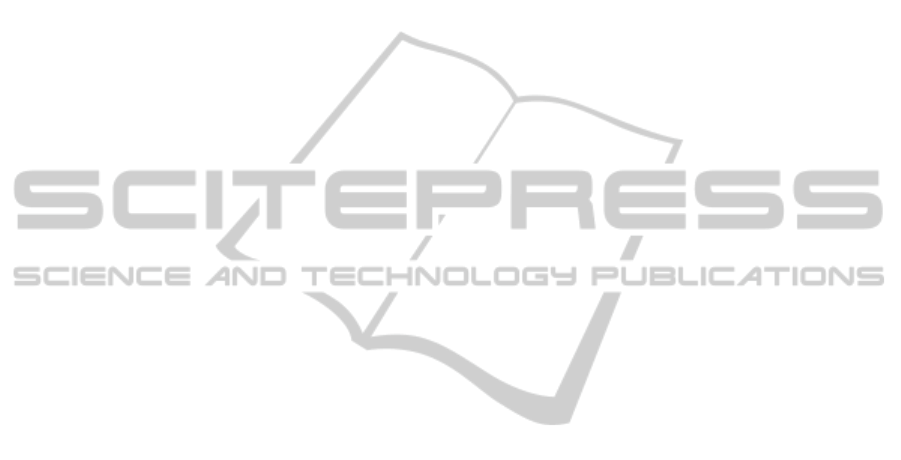
developed regions, venture investment is not the best
innovation investment method. The relation, namely
the venture investment has much larger or times of
stimulating influence on patent innovation than the
effects of R&D, stands no ground.
First, according to the model, if two industries
differ in characteristics of technology and economy,
the innovation investment forms that they fit for will
differ accordingly. For example, as to the rising
industries with vast prospects, who have
sophisticated technology and great uncertainty, and
who face fierce competitive market without mature
standard, such as IT, artificial intelligence, genetic
pharmaceuticals, venture capital is an optimum
innovation investment. However, as to the industries
with relatively mature technology and serious
competition such as textile and light industry, due to
mature technology standards , specified market, and
high level of marketization, the suitable innovation
investment mode are corporate R&D input or private
investment. The reason is that in this kind of
industry, the growth margin is limited. If the venture
investment enters this kind of industry, the rate of
return will be very low. At the same time, since the
cost of transaction and management is very high, the
community income of society doesn’t accord with
personal income, and the rate of return of national
R&D investment will be low too. As a result, for this
kind of industry, these two kinds of innovation
investment modes may not be suitable.
Then, for the developing countries and less-
developed regions, what are the essential differences
in terms of characteristics of industrial technology
and economy between them and the developed
countries? The writer maintains that the most
distinctive difference between them lies in that the
developed countries are in the leading edge of
industrial technology and economy, while the
developing countries and less-developed regions are
mostly in the following edge. Just as Mr. Lin Yifu
point out, because the developed counties occupy
the leading positions in global industrial chain, in
most of the cases enterprises have different views on
the problem that which industry will come as next
new and promising industry in the national
economy, so they form no social consensus. Among
various investment options, projects of few
enterprises succeed, while projects from most
enterprises would fail. The continual economic
development relies on the choice of market. Later
reality proves that the investment projects of a
number of successful enterprises will promote next
round of emerging of new industry, and drive the
development of entire national economy. However,
the industries of developing countries position low
in the global chain of industry, the economic
development of developing countries positions
inside the global industrial chain, go through a
process of upgrading along the track of the current
industry with varied capital and technology
intensity. The industrial upgrading during economic
developing, the enterprises invest in the technology-
mature, product-existing-market industries inside the
global industrial chain. Which industry is new and
which is promising? The enterprises inside the
economy are opting to see eye to eye with one
another, and swarm into it one by one and form
“emergence”. (Lin Yifu, 2007) This difference
between the developing countries and the less-
developed regions decides their essential differences
in technology innovation: the technology innovation
activities in the developed countries position mainly
in the industries in Section Ⅲ , Ⅳ , and Ⅴ of the
model; while the technology innovation activities in
the developing countries and less-developed regions
position mainly in the industries in the Section Ⅰ,
Ⅱ, and Ⅲ of the model. That is to say, in the
developing countries and less-developed regions, in
terms of industrial structure, the industries with
relative mature technology and high level of market
competition dominate. This judgment could be
proved by the proportion and changes of added
value of Chinese high-tech industry in GDP since
1996. The statistics in Table 1 indicate that the
proportion of added value of high-tech industry of
China in GDP will rise from 1.81% in1996 to 4.48%
of 2007, presenting an entire rising trend. Although
it indicates a great advancement of high-tech
industry of China over more than ten years, it, at the
same time, also presents an important fact that the
scale of the high-tech industry of China is still very
small, and other traditional industries apart from
high-tech industry still dominate the industrial
structure of China.
Different sections maintain different
characteristics of industrial technology and
economy, so the suitable innovation investment
mode should be different too. In terms of the
characteristics of technology and economy, high-
tech industry positions in the section Ⅳ of the
model, and the suitable innovation investment mode
in the rising phase is national public R&D input, and
in the following phase is venture investment.
However, the industrial system of the developing
countries and less-developed regions is still
dominated by the industries in Section Ⅰ, Ⅱ, and
Ⅲ, so the innovation investment mode should be
ICEIS 2011 - 13th International Conference on Enterprise Information Systems
620