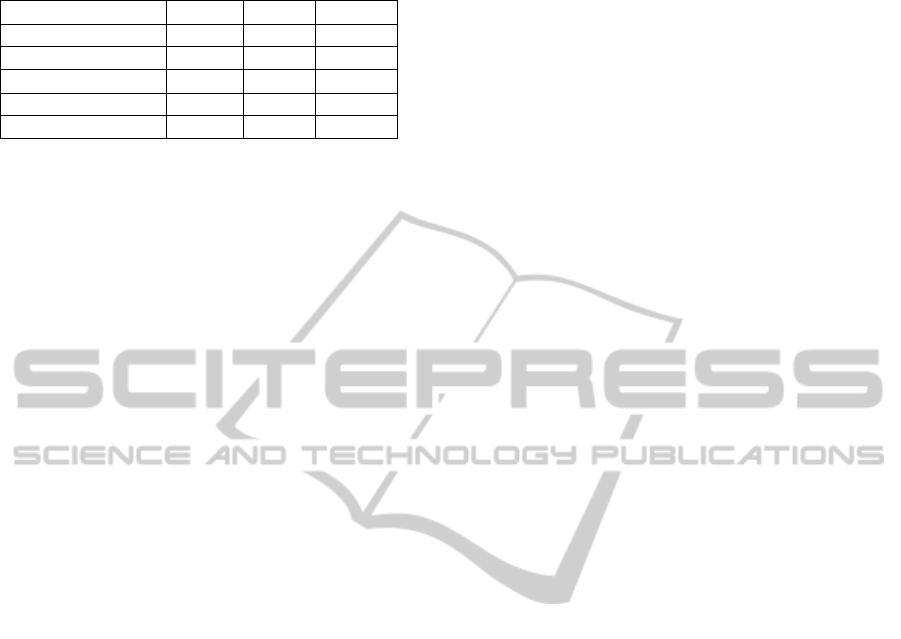
Table 1: Results of all fuzzy approaches to the global
temperature change problem.
Method MSE #Rules Time
ANFIS 2.38% 27 15sec.
TSK-IRL-R 3.03% 50 50min.
MOGUL-TSK-R 3.09% 121 >60min.
MOGUL-IRLHC-R 10.08% 34 28min.
FIR 0.25% 56 5sec.
Therefore, it can be concluded that the different
fuzzy approaches used to model the global
temperature change problem are useful for the task
at hand, because all of them have a high level of
prediction accuracy. Depending on the users
interests it can be more desirable to choose a
methodology with high precision in the prediction,
like FIR, or a less precise model but with a small
number of rules in it, like ANFIS, MOGUL-IRLHC-
R or TSK-IRL-R.
This work is an initial attempt to compare
different types of fuzzy modeling approaches when
dealing with ecological systems. It does not pretend,
at this point, to be an exhaustive and rigorous
comparison, but to give a first inside into hybrid
fuzzy modeling of ecological problems. The next
step is to incorporate other fuzzy-based
methodologies, such is the LR-FIR, which is an
attempt to reduce the number of FIR rules obtained
while minimizing the loss of precision in the
prediction. Finally, we plan to study other ecological
problems mainly focused in climate systems.
5 CONCLUSIONS
This paper studies the usefulness of hybrid fuzzy
modelling approaches when dealing with a real
ecological system, i.e. the global temperature
change. A box model of the ocean-atmosphere, that
reproduces satisfactorily the wide range of
temperature increase reported by the IPCC, is used.
From the temperature increase calculated with
the box model, different hybrid fuzzy models are
built. Concretely, the ANFIS that is a neuro-fuzzy
system, the TSK-IRL-R, MOGUL-TSK-R and
MOGUL-IRLHC-R that are genetic-fuzzy systems
based on the iterative rule learning approach, and the
FIR methodology. All the models are able to predict
accurately the global temperature increase in the
year 2100. The fuzzy models presented in this paper
are simpler than the box model and are much more
understandable from a policy maker point of view.
REFERENCES
Alcalá, R, Alcalá-Fdez. J., Casillas, J., Cordón, O.,
Herrera, F., 2007. Local identification of prototypes
for genetic learning of accurate TSK fuzzy rule-based
systems. International Journal of Intelligent Systems,
22, 909-941.
Bloch, I., 2005. Fuzzy spatial relationships for image
processing and interpretation: a review. Image and
Vision Computing, 23(2), 89-110.
Cordon, O., Herrera, F., 1999. A Two-Stage Evolutionary
Process for Designing TSK Fuzzy Rule-Based
Systems. IEEE Transactions On Systems, Man, And
Cybernetics—Part B: Cybernetics, 29 (6).
Cordon, O., Herrera, F., 2001. Hybridizing genetic
algorithms with sharing scheme and evolution
strategies for designing approximate fuzzy rule-based
systems. Fuzzy sets and systems, 118, 235-255.
Cordon, O., Herrera, F., Hoffmann, F., Magdalena, L.,
2001. Genetic Fuzzy Systems. Evolutionary Tuning
and Learning of Fuzzy Knowledge Bases. Vol. 19 of
Advances in Fuzzy Systems - Applications and
Theory. World Scientific.
Escobet, A., Nebot, A., Cellier, F. E., 2008. Visual-FIR: A
tool for model identification and prediction of
dynamical complex systems. Simulation Practice and
Theory, 16, 76-92.
IPCC, 2007. Climate Change. Cambridge University
Press.
Jang, J.R., 1993. ANFIS: Adaptive-Network-Based Fuzzy
Inference System. IEEE Transactions on systems, man
and cybernetics, 23 (3).
Keel Platform,2004. http://sci2s.ugr.es/keel/developpment.
php.
Klir, G. J., Elias, D., 2002. Architecture of Systems
Problem Solving,Plenum Press. New York, 2
nd
edition.
McGuffie, K., Henderson-Sellers, A., 2005. A Climate
Modelling Primer. Third Edition. Wiley.
Nebot, A., Mugica, F., Cellier, F. E., Vallverdu, M., 2003.
Modeling and Simulation of the Central Nervous
System Control with Genetic Fuzzy Models.
Simulation: Society for Modeling and Simulation
International, 79(11), 648-669.
Watanabe, K., Izumi, K., Maki, J., Fujimoto, K., 2005. A
Fuzzy Behavior-Based Control for Mobile Robots
Using Adaptive Fusion Units. Journal of Intelligent
and Robotic Systems, 42(1), 27-49.
Wigley, T. M. L., Schlesinger, M. E., 1985. Analytical
solution for the effect of increasing CO2 on global
mean temperature. Nature, 315, 649-652.
Williams, P. D., 2005. Modelling climate change: The role
of unresolved processes. Phil. Trans. R. Soc. A, 363,
2931-2946.
Zadeh, L. A., 1965. Fuzzy Sets. Information and Control.
8(3), 338-353.
FUZZY APPROACHES FOR MODELING DYNAMICAL ECOLOGICAL SYSTEMS
379