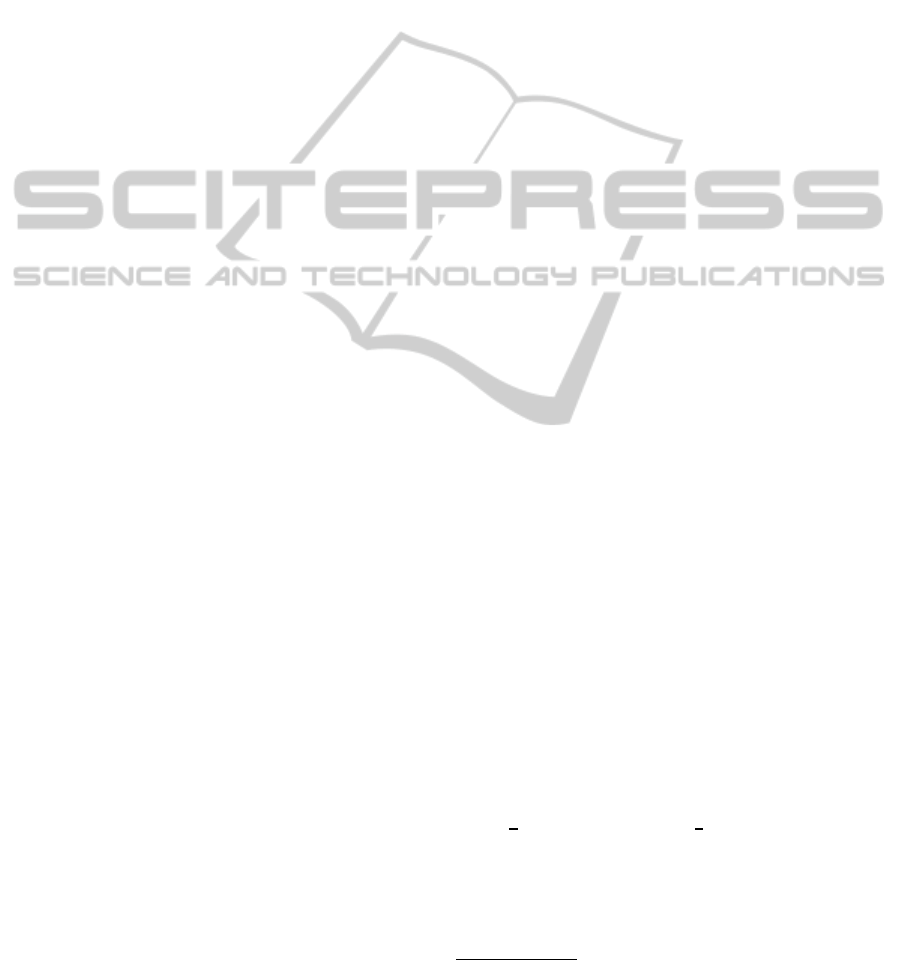
sic benchmarks of FDs to verify both the efficiency
and behavior of the algorithms.
Another important concern is the design of infer-
ence systems for the management of FDs that may
serve as a basis for the development of automated
management of FDs. Armstrong’s axiomatic system
is the core for many logics of FDs. All these logics
have a common characteristic: they use the transitiv-
ity rule which limits their direct application and the
further development of methods with efficient deduc-
tion capabilities. As a conclusion, these logics are not
used in the definition of FDs management algorithms.
There exists another logic approach that substi-
tutes the transitivity rule by a new rule and allows
the creation of automatic algorithms for the manage-
ment of FDs. The authors (
´
Angel Mora et al., 2004)
presented the Simplification Logic for FDs (SL
FD
),
which is equivalent to Armstrong’s axiomatic system.
The main core of SL
FD
is a new rule of simplifica-
tion, that allow the elimination of redundant attributes
efficiently, turning it into an executable logic which
opens the door to the creation of efficient algorithms.
The authors have also developed a set of algo-
rithms to solve the most important FDs problems: the
closure of a set of atoms, the redundancy removal to
get FDs basis and the calculation of minimal keys.
3 A DISCUSSION AROUND
IMPLEMENTATION ISSUES
All the algorithms for FDs deal with a simple data
structure: FDs are represented using two associated
sets of attributes. Their flow are mainly based on
primitives set operators: union, intersection, differ-
ence, etc.
As (Wirth, 1978) points out, programs arenotonly
algorithms, they depend strongly in data structures.
We have made two version of the implementation of
set of attributes. The two implementations are based
on the representation of the set of attributes as a set of
bits. So we have two versions of each DF algorithm:
Fixed. The size of the set of atoms of is fixed and
each bit represents an attribute. The cost of set
operators depends on the maximum number of at-
tributes of all the FDs in the set.
Sparse. The size of the set is variable, having the
same cardinality than the number of attributes on
each side of the FD. The cost of set operators de-
pend on the size of the FD involved in the concrete
execution.
Sparse implementations are recommended when
the number of attributes which form FD, is very low
compared to the cardinality of the set of atoms. This
situation may be found on those models with a sig-
nificant number of “small” FDs. In models where all
the attributes appears in only a few number of FDs is
better tackled using the fixed approach. A degener-
ated version of this situation is the start models, used
in data warehousing, with a central table containing
the data analysis, surrounded by other smaller tables
called dimension tables.
The standard algorithm (Maier, 1983) is first pre-
sented in the literature that calculates the closure in a
nonlinear time: O(kUkkΓk
2
)
1
, where Γ is the set of
FDs and U the set of all attributes in Γ.
In this work we have consider five different clo-
sure algorithms which appears in the literature: (Beeri
and Bernstein, 1979), (Diederich and Milton, 1988),
(Paredaens et al., 1989) , (Maier, 1983) and (Mora
et al., 2006). Each algorithm, as we have mention
above, has been development in two different ver-
sions: fixed and sparse. To compare the efficiency
of these algorithms, we have also developed a method
to generate random sets of FDs with different char-
acteristics, provided by a set of different parameters
detalied above.
3.1 Benchmarking of Functional
Dependencies
As we have mentioned, the lack of benchmarks for
FDs limits the unified comparison of proofs for the ef-
ficiency and behavior of the FDs algorithms. Unless it
is possible to generate random FDs sets, a more depu-
rated method to produce set of FDs which represent
different models is demanded. He have developed
strategies to increase the control in the generation of
FDs sets. In our approach the user can parametrize
the random generation which determines the selection
of an strategy. This strategies are characterized by a
combination of the cardinality of the set of attributes
and the size
2
of the right and left hand sides in the
FDs:
Size. This is the first strategy used in (Mora et al.,
2006). In this strategy, we provide a maximum
level to the size of FDs and the left size is limited
to
1
4
of this threshold and
1
3
on the right.
Vanilla. The user provides two values which repre-
sent the maximum percentage of attributes on the
left and on the right. The percentages determines
two separate lengths in both sides of the FDs and
1
kXk
denotes the cardinality of
X
2
The size is defined in the literature as the sum of the
lengths of the left-hand side and the right-hand side.
ICSOFT 2011 - 6th International Conference on Software and Data Technologies
314