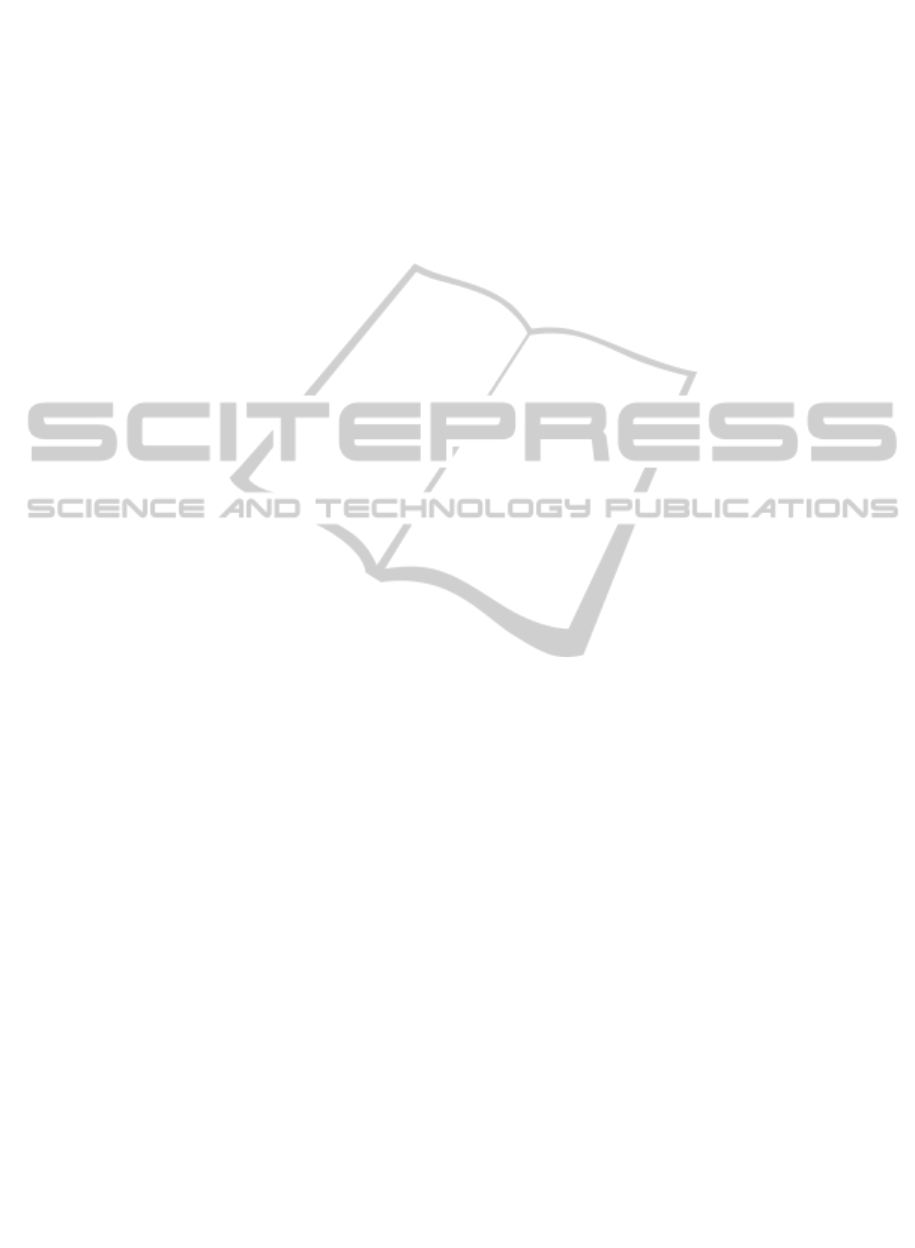
heterogeneous data sources. The data libraries could
have different semantics and syntax, it will be
difficult to extract useful information. Sophisticated
DM tools are needed for this purpose.
In other hands, (Adeva et al., 2007), they
introduces an intrusion detection software component
based on text mining techniques, using text
categorization. This approach is capable to learning
the characteristic of both normal and malicious user
behavior from the log entries generated by the web
application server Text mining refers to the discovery
of non-trivial, previously unknown and potentially
useful knowledge from a collection of text.
Currently, Text Mining (TM) has become an
inevitable part in information retrieval, around 80%
of the information stored in computer consist of text
and digital files. According to work by (Lopes et al.
2007), they framework for visual text mining to
support exploration of both general structure and
relevant topics within a textual document collection,
in this effort, they have answer and examine sets of
documents to achieve understanding of their structure
and to locate relevant information. This is reinforced
by subsequent research by (Zhang et al., 2008), they
argued text classification, namely text categorization,
is defined as assigning predefined categories to text
documents, where documents can be news stories,
technical reports, web pages, and categories are most
often subjects or topics, but may also be based on
style (genres), pertinence, etc.
3 ANALYSIS PROBLEM
While several work have been proposed, there are
several challenges for solving these problems,
including handling dynamic data, sparse data,
incomplete data, uncertain data, and
semistructured/unstructured data. We have
addressing these challenges based on some effort
problems from previously work;
1) The problems are not fully defined in advance.
Grammars will have to be modified to take
account of new data. This is not easy: the
addition of just one new example can
completely alter a grammar and render
worthless all the work that has been expended
in building it, declared by (Witten et al., 1999).
2) There also some effort and problem from
(Singhal, 2007) and (Junqi & Zhengbing, 2008)
to introduce the concepts hybrid approach
effectively with detecting normal usages and
malicious activities using heterogeneous data.
Furthermore, what makes this solution different
from others?
3) How to collecting and integrating information
from different structure, data format, label,
meta data and variable of data. These data set
bulk in information and growing from
community or security services?
4) How we can convert and integrating this data
into information, and subsequently into
knowledge.
5) How to extract the relationships, and then
correlate data source to run on the new
environment if the data sources could be based
on complex structure and many relationships?
6) Is it true to integrate data for the process of the
standardization data definitions and data
structures by using a common conceptual
schema across a collection of data sources?
With respect work by (Singhal, 2007) present four
data source with multiple audit streams from diverse
cyber sensor: (i) raw network traffic, (ii) netflow
data, (iii) system call, and (iv) output alert from IDS.
Unfortunately, we assume this method can not
effective with new challenge of intrusion threat.
However, with respect we improve and expand this
opinion to our approach, in this approach we use
sixteen event parameters from heterogeneous data
input. We present sixteen interrelated of information
in database for knowledge process. Accordingly,
obtaining general pattern with variation diversity
structure, label, and variable of data to potentially
useful knowledge is another part of this research.
In this study, DM is used to perform data
collection using history, patterns, and relationships to
classification and estimation of attack in stream
network. This is due to hybrid system receive data
from many different sources and it is expected that a
hybrid system has the potential to detect
sophisticated attacks that involve multiple networks
with the information from multiple sources. As a
mentioned above, Learning technique from DM can
be solution for research objective (i) prediction of
attack pattern, (ii) identification from anomaly
habitual activity, (iii) estimation normal activity
based on habitual activity, (iv) classification
attack/suspicious packet, (v) mapping habitual-
activity, and (vi) early prevention security violation.
We use DW to collecting scattered information in
routine update regularly from provider or security
community, we illustration in Figure 1. From our
observation, these data can be useful information to
be associated with other. The information,
increasingly large of volume dataset and
multidimensional data has grown rapidly in recent
RESEARCH ON HETEREGENEOUS DATA FOR RECOGNIZING THREAT
223