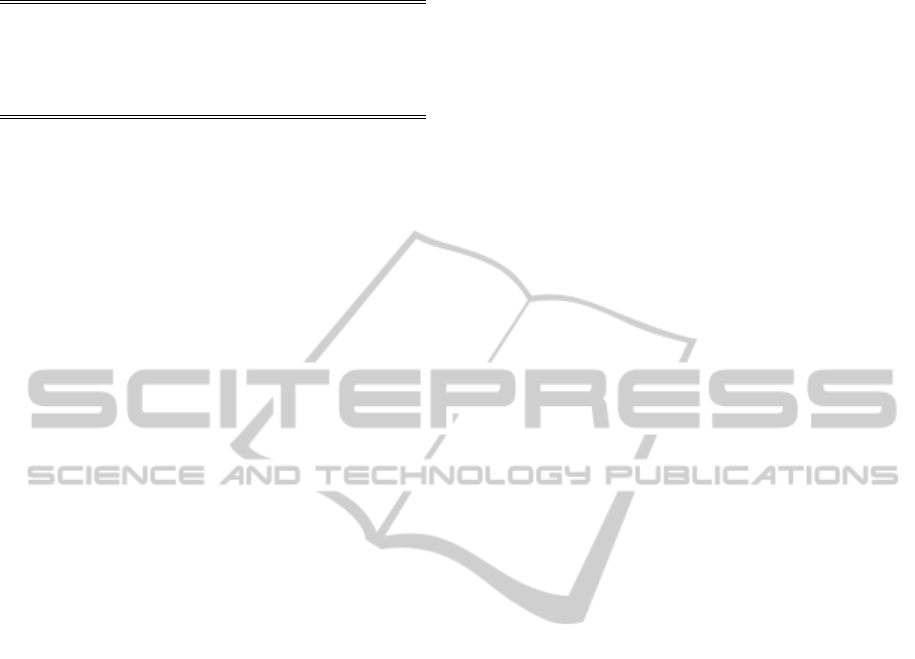
Table 2: Ant Double-B model performance parameter.
6 CONCLUSIONS
This paper is based on CN, through analyzing the
advantage and disadvantage of current network
traffic prediction models. And points out that they
are hard to be applied in CN directly. Then a new
model named ACA Double-BP model is proposed
which has great self-learning and self-adaptation
ability. Comparing with other models, ACA Double-
BP solves the problem of low speed convergence
and local optimum, improves the prevision by means
of rejecting abnormal data. BP does not depend on
training samples using ACA at all. Meanwhile, using
hybrid model obtains high fitting and prediction
prevision. It is applied in CN by using self-
organization and self-learning algorithm. The
Simulation in MATLAB and comparison with WNN
shows that performance of the novel model is better.
But ACA Double-BP is very complex, so how to
improve the efficiency with high-precision character
is the researching trend of this paper.
ACKNOWLEDGEMENTS
This work is partly supported by the Fundamental
Research Funds for the Central Universities of China
under Grant No.2009YJS034, and Beijing Nature
Science Foundation of China (No.4112044).
REFERENCES
Ryan W. Thomas, Luiz A. DaSilva, and Allen B.
Mackenzie, “Cognitive networks”, in Proc.IEEE
DySPAN 2005, Nov. 2005, pp. 352-360.
Zhaoxia Wang, Yugeng Sun, Zengqiang Chen, and Zhuzhi
Yuan, “Study of predicting network traffic using fuzzy
neural networks”, Journal on Communication, vol.26,
pp. 136-140, Mar. 2005.
Qi Jin, Changxing Pei, and Changhua Zhu, ”ARIMA
analysis method in network traffic”, Journal of Xidian
University ( Natural Science), vol.30, pp. 6–10, Feb.
2003.
Guoqiang Yu and Changshui Zhang, “Switching ARIMA
model based forecasting for traffic flow”, in
ICASSP'04. Canana, 2004, Vol.2, pp.429-432.
Aimin Sang and Sanqi Li, “A predictability analysis of
network traffic”, in INFOCOM 2000.Nineteenth
Annual Joint Conference of the IEEE Computer and
Communications Societies, Tel Aviv, Israel, 2000,
Vol.1, pp.342–351.
Chuanshan Gao, Liangxiu Han, Zhiwei Cen and Chunbo
Chu, “A new multi fractal traffic model based on the
wavelet transform”, in Proceedings of the ISCA 14th
International Conference: Parallel and Distributed
Computing Systems, Richardson, Texas USA, Aug.8–
10.2001, pp.157–162.
W. E. Leland, M. S. Taqqu, W. Willinger, and D. V.
Wilson, “On the self-similar nature of Ethernet traffic
(extended version)”, IEEE/ACM Transactions on
Networking, vol.2, pp. 1-15, Jan.1994.
V. Paxson and S. Floyd, “Wide-area traffic: The failure of
Poisson modeling”, IEEE/ACM Transactions on
Networking, vol.3, pp.226-244, Mar. 1995.
D. Husmeier and J. G. Taylor, “Neural networks for
predicting conditional probability densities: improved
training scheme combining EM and RVFL”, Neural
Networks, vol.11, pp. 89,116, Jan. 1998.
D. Hussein, “An object-oriented neural network approach
to short-term traffic forecasting”, European of
Operational Research, vol.131, pp.253-261, Feb.
2001.
Wakurah and J. M. Zurada, “Bi-directional computing
architecture for time series prediction”, Neural
Networks, vol.14, pp. l307-1321, Sep. 2001.
Jie Li, Xiuhong Hou, and Zhijie Han, ”Application of
kalman filter and wavelt in traffic prediction”, Journal
of Electronics & Information Technology, vol. 29, pp.
725-728, Mar. 2007.
Ting Lei and Zhenwei Yu, “A wavelet neural network
model of network traffic forecast”, Computer
Application, vol.26, pp.526-528, Mar. 2006.
Wang Peng and Liu Yuan, “Network traffic prediction
based on improved BP wavelet neural network”, in
Wireless Communications, Networking and Mobile
Computing 2008, Oct. 2008, pp.1-5.
Qigang Zhao, Xuming Fang, and Qunzhan Li, “WNN—
based NGN traffic prediction”, in Proc. ISADS 2005,
Chengdu, China, Apr. 2005, pp. 230-234.
Meng Yao, Yuan Liu, and Gang Zhou, ”Network traffic
prediction model of wavelet combined neural
network”, Computer Engineering and Design
, vlo.28,
pp. 5135-5136 and 5159, Nov. 2007.
Nili Tian and Li Yu, ”A WAN network traffic prediction
model based on wavelet transform and FIR neural
networks”, Journal of Electronics & Information
Technology, vol.30, pp. 2499-2502, Oct. 2008.
R. Schoonderwoerd, O. Holland and J. Bruten, “Ant-based
load balancing in tele-communications networks”,
Adaptive Behavior, vol. 5, pp. 169-207, Feb. 1996.
Bingrong Hong, Feihu Jin, and Qingji Gao, “Multi-layer
feedforward neural network based on ant colony
Name MSE/% SSE/% MRE/% MAE/%
WNN 1.74 10.57 21.14 7.57
ACA
Double-
BP
(time)
24h 1.90 8.26 22.79 7.97
1h 1.09 4.59 14.38 3.52
2h 1.25 6.21 17.56 16.74
ICEIS 2011 - 13th International Conference on Enterprise Information Systems
434