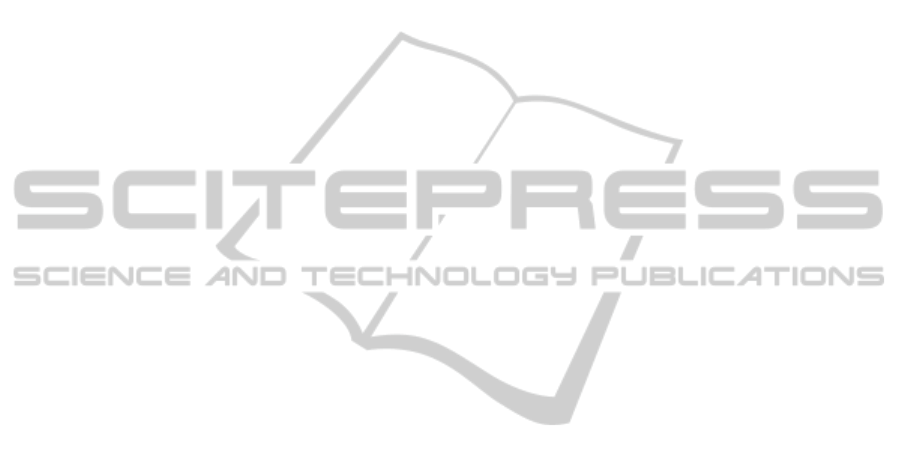
• We also show experimentally that it is able to re-
duce the ambiguity of “weakly labeling” in im-
age annotation, and separate the foreground ob-
jects from the scene in finer levels of the cascade.
The experiments show promising results of the
proposed method in comparison with several base-
lines on Corel5K. Experiments suggest that as long as
the finer levels can bring “newinformation”, they help
to obtain better detection of foreground objects. For
the future work, we would like to focus more on the
role of context in reducing the ambiguity of “weakly
labeling”.
REFERENCES
Akbas, E. and Vural, F. T. Y. (2007). Automatic image an-
notation by ensemble of visual descriptors. In IEEE
Conf. on CVPR, pages 1–8, Los Alamitos, CA, USA.
Andrews, S., Hofmann, T., and Tsochantaridis, I. (2002).
Multiple instance learning with generalized support
vector machines. In 18th AAAI National Conference
on Artificial intelligence, pages 943–944, Menlo Park,
CA, USA.
Barnard, K., Duygulu, P., Forsyth, D., Freitas, N. D., Blei,
D. M., K, J., Hofmann, T., Poggio, T., and Shawe-
taylor, J. (2003). Matching words and pictures. Jour-
nal of Machine Learning Research, 3:1107–1135.
Blei, D. M. and Jordan, M. I. (2003). Modeling annotated
data. In Proc. of the 26th ACM SIGIR, pages 127–134.
Carneiro, G., Chan, A. B., Moreno, P. J., and Vasconcelos,
N. (2007). Supervised learning of semantic classes for
image annotation and retrieval. IEEE Trans. PAMI,
29(3):394–410.
Deselaers, T. and Ferrari, V. (2010). A conditional random
field for multiple-instance learning. In Proc. of The
27th ICML, pages 287–294.
Deselaers, T., Keysers, D., and Ney, H. (2008). Features
for image retrieval: an experimental comparison. Inf.
Retr., 11:77–107.
Douze, M., J´egou, H., Sandhawalia, H., Amsaleg, L., and
Schmid, C. (2009). Evaluation of gist descriptors for
web-scale image search. In Proc. of the ACM CIVR,
pages 1–8, New York, NY, USA.
Duygulu, P., Barnard, K., de Freitas, J. F. G., and Forsyth,
D. A. (2002). Object recognition as machine transla-
tion: Learning a lexicon for a fixed image vocabulary.
In Proc. of the 7th ECCV, pages 97–112, London, UK.
Springer-Verlag.
Feng, S. L., Manmatha, R., and Lavrenko, V. (2004). Mul-
tiple bernoulli relevance models for image and video
annotation. In Proc. of the 2004 CVPR.
Hofmann, T. (1999). Probabilistic latent semantic indexing.
In Proc. of the 22nd ACM SIGIR, pages 50–57, New
York, NY, USA.
J´egou, H., Douze, M., and Schmid, C. (2010). Improving
bag-of-features for large scale image search. Int. J.
Comput. Vision, 87(3):316–336.
Jeon, J., Lavrenko, V., and Manmatha, R. (2003). Au-
tomatic image annotation and retrieval using cross-
media relevance models. In Proc. of the 26th int. ACM
SIGIR, pages 119–126.
Jeon, J., Lavrenko, V., and Manmatha, R. (2004). Auto-
matic image annotation of news images with large vo-
cabularies and low quality training data. In Proc. of
ACM Multimedia.
Kennedy, L. S. and Chang, S.-F. (2007). A reranking ap-
proach for context-based concept fusion in video in-
dexing and retrieval. In Proc. of the 6th ACM int. on
CIVR, pages 333–340, New York, NY, USA. ACM.
Lavrenko, V., Manmatha, R., and Jeon, J. (2003). A model
for learning the semantics of pictures. In Advances
in Neural Information Processing Systems (NIPS’03).
MIT Press.
Lazebnix, S., Schmid, C., and Ponce, J. (2009). Object Cat-
egorization: Computer & Human Vision Perspectives,
chapter Spatial Pyramid Matching. Cambridge Uni-
versity Press.
Makadia, A., Pavlovic, V., and Kumar, S. (2010). Base-
lines for image annotation. Int. J. Comput. Vision,
90(1):88–105.
Maron, O. and Lozano-P´erez, T. (1998). A framework for
multiple-instance learning. In Proc. of the Conf. on
Advances in Neural Information Processing Systems,
NIPS ’97, pages 570–576, Cambridge, MA, USA.
MIT Press.
Monay, F. and Gatica-Perez, D. (2007). Modeling semantic
aspects for cross-media image indexing. IEEE Trans.
Pattern Anal. Mach. Intell., 29(10):1802–1817.
Nguyen, C.-T., Kaothanthong, N., Phan, X.-H., and
Tokuyama, T. (2010). A feature-word-topic model for
image annotation. In Proc. of the 19th ACM CIKM,
pages 1481–1484.
Oliva, A. and Torralba, A. (2001). Modeling the shape of
the scene: A holistic representation of the spatial en-
velope. Int. J. of Comput. Vision, 42:145–175.
Sch¨olkopf, B., Burges, C. J. C., and Smola, A. J., editors
(1999). Advances in kernel methods: support vector
learning. MIT Press, Cambridge, MA, USA.
Szummer, M. and Picard, R. W. (1998). Indoor-outdoor
image classification. In Proc. of the 1998 Int. Work-
shop on Content-Based Access of Image and Video
Databases, page 42, Washington, DC, USA.
Torralba, A., Murphy, K. P., and Freeman, W. T. (2010).
Using the forest to see the trees: exploiting context
for visual object detection and localization. Commun.
ACM, 53(3):107–114.
Viola, P. and Jones, M. (2001). Rapid object detection using
a boosted cascade of simple features. In Proc. of IEEE
CVPR, volume 1, pages I–511 – I–518 vol.1.
Yang, C., Dong, M., and Hua, J. (2006). Region-based
image annotation using asymmetrical support vector
machine-based multiple-instance learning. In Proc. of
the 2006 IEEE CVPR, pages 2057–2063, Washington,
DC, USA.
CASCADE OF MULTI-LEVEL MULTI-INSTANCE CLASSIFIERS FOR IMAGE ANNOTATION
23