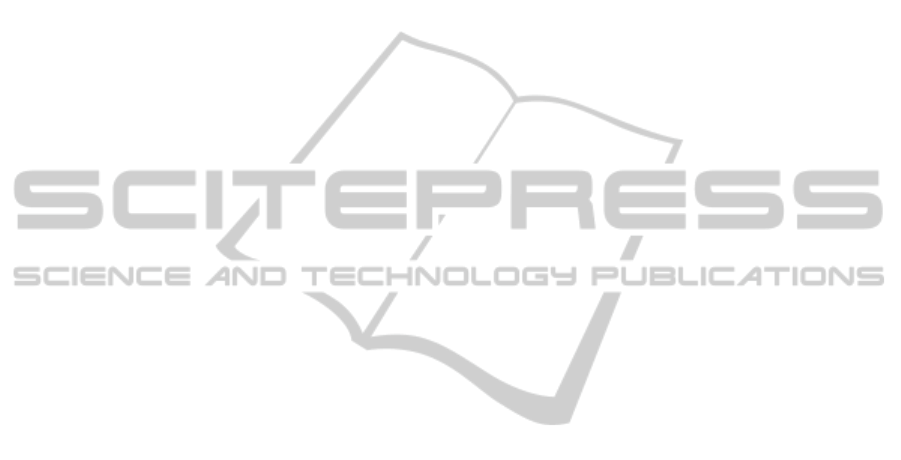
can be applied in a resource-efficient fashion and
yields results that are useful for organizations.
However, there are limitations related to this
work with respect to the Design Science Evaluation
Framework. First, our empirical evidence is based
on several pilot studies but only a single field
application. Further field studies have to be executed
to expand the evaluation of the Col-MM artefacts
and to further enhance the Col-MM. Particular care
will have to be taken to ensure that Col-MM can
take into account all levels of collaboration and all
collaboration processes in an organization in
different settings. This cannot be achieved by just
expanding the number of criteria as this will overly
complicate the use of the model. Second, at this
stage, the Col-MM cannot yet be used to investigate
a correlation between collaboration maturity levels
and organizational/team performance. However, it
provides a first step into this direction.
We recommend several directions for future
research to enhance the current version of Col-MM.
First, the model has to be applied in different types
of organizations for different types of teams. The
experiences from these applications will assist in the
further development and evaluation of the Col-MM
artefacts. Second, organizational and team
performance measures have to be developed to
enable an analysis of the relationship between
collaboration maturity and organizational
productivity. Fourth, from a behavioural science
perspective, some further confirmatory studies
should be performed using Structural Equation
Modelling (Bollen, 1989) to validate the correlation
between these variables (i.e. Col-MM constructs and
performance).
REFERENCES
Banker, R., Bardhan, I., Asdemir, O. (2006).
Understanding the impact of collaboration software on
product design and development, Information Systems
Research, 17, 4.
Bollen K. A. (1989). Structural Equations with Latent
Variables, Wiley.
Boughzala I. (2007). Ingénierie de la collaboration:
théories, technologies et pratiques, Paris, Hermès,
September 2007.
Boughzala, I., Vreede, G. J. de (2012). A Collaboration
Maturity Model: Development and Exploratory
Application, Proceedings of the 45
th
Hawaii
International Conference on System Sciences, Hawaii,
January 2012.
Briggs, R. O., Kolfschoten, G. L., Vreede, G. J. de, &
Dean, D. L. (2006, August). Defining Key Concepts
for Collaboration Engineering, in: Irma Garcia, Raúl
Trejo (eds.). Proceedings of the 12
th
Americas
Conference on Information Systems, Acapulco,
Mexico, 121-128.
Chiesa, V., Coughlan, P. and Voss, C. (1996).
Development of a technical innovation audit. Journal
of Product Innovation Management, 13, 2, 105-136.
Chudoba K. M., Wynn E., Lu M., & Watson-Manheim M.
B. (2005). How virtual are we? Measuring virtuality
and understanding its impact in a global organization,
Information Systems Journal, 15, 279–306.
Clark, K. B., T. Fujimoto. (1991). Product development
performance: Strategy, organization and management
in the world auto industry. Harvard Business School
Press, Boston, MA.
Crosby, P. B. (1979). Quality is Free, McGraw-Hill, New
York.
Damianos L., Hirschman L., Kozierok R., Kurtz J.,
Greenberg A., Holgado R., Walls K., Laskowski S.,
Scholtz J. (1999). Evaluation for Collaborative
Systems, ACM Computing Surveys (CSUR), 31, 2,
Article 15.
Daoudi J., Bourgault M. (2007) What Do We Know About
Collaborative Maturity? 4th Annual Sprott Doctoral
Symposium, April 19-20.
Den Hengst M., Kolfschoten G., Dean D. L., &
Chakrapani, A. (2006). Assessing the quality of
collaborative processes. Proceeding of the 39th annual
Hawaii International Conference on System Sciences,
IEEE Computer Society.
Dooley, K., Subra, A., & Anderson, J. (2001). Maturity
and its Impact on New Product Development Project
Performance, Research in Engineering Design, 13, 30-
29.
Fraser, P., Farrukh, C., & Gregory, M., (2003). Managing
product development collaborations – A process
maturity approach, Proceedings of the Institution of
Mechanical Engineers, 217, 11, 1499-1519.
Fraser, P., Moultrie, J., & Gregory, M., (2002). The use of
maturity models / grids as a tool in assessing product
development capability, IEEE International
Engineering Management Conference, Cambridge,
August 19–20, 2002.
Fraser, P., Moultrie, J., & Holdway, R. (2001).
Exploratory studies of a proposed design maturity
model. 8th International Product Development
Management Conference, University of Twente,
Holland, June 11-12.
Frost and Sullivan (2007). Meetings Around the World:
The Impact of Collaboration on Business
Performance. White paper available at http://news
center.verizon.com/kit/collaboration¬/MAW_WP.pdf.
(Last accessed: 3 April 2011)
Hansen, M. T., Nohria, N. (2004), How to build
collaborative advantage. MIT Sloan Management
Review, 46, 1, 22-30.
Harter, D. E., Krishman, M. S., & Slaughter, S. A. (2000).
Effects of Process Maturity on Quality, Cycle Time,
and Effort in Software Product Development.
Management Science, 46, 4, 451-466.
KMIS 2011 - International Conference on Knowledge Management and Information Sharing
36