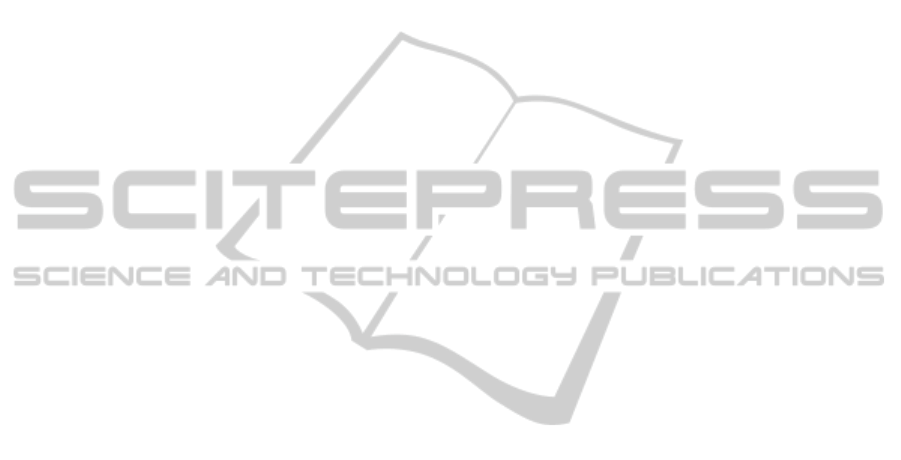
model allows taking into account interactions
between the processes and making assessment of
REDD policies applying similar method in all
countries. The model is designed to use data
available at different scales (from local, grid cell
specific to global). One of the important
requirements to the data composition is consistency
of all constituents. The approach proves its validity
by providing plausible results compared against
independent estimates and tested by national experts
in EU countries. The model results are widely used
for integrated assessment purposes or in other
applications.
Further research: To improve performance of the
model in tropics we plan to introduce initialisation
of deforestation in cells using remote sensing data
and add a road network that is shown to be an
important deforestation driver (Kirby et al, 2006).
ACKNOWLEDGEMENTS
We are grateful to Michael Obersteiner and Hannes
Bottcher for useful ideas on model development and
comments on the model performance, and Andriy
Bun for helping with model code.
The model development was supported by the
following EC funded projects: CC-TAME,
ClimateCost, BEE Project, GEO-BENE, INSEA.
REFERENCES
Benitez P. C. and Obersteiner M., 2006. Site identification
for carbon sequestration in Latin America: A grid-
based economic approach. Forest Policy and
Ecconomics, 8:636–651.
Böttcher H., Verkerk H., Gusti M. et al. 2011. Projection
of the EU27 forest carbon sink and effects of recent
bioenergy policies using two advanced forestry
models. Under preparation
Cramer W, Kicklighter D. W, Bondeau A. et al, 1999.
Comparing global models of terrestrial net primary
productivity (NPP): Overview and key results. Global
Change Biology, 5(S1): 1–15.
FAO, 2010. Global Forest Resources Assessment 2010.
Main Report. Food and Agriculture Organization of
the United Nations, Rome, 2010, 375p.
GGI Scenario Database, 2007. International Institute for
Applied System Analysis (IIASA) GGI Scenario
Database, http://www.iiasa.ac.at/Research/GGI/DB/
Grubler A., Nakicenovic N., Riahi K. et al, 2007.
Integrated assessment of uncertainties in greenhouse
gas emissions and their mitigation: Introduction and
overview. Technological Forecasting and Social
Change, 74 (7): 873-886
Gusti M., Havlik P., Obersteiner M., 2008. Technical
Description of the IIASA Model Cluster. The Eliasch
Review. Office of Climate Change, UK.
Gusti M., Havlik P., Obersteiner M., 2009. How much
additional carbon can be stored in forests if economic
measures are used and how much could it cost?
Research Reports of the National University of
Bioresources and Nature Management of Ukraine,
Issue 135.
Gusti M., 2010a. An Algorithm for Simulation of Forest
Management Decisions in the Global Forest Model.
Artificial Intelligence, 4: 45-49
Gusti M., 2010b. An Algorithm for Simulation of Forest
Management Response to Carbon Price Incentive.
Proceedings of the conference ‘Modern Information
Technologies in Economy, Management and
Education”, 12 November 2010 Lviv, pp. 94-97
Gusti M., 2010c. Uncertainty of BAU emissions in
LULUCF sector: Sensitivity analysis of the Global
Forest Model. Proceedings of the 3rd International
Workshop on Uncertainty in Greenhouse Gas
Inventories, LPNU, Lviv, 299p. - pp.73-80.
JRC 2003. The Global Land Cover Map for the Year
2000. GLC2000 database. European Commission
Joint Research Centre; http://www-gvm.jrc.it/glc2000
Kindermann G., Obersteiner M., Rametsteiner E. and
McCallcum I., 2006. Predicting the Deforestation–
Trend under Different Carbon–Prices. Carbon Balance
and Management, 1:15
Kindermann G., McCallum I., Fritz S., Obersteiner M.,
2008a. A global forest growing stock, biomass and
carbon map based on FAO statistics. Silva Fennica,
42(3): 387-396
Kindermann G., Obersteiner M., Sohngen B., et al, 2008b.
Global cost estimates of reducing carbon emissions
through avoided deforestation. PNAS, 105 (30):
10302–10307.
Kirby K., W. Laurance, A. Albernaz, et al. 2006. The
future of deforestation in the Brazilian Amazon.
Futures, 38: 432-453
Ramankutty N., Foley J. A., Norman J., McSweeney K.,
2002. The global distribution of cultivable lands:
current patterns and sensitivity to possible climate
change. Global Ecology & Biogeography, 11(5): 377-
392.
Ramankutty, N., and J. A. Foley, 1999. Estimating
historical changes in global land cover: croplands from
1700 to 1992. Global Biogeochemical Cycles, 13(4):
997-1027.
Tubiello F. N., Fischer G., 2007. Reducing climate change
impacts on agriculture: Global and regional effects of
mitigation, 2000-2080. Technological Forecasting and
Social Change, 74 (7): 1030-1056
WDPA Consortium, 2004. World Database on Protected
Areas. Copyright World Conservation Union (IUCN)
and UNEP-World Conservation Monitoring Centre.
World Bank, 2005. World Development Indicators. World
Bank.
AN APPROACH TO MODELING LANDUSE CHANGE AND FOREST MANAGEMENT ON A GLOBAL SCALE
185