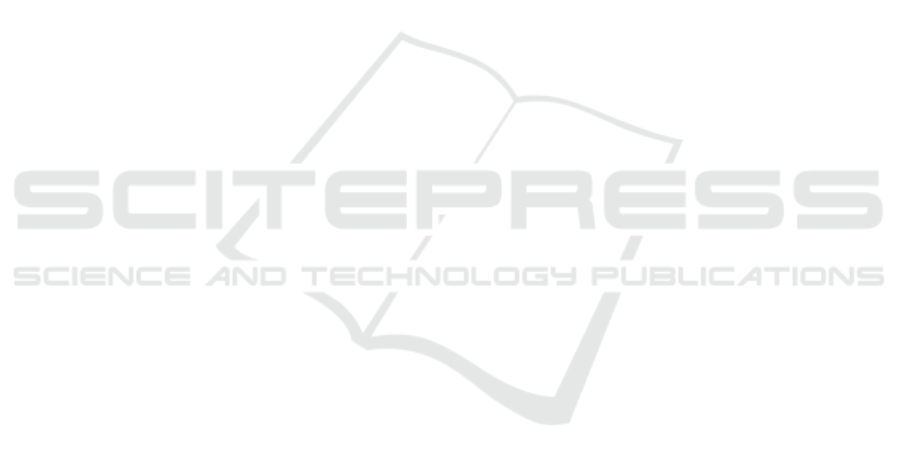
MULTI-SCALE APPROACH TO POPULATION BALANCE
MODELLING OF DISPERSE SYSTEMS
Béla G. Lakatos
Institute of Process Engineering, University of Pannonia, Egyetem Street 10, Veszprém, Hungary
Keywords: Disperse systems, Multi-scale modelling, Population balance, Crystallization, Two-population model.
Abstract: A three-scale model is presented and analysed using the multi-scale methodology of complex systems. The
micro-scale model is formulated as a set of stochastic differential equations for the individual disperse
objects and it is shown that the population balance equation, containing also terms describing collision
interchange of extensive quantities between the disperse elements is a meso-scale model of disperse
systems. The macro-scale model is formulated by means of the moments of internal quantities. As an
example a two-population model, governing the coupled behaviour of crystals and fluid elements is
presented for describing micromixing in solution crystallization.
1 INTRODUCTION
Disperse systems of chemical engineering, contain-
ing large numbers of individual interacting dispersed
objects such as solid particles, liquid droplets or gas
bubbles, or often combinations of those are nonequi-
librium and (usually) nonlinear multi-phase systems.
Their characteristic property is that, not depending
on the nature of disperse elements, a number of in-
teracting size and time scales can be distinguished
and identified therefore these systems, in principle,
belong to the class of complex multi-scale systems .
The multi-scale structure of disperse systems has
been considered relating to different modelling and
computational problems (Eberard et al., 2005; Ing-
ram and Cameron, 2002; Li et al., 2004;
Wei, 2007),
and also in context of population balance models.
(Mazzotti, 2010; Lakatos, 2010). However, the mul-
tiscale nature of the population balance models of
disperse systems has not been analysed in details
yet.
In this paper the population balance models of
disperse systems are analysed applying the multi-
scale methodology of complex systems. The micro-
scale model is formulated as a set of stochastic dif-
ferential equations for the individual disperse objects
with collision interactions and it is shown that the
population balance equation, containing also terms
describing interchange of extensive quantities bet-
ween the disperse elements is a meso-scale model of
disperse systems. The macro-scale model is for-
mulated using the moments of internal quantities. As
an example a two-population model, governing the
coupled behaviour of crystals and fluid elements is
presented for describing micromixing in reaction
crystallization.
2 MICRO-SCALE MODEL
Consider a large population of interacting disperse
objects, solid or fluid particles moving stochastically
in a continuous carrier phase. Let us assume that 1)
the extensive quantities carried by the disperse ele-
ments, such as mass of chemical species and heat are
distributed homogeneously inside those, or internal
motion of those is irrelevant regarding the behaviour
of system; 2) the continuous phase is modelled on
kinetic scale, i.e. by means of concentrations of che-
mical species; 3) collision interactions of disperse
elements may cause their coalescence, aggregation,
breakup as well as interchange of extensive quanti-
ties between the colliding elements. Therefore the
micro-scale of the disperse system can be assigned
by the individual disperse elements.
Assuming that
p
x
and
p
u
denote, respectively,
the space coordinates and velocities along those of a
disperse element, v
p
denotes its volume,
p
c
stands
for the vector of concentrations of K≥0 relevant
chemical species inside the disperse elements and T
p
denotes its temperature. Then the state of a disperse
186
Lakatos B..
MULTI-SCALE APPROACH TO POPULATION BALANCE MODELLING OF DISPERSE SYSTEMS.
DOI: 10.5220/0003620701860191
In Proceedings of 1st International Conference on Simulation and Modeling Methodologies, Technologies and Applications (SIMULTECH-2011), pages
186-191
ISBN: 978-989-8425-78-2
Copyright
c
2011 SCITEPRESS (Science and Technology Publications, Lda.)