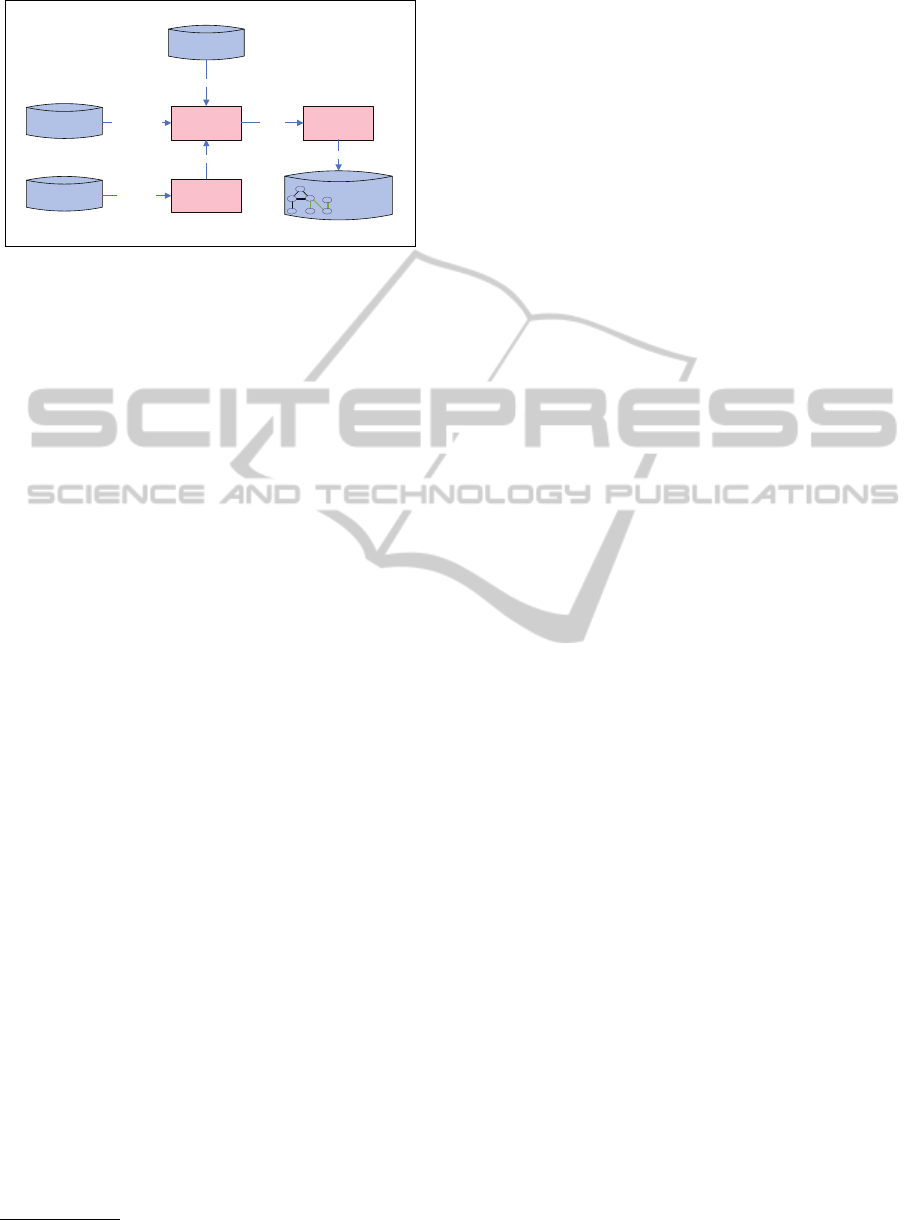
&RQFHSW1HW
:RUG1HW
/,:&
(QULFKPHQW
&RQFHSWV&!
6\QVHWV6!
$QQRWDWLRQV$! $QQRWDWLRQ &RQWH[WXDOL]DWLRQ
&6!
&6$!
&$!
3V\FKR1HW
Figure 1: PsychoNet 2 Building Framework.
text (Gill et al., 2008; Hancock et al., 2008; Hancock
et al., 2007), identifying the gender of bloggers (Now-
son and Oberlander, 2006), recognizing the personal-
ity (Gill, 2003; Mairesse et al., 2007), studying the de-
mographic differentiations across the styles of blog-
gers (Mohtasseb and Ahmed, 2010a), and in author-
ship identification (Mohtasseb and Ahmed, 2009a;
Mohtasseb and Ahmed, 2009b). However, all of these
tasks have been applied on the word level rather than
the concept level, which is available in PsychoNet 1.
The ConceptNet knowledgebase is a semantic
network encompasses the spatial, physical, social,
temporal aspects of everyday life (Liu and Singh,
2004). ConceptNet is generated automatically from
the 700,000 sentences of the Open Mind Common
Sense corpus
1
. ConceptNet is currently considered
to be the largest commonsense semantic network
containing over 250,000 nodes. Nodes are semi-
structured English fragments, interrelated by an on-
tology of twenty semantic relations (predicates). Con-
ceptNet is very useful in describing real life scenes
which makes it a good candidate to be integrated with
LIWC that will add the psycholinguistic dimension.
WordNet is a large lexical database of English
(Miller, 1995). Nouns, verbs, adjectives and adverbs
are grouped into sets of cognitive synonyms (synsets).
It is a very rich domain-independent knowledgebase
of lexical units that consist of various forms of syn-
onyms. WordNet is effective for studying the rela-
tionships within similar words in terms of meaning,
generalization or specialization.
On the other hand, PsychoNet 1 introduced the
first development of ConceptNet towards psycholin-
guistic direction, utilizing LIWC. It has been built by
a fully automated engine that performs lexical anal-
ysis on concepts and extracts the corresponding psy-
cholinguistic categories. It allows the researcher to
use one coherent knowledgebase that has the power of
semantic commonsense and psycholinguistic taxon-
1
http://web.media.mit.edu/ push/OMCS-Research.html
omy. Moreover, PsychoNet 1 simplified applying text
classification tasks in ConceptNet and allows filtering
the huge concept graphs based on a key category for
a specific application. PsychoNet 2 introduces further
improvement on PsychoNet 1 as being explained in
the next section.
3 PsychoNet 2
In PsychoNet 1 (Mohtasseb and Ahmed, 2010b), each
node is a concept associated with a psychometric field
that contains the psycholinguistic categories (annota-
tions) and their relevance degree. In PsychoNet 2,
many limitations have been addressed including miss-
ing concepts and contextualization, and more sub-
stantial improvements are introduced through the ad-
dition of two new stages as depicted in Figure 1.
The first stage, Enrichment, utilizes WordNet to deal
with those concepts, existing in ConceptNet, that do
not have matching LIWC annotations. The resulting
synonym sets, for the original component words, are
then annotated using LIWC. This is explained in de-
tail in Section 3.1. Section 3.2 presents the second
stage, Contextualization, that starts by selecting the
synonym sets that share the same set of annotations.
Then, it deduces the high ranked annotations that po-
tentially represent the context of the concept. The fol-
lowing subsections explain the two new stages; En-
richment and Contextualization, respectively.
3.1 Enrichment
Through our analysis of PsychoNet 1, it has been
found that there were 21498 concepts that have not
been included. Moreover, the analysis showed that
31863 words, which belong to the commonsense con-
cepts, do not have matching LIWC categories. To
address this and try to annotate and include most
concepts, we had to develop a way to enrich LIWC
to include those missing words and their variations.
Therefore, WordNet is utilized here to expand and en-
rich the contents of LIWC based on the commonsense
words of ConceptNet, as explained below.
Assume that W = {w
1
, w
2
, . . . , w
n
} is the set of
commonsense words that do not have LIWC anno-
tations. For each word w
i
∈ W , all synsets (synonym
sets) {S
1
, S
2
, . . . , S
m
}, of this word, are extracted using
WordNet. Hence, S
j
= {s
1
, s
2
, . . . , s
l
} represents one
of the synsets where s
k
is a synonym for w
i
within the
context of the synset S
j
. A
S
j
= {a
1
, a
2
, . . . , a
z
} is the
list of LIWC annotation of S
j
if there were cross joint
annotations across all s
k
. Then, the set of final LIWC
KEOD 2011 - International Conference on Knowledge Engineering and Ontology Development
340