarafoglou,
2002, 2005). For DEA beginners, Ramanathan
(2003) provided an excellent introductory material.
The more comprehensive DEA expositions can be
found in the recent publication by Cooper et al.
(2006). In the sections that follow, we shall briefly
introduce the basic DEA methodology.
Assume that there are K DMU
s
, e.g. electricity
distribution utilities, to be evaluated that covert N
inputs to M outputs. Further assume that DMU
k
consumes x
nk
>=0 of input n to produce y
mk
>=0 of
output m and each DMU has at least one positive
input and one positive output (Fare et al., 1994b;
Cooper et al., 2004). Based on the efficiency
concept. in engineering, the efficiency of a DMU,
says DMU
o
(o=1,2,...,K), can be estimated by the
ratio of its virtual output(weighted combination of
outputs) to its virtual input(weighted combination of
inputs). To avoid the arbitrariness in assigning the
weights for inputs and outputs, Charnes et al. (1978)
developed an optimization model known as the CCR
in ratio form to determine the optimal weights for
DMU
o
by maximizing its ratio of virtual output to
virtual input while keeping the ratios for all the
DMU
s
not more than one.
2.2 Basic AHP Methodology
Analytic Hierarchy Process(AHP) is theorized by
U.S. Operations Research Professor Saaty TL. It is a
simple, flexible and practical method for multiple
criteria decisions making. It is based on a hierarchy
of multi-objective, subjective judgments based on a
range of options for calculating the relative
importance, followed by a top down basis, through
the decision-makers for each sub-index layer and
index layer provided by the importance of subjective
judgments in pairs, for each unit down to the
pairwise comparison matrix to establish.
Comparison of first through calculating the feature
vector matrix elements get the same level on a level
for the relative importance of the same unit, and then
in accordance with the order from the bottom up
Yici, calculate aggregate importance, end up ranking
value of each option. AHP process was people's
thinking process by fully reflect the preferences of
decision makers, decision makers experience will be
quantified, so as to provide decision makers with
quantitative forms of decisions making. But its
limitations can not be ignored: it relies heavily on
people's experience, subjective factors is large, it can
only rule out the thought process up to the serious
non-compliance, but can not rule out the possible
existence of individual decision-makers A serious
one-sidedness.
2.3 Evaluation of the Significance
of AHP-DEA
The above method of DEA-AHP method described
shows, DEA methods for assessing the results of the
program is totally dependent on the objective
evaluation of indicator data, without considering the
preferences of decision makers, and can only be
divided into units based on the dichotomy of
decision-making both active and inactive Part of
effective decision-making unit of the information
given is too small, can not be a reasonable sort; and
simple AHP, due to the characteristics of semi-
qualitative semi-quantitative determined by its lack
of strict objectivity, subjective factors, too. Taking
into account the practical problems of evaluation
reflects the degree of importance among may vary,
so the decision makers in order to reflect the
preferences of the different level of evaluation, so
that the evaluation of a more comprehensive and
reasonable, considering the above two methods the
author Advantages and disadvantages in use of data
envelopment analysis and analytic hierarchy process
method are combined to establish the subjective and
objective integrated multi-objective comprehensive
evaluation model. The model make up the traditional
method of data envelopment analysis does not
consider the lack of decision-makers preferences,
and overcome the many levels of analysis and
decision making the current weakness of
subjectivity, the evaluation results more
comprehensive and more realistic.
ICEIS 2011 - 13th International Conference on Enterprise Information Systems
528