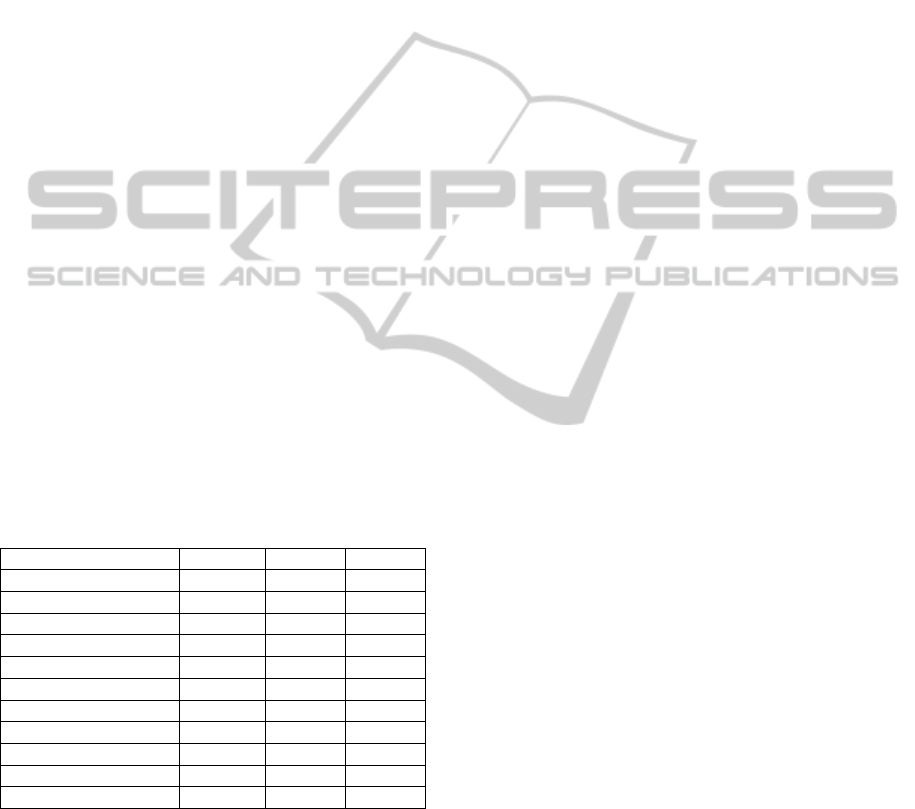
language model considering punctuation symbols as
words. Similar method is used for speaker change
marking too. Other keys are reserved for special
announcements and situations.
The re-speaker is trained on the real video files
containing OOV words (mainly named entities) that
should be added to the LVCSR system just during
the captioning. This is a crucial procedure that
should not take much time. We have implemented a
method for word additions in real-time during the
recognition of LVCSR system. The simplest way is
to type a word (or multi-word) and confirm it. The
system searches for the word in the special large lists
of named entities and if succeeds, it adds the word
with correct phonetic transcription(s) to the LVCSR
system. In the case of unknown word, automatic
phonetic transcription is proposed and optionally
modified by the re-speaker. This approach
minimizes the time for word addition in most cases.
3 EVALUATION
To assess the training possibilities of the described
system, we have evaluated three re-speakers who got
through all the training phases. The re-speakers
produced the captions for the same real TV debate
(61 minutes) and the final captions were evaluated.
The recognition accuracy (including the pending
words corrections) and some statistics collected
during the captioning are presented in Table 1.
Table 1: Evaluation of re-speakers on the TV debate.
NZ EK PZ
Training time
138 hours 98 hours 78 hours
Recognition accuracy
97,37 % 97,34 % 94,32 %
Suitability measure
85,66 % 84,41 % 82,38 %
Words
4294 2960 4217
Word additions
8 12 12
Word corrections
83 66 73
Word dispatches
60 136 100
Commas
319 170 287
Full stops
341 248 335
Question marks
49 48 57
New speakers
109 103 120
4 CONCLUSIONS
The proposed system facilitates the re-speaker
training by decomposing the education process into
four gradual phases, making the training easier and
thus faster. The overall training time is highly
individual, but according to our expertise, we expect
the time of intensive training plan to be set from 2 to
3 months (100 training hours at minimum).
As the development of our training system
continues, we want to allow synchronous training of
caption correctors that are indispensable for error-
free captioning of some critical TV broadcastings.
ACKNOWLEDGEMENTS
This work was supported by the Ministry of
Education of the Czech Republic under the projects
MŠMT 2C06020 and MŠMT LC536 and by the
grant of The University of West Bohemia, project
No. SGS-2010-054.
REFERENCES
Boulianne, G., Beaumont, J.-F., Boisvert, M., Brousseau,
J., Cardinal, P., Chapdelaine, C., Comeau, M., Ouellet,
P., Osterrath, F., 2006. In International Conference on
Spoken Language Processing.
Evans, M. J., 2003. Speech Recognition in Assisted and
Live Subtitling for Television. WHP 065. BBC R&D
White Papers.
Homma, S., Kobayashi, A., Oku, T., Sato, S., Imai, T.,
Takagi, T., 2008. New Real-Time Closed-Captioning
System for Japanese Broadcast News Programs. In
Computers Helping People with Special Needs.
Springer.
Neto, J., Meinedo, H., Viveiros, M., Cassaca, R., Martins,
C., Caseiro, D., 2008. Broadcast news subtitling
system in Portuguese. In IEEE International
Conference on Acoustics, Speech and Signal
Processing.
Pražák, A., Müller, L., Psutka, J. V., Psutka, J., 2007.
LIVE TV SUBTITLING - Fast 2-pass LVCSR System
for Online Subtitling. In International Conference on
Signal Processing and Multimedia Applications.
Pražák, A., Zajíc, Z., Machlica, L., Psutka, J. V., 2009.
Fast Speaker Adaptation in Automatic Online
Subtitling. In International Conference on Signal
Processing and Multimedia Applications.
Verhelst, W., 2000. Overlap-add methods for time-scaling
of speech. In Speech Communication. Elsevier.
Wald, M. and Bell, J.-M. and Boulain, P. and Doody, K.
and Gerrard, J., 2007. Correcting automatic speech
recognition captioning errors in real time. In
International Journal of Speech Technology.
SIGMAP 2011 - International Conference on Signal Processing and Multimedia Applications
220