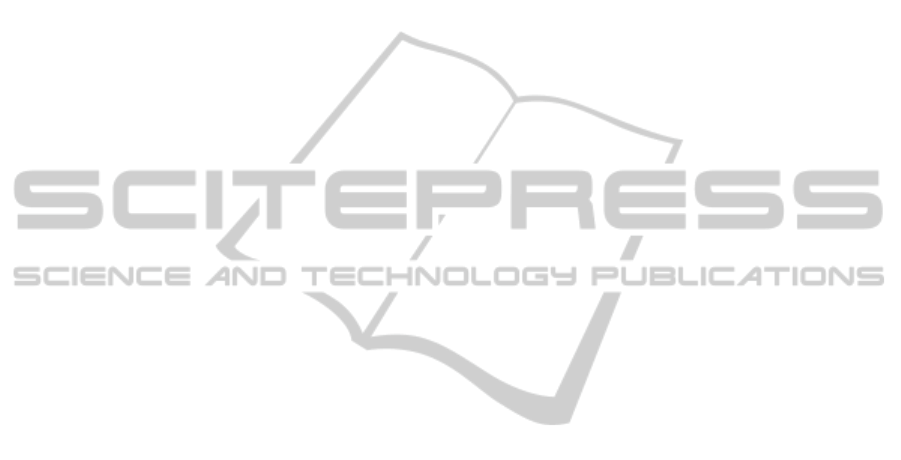
4 CONCLUSIONS AND FUTURE
WORK
In this paper we highlight the necessity to
incorporate the temporal measures in studying the
evolution of geographical objects over the time.
In order to achieve this objective we proposed an
approach aiming to extract knowledge from a
spatiotemporal database by the means of
spatiotemporal mining queries merging both data
mining and time management concepts.
As future work, the most meaningful rules will
be processed by a learning system (e.g. neural
network) in order to identify unknown future risks.
We will also evaluate the possibility to
implement our approach in a wider range of risk
prediction applications including appropriate input
parameters suitable for the study region and the kind
of risk to predict.
REFERENCES
Agrawal, R., Srikant, R. 1994. Fast algorithms for mining
association rules in large databases. Research Report
RJ 9839, IBM Almaden Research Center, San Jose,
California.
Bogorny, V., 2006. Enhancing spatial association rule
mining in geographical database. Thesis presented In
partial fulfillment of the requirements for the degree of
doctor in computer science, federal university of” Rio
Grande du sul”, Porto Alegre.
Bogorny, V., Bart, K., Luis, O., 2008. A Spatio-temporal
Data Mining Query Language for Moving Object
Trajectories. Technical Report TR-357. Federal
University of Rio Grande do Sul, Porto Alegre, Brazil.
Boulicaut, J. F. and Masson, C., 2005, Data Mining Query
Languages. In The Data Mining and Knowledge
Discovery Handbook, O. Maimon and L. Rokach
(Eds) (Springer), pp. 715{727.
Chen, X., Zaniolo, C., 2000. SQL
ST
: A Spatiotemporal
Data Model and Query Language. International
Conference on Conceptual Modeling.
Chen, X., Petrounias, I., 1998, Language Support for
Temporal Data Mining. In Proceedings of the
Proceedings of the Second European Symposium on
Principles of Data Mining and Knowledge Discovery
(London, UK: Springer-Verlag), pp. 282{290.
Dbminer Technology 2000.Inc. DBMiner Enterprise 2.0.
Available at DBMiner Technology site. URL: http://
www.dbminer.com.
Erlend, T., Mads, N., 2010. Representing topological
relationships for spatiotemporal objects. In
GEOINFORMATICA. (published with open access at
Springerlink.com).
Fayyad, U., Piatesky-Shapiro, G., Smyth, P., 1996. From
Data Mining to Knowledge Discovery: An Overview.
Advances in KDD and Data Mining, AAAI,
Han, J., Koperski, K., Stefanovic, N., 1997. GeoMiner: A
System Prototype for Spatial Data Mining. In ACM-
SIGMOD Int’l Conf. On Management of Data
(SIGMOD'97), Tucson, Arizona.
Han, J., Fu, Y., Wang, W., Koperski, K. and Zaiane, O.,
1996, Dmql: A data mining query language for
relational databases. In Proceedings of the
SIGMOD'96 Workshop on Research Issues in
DataMining and Knowledge Discovery, Montreal,
Canada, pp. 27{33.
Han, J., 1995, Mining Knowledge at Multiple Concept
Levels. In Proceedings of the CIKM (ACM), pp.
19{24.
Imielinski, T. and Virmani, A., 1999, MSQL: A Query
Language for Database Mining. Data Mining and
Knowledge Discovery, 3, 373{408.
Koperski, K., 1999. A progressive refinement approach to
spatial data mining. Doctorate Thesis. In Simon Fraser
University.
Koperski, K., Han, J., 1995. Discovery of spatial
association rules in Geographic Information
Databases. In proc.4
th
Int’Symp on large Databases
(SSD’95), pp47-66, Portland.
Manco, G., Baglioni, M., Giannotti, F., Kuijpers, B.,
Raffaet, A., Renso, C., 2008. Querying and Reasoning
for Spatiotemporal Data Mining F. Giannotti and D.
Pedreschi (eds.) Mobility, Data Mining and Privacy.c
Springer-Verlag Berlin Heidelberg .
Marcelino, P., and Robin, J., 2004. SKDQL, a structured
language to specify knowledge discovery processes
and queries. Lecture Notes in Artificial Intelligence
3171, Springer.
Malerba, D., Appice, A. and Ceci, M., 2004, A Data
Mining Query Language for Knowledge Discovery in
a Geographical Information System. In Proceedings of
the Database Support for Data Mining Applications,
pp. 95{116.
Meo, R., Psaila, G. and Ceri, S., 1996, A New SQL-like
Operator for Mining Association Rules. In
Proceedings of the VLDB, T.M. Vijayaraman, A.P.
Buchmann, C. Mohan and N.L. Sarda (Eds) (Morgan
Kaufmann), pp. 122{133
Roshan, N., Asghar, A., 1996. The Management of Spatio-
Temporal Data in a National Geographic Information
System. Springer.
Turki, Y., Faïz, S., 2009. Apport des règles d'association
spatiales pour l'alimentation automatique des bases de
données géographiques. International journal of
Geomatic. Hermès–Lavoisier editions, Paris, France,
Vol. 19/ N°1/2009, pp.27-44.
Wang, H. and Zaniolo, C., 2003, ATLaS: A Native
Extension of SQL for Data Mining. In Proceedings of
the SDM, D. Barbar_a and C. Kamath (Eds) (SIAM).
Zeitouni, K., Yeh, L., 1999. Les bases de données
spatiales et le data mining spatial. International
journal of Geomatic, Vol 9, N° 4.
QUERYING AND MINING SPATIOTEMPORAL ASSOCIATION RULES
405