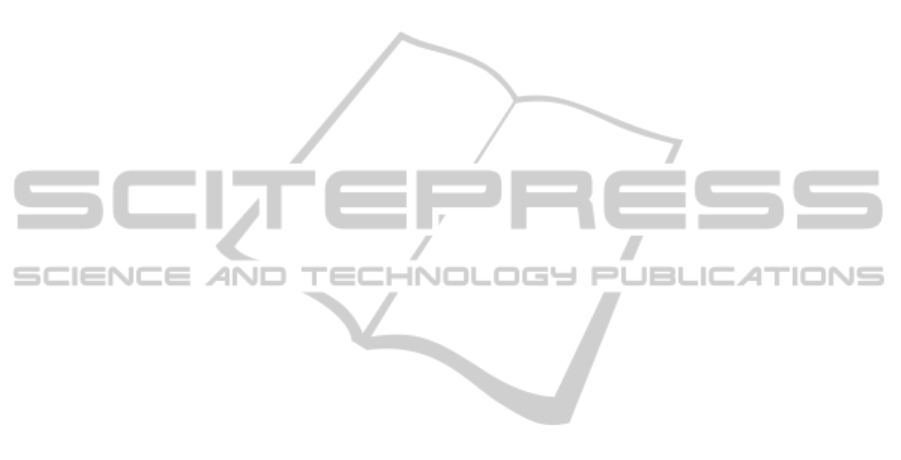
new knowledge which is not yet expressed by the on-
tology thus, our idea was to translate new relations
discovered by the learning process into knowledge
which may be useful to enrich the initial ontology.
6 CONCLUSIONS AND FUTURE
WORKS
In this paper, we have proposed a new two-way ap-
proach, named OOBN-Ontology Cooperation (2OC)
for OOBN structure learning and automatic ontology
enrichment. The leading idea of our approach is to
capitalize on analyzing the elements that are common
to both tasks with the intension of improving their
state-of-the-art methods. In fact, our work is consid-
ered as an initiative aiming to set up new bridges be-
tween PGMs and ontologies. The originality of our
method lies first, on the use of the OOBN framework
which allowed us to address an extended range of on-
tologies, second, on its bidirectional benefit as it en-
sures a real cooperation, in both ways, between on-
tologies and OOBNs.
Nevertheless, this current version is subject to sev-
eral improvements. As a first line of research, we aim
to implement our method and test it on real world ap-
plications based on ontologies, furthermore, in this
work, our aim was to provide a warning system able
to propose a set of possible changes to the ontology
engineers. The discovered relations and / or con-
cepts have to be denominated so, as possible research
direction, we will be interested in natural language
processing (NLP) methods to allow the automation
of this process. Our last perspective concerns the
use of another PGM, Probabilistic Relational Models
(Getoor et al., 2007),whose characteristics are similar
to OOBNs.
REFERENCES
Bangsø, O., Langseth, H., and Nielsen, T. D. (2001). Struc-
tural learning in object oriented domains. In Proceed-
ings of the 14th International Florida Artificial Intel-
ligence Research Society Conference, pages 340–344.
Bangsø, O. and Wuillemin, P.-H. (2000). Object oriented
bayesian networks: a framework for top-down spec-
ification of large bayesian networks with repetitive
structures. Technical report, Aalborg University.
Bellandi, A. and Turini, F. (2009). Extending ontology
queries with bayesian network reasoning. In Proceed-
ings of the 13th International Conference on Intelli-
gent Engineering Systems, pages 165–170, Barbados.
Ben Ishak, M., Leray, P., and Ben Amor, N. (2011).
Ontology-based generation of object oriented
bayesian networks. In Proceedings of the 8th
Bayesian Modelling Applications Workshop, pages
12–20, Barcelona, Spain.
Ben Messaoud, M., Leray, P., and Ben Amor, N. (2011).
Semcado: a serendipitous strategy for learning causal
bayesian networks using ontologies. In Proceedings
of the 11th European Conference on Symbolic and
Quantitative Approaches to Reasoning with Uncer-
tainty, pages 182–193, Belfast, Northern Ireland.
Costa, P. and Laskey, K. (2006). PR-OWL: A framework
for probabilistic ontologies. Frontiers In Artificial In-
telligence and Applications, 150:237–249.
Ding, Z. and Peng, Y. (2004). A probabilistic extension to
ontology language OWL. In Proceedings of the 37th
Hawaii International Conference On System Sciences.
Fenz, S., Tjoa, M., and Hudec, M. (2009). Ontology-based
generation of bayesian networks. In Proceedings of
the 3rd International Conference on Complex, Intelli-
gent and Software Intensive Systems, pages 712–717,
Fukuoka, Japan.
Getoor, L., Friedman, N., Koller, D., Pfeffer, A., and Taskar,
B. (2007). Probabilistic relational models. In Getoor,
L. and Taskar, B., editors, An Introduction to Statisti-
cal Relational Learning, pages 129–174. MIT Press.
Gruber, T. (1993). A translation approach to portable ontol-
ogy specifications. Knowledge Acquisition, 5(2):199–
220.
Helsper, E. M. and van der Gaag, L. C. (2002). Building
bayesian networks through ontologies. In Proceedings
of the 15th European Conference on Artificial Intelli-
gence, pages 680–684, Amsterdam.
Khattak, A. M., Latif, K., Lee, S., and Lee, Y.-K. (2009).
Ontology evolution: A survey and future challenges.
In The 2nd International Conference on u-and e- Ser-
vice, Science and Technology, pages 285–300, Jeju.
Koller, D. and Pfeffer, A. (1997). Object-oriented bayesian
networks. In Proceedings of the 13th Conference on
Uncertainty in Artificial Intelligence, pages 302–313,
Providence, Rhode Island, USA. Morgan Kaufmann.
Langseth, H. and Nielsen, T. D. (2003). Fusion of domain
knowledge with data for structural learning in object
oriented domains. Journal of Machine Learning Re-
search, 4:339–368.
Pearl, J. (1988). Probabilistic reasoning in intelligent sys-
tems. Morgan Kaufmann, San Franciscos.
Stojanovic, L., Maedche, A., Stojanovic, N., and Motik, B.
(2002). User-driven ontology evolution management.
In Proceedings of the 13th International Conference
on Knowledge Engineering and Knowledge Manage-
ment, pages 285–300, Siguenza, Spain.
Yang, Y. and Calmet, J. (2005). Ontobayes: An ontology-
driven uncertainty model. In The International Con-
ference on Intelligent Agents, Web Technologies and
Internet Commerce, pages 457–463, Vienna, Austria.
Zheng, H., Kang, B.-Y., and Kim, H.-G. (2008). An
ontology-based bayesian network approach for rep-
resenting uncertainty in clinical practice guidelines.
In ISWC International Workshops, URSW 2005-2007,
Revised Selected and Invited Papers, pages 161–173.
KEOD 2011 - International Conference on Knowledge Engineering and Ontology Development
194