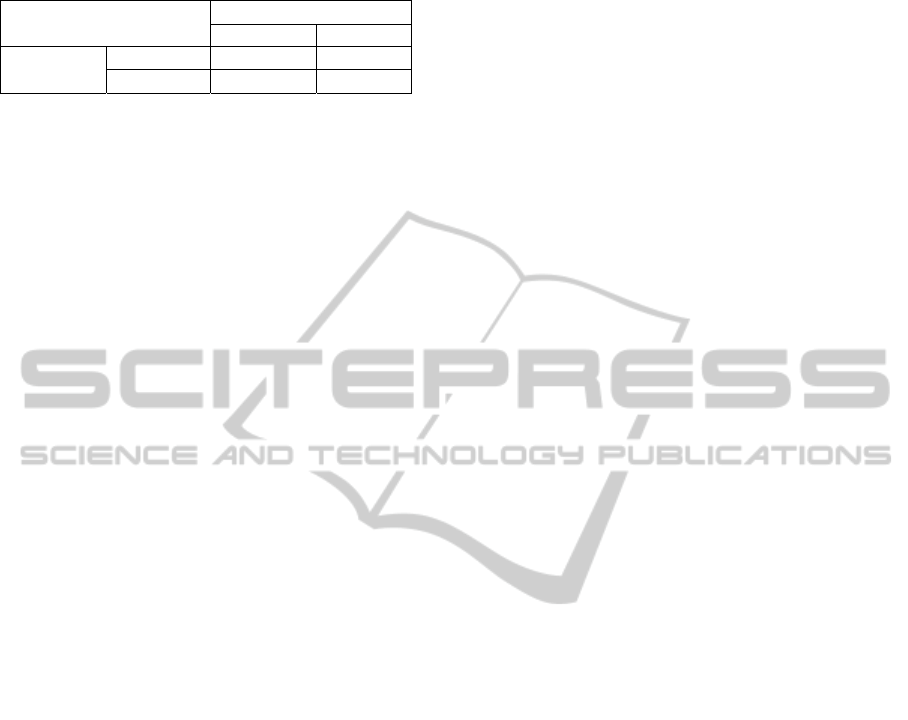
Table 3: Confusion matrix: average values of the
validation results of 5 different M6 MARS models trained.
Real category
Non-bankr. Bankr.
Predicted
category
Non-bankr. 11,513 6
Bankr. 1,339 46
In addition, according to the information
contained in Table 3 it must be remarked that the
specificity of the model is 89.58%, that is, it is able
to detect 89.58% of the companies that did not go
bankrupt. It also detects 88.46% of all those
companies that went bankrupt (sensitivity). Finally,
we must also underline that the global accuracy of
the model is 89.58%.
4.2 Benchmark Techniques
As indicated above, the benchmark techniques used
to compare with the results obtained by the
algorithm proposed in the present paper were two:
back propagation NN and MARS. The model has 5
neurons in the input layer and 7 in the intermediate.
The MARS model obtained was of degree 2
although no maximum degree condition was
imposed.
For the estimation of the accuracy of NN and
MARS, we followed a procedure similar to that
proposed to test the accuracy of the proposed
algorithm. NN and the MARS model were applied to
five random selected training data bases (80% of the
data chosen at random) and tested over their
corresponding validation subsets (the remaining
20% of the database).
For the case of the NN model, the results
obtained in the five runs yielded an average
specificity of 99.95%, an average sensitivity of
21.00% and an average global accuracy of
99.01%.Although the specificity the NN-based
device is higher than that of our proposal, it is
inefficient for the detection of bankrupt companies,
due to its low sensitivity. This makes this model
useless for decision-aid purposes because the costs
of the error consisting in considering a bankrupt
company as non-bankrupt are very much higher than
that of the opposite error.
The results obtained for the simple MARS model
were as follows: average specificity of 99.79%,
average sensitivity of 3.85% and average global
accuracy of 99.78%. These results are even worse
than those of NN, so it can be concluded that the
simple MARS model is also useless for practical
purposes.
5 SUMMARY, CONCLUDING
REMARKS AND FURTHER
RESEARCH
This paper proposes a new approach to the
forecasting of firms’ bankruptcy. Our proposal is a
hybrid method in which sound companies are
divided in clusters according to their financial
similarities and then each cluster is replaced by a
director vector which summarizes all of them. In
order to do this, we use SOM mapping. Once the
companies in clusters have been replaced by director
vectors, we estimate a classification model through
MARS.
We used two benchmark techniques to compare
with the results obtained by the algorithm proposed
in the present paper: a back propagation neural
network and a MARS model.
Our results show that the proposed hybrid
approach is much more accurate than the benchmark
techniques for the identification of the companies
that go bankrupt. As future research efforts we can
mention the application of the procedure proposed in
the present research to other related tasks in the field
of financial statements analysis (i.e. prediction of
takeovers, analysis of bond ratings, etc.).
REFERENCES
Altman, E. I., 1968. Financial ratios, discriminant analysis
and the prediction of the corporate bankruptcy, Journal
of Finance, 23, 589-609.
Altman, E. I., 1993. Corporate Financial Distress and
Bankruptcy, New York: John Wiley and Sons.
Bank for International Settlements (BIS), 2006.
International Convergence of Capital Measurement
and Capital Standards. A Revised Framework, Basel:
BIS.
Foglia A., Iannotti S., Marullo-Reedtz, P., 2001. The
Definition of the Grading Scales in Banks’ Internal
Rating Systems. Economic Notes, 30, 421-456.
Friedman, J. H., 1991. Multivariate adaptive regression
splines. Annals of Statistics, vol. 19: 1-141.
Kohonen T., 1995. Self-Organizing Maps, Berlin:
Springer-Verlag, 1
st
edition.
Mahalanobis, P. C., 1936). On the generalised distance in
statistics. Proceedings of the National Institute of
Science of India, 12: 49–55.
Perner P., 2008. Advances in Data Mining - Medical
Applications, E-commerce, Marketing, and Theoretical
Aspects. Berlin: Springer-Verlag, 1
st
edition.
Rousseeuw P. J., Van Zomeren B. C., 1990. Unmasking
multivariate outliers and leverage points. Journal of
the American Statistical Association, 85, 633-651.
KMIS 2011 - International Conference on Knowledge Management and Information Sharing
286