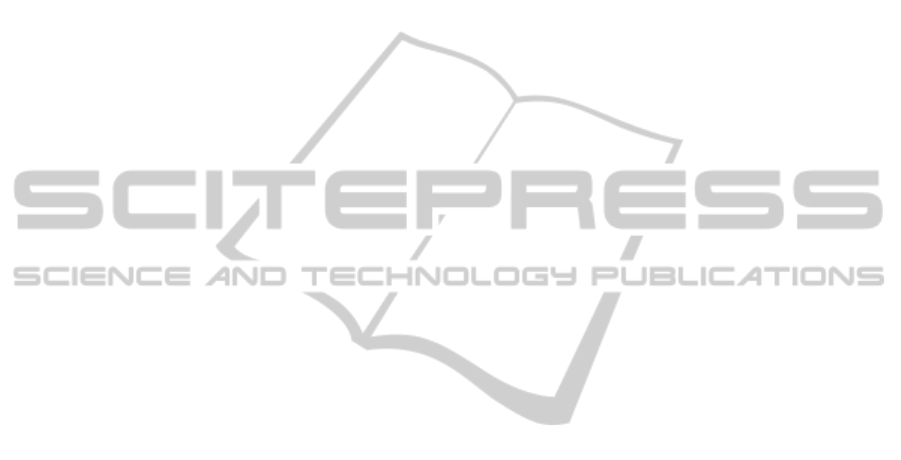
(market complaints) to less than 1/20th their original
incidence.
4.2 Application to Similar Problems
With its effectiveness verified, the authors were able
to apply the New Development Design CAE
Employment Model to critical development design
technologies for automotive production, including
predicting and controlling the special characteristics
of automobile lifting power, anti-vibration design of
door mirrors (Amasaka, 2010b), urethane seat foam
molding (Amasaka, 2007), and loosening bolts
(Yamada and Amasaka, 2011:). In each of these
cases as well, discrepancy was 3–5% versus
prototype testing. Based on the achieved results, the
model is now being used as an intelligent support
tool for optimizing product design processes.
5 CONCLUSIONS
This research addresses the necessity reforming the
business processes associated with development
design, a critical component of manufacturing, by
proposing a New Development Design CAE
Employment Model that takes manufacturers away
from conventional preproduction and prototype
testing methods and towards a better predictive
evaluation method. The effectiveness of the model
was verified by successfully applying it to the
technological problem of development design
bottlenecks at auto manufacturers. The author wants
to make it further established as "Optimal CAE
Design Approach Mode" by deployment of this
model from now on.
ACKNOWLEDGEMENTS
The author would like to acknowledge the generous
support received from the following researchers. All
those at Working Group No. 4) studies in JSQC,
study group on the ideal situation for quality
management in the manufacturing industry, and
those connected with the Amasaka laboratory at
Aoyama Gakuin University.
REFERENCES
Amasaka, K., 2004, Science SQC, New Quality Control
Principle – The Quality Control of Toyota, Springer.
Amasaka, K., 2005a, Constructing a Customer Science
Application System “CS-CIANS”, WSEAS
Transactions on Business and Economics, Issue 3,
Vol. 2, pp. 135-142.
Amasaka, K.,, 2005b, Interim Report of WG4’s studies in
JSQC Research on Simulation and SQC(1)-A Study of
the High Quality Assurance CAE Model for Car
Development Design,, 1st Technical Conference
Nagano, Japan, pp. 93-98.
Amasaka, K., 2007, Highly Reliable CAE Model, The
Key to Strategic Development of Advanced TDS,
Journal of Advanced Manufacturing Systems, Vol. 6,
No. 2, pp. 159-176.
Amasaka, K., 2008, An Integrated Intelligence
Development Design CAE Model Utilizing New JIT,
Journal of Advanced Manufacturing Systems, Vol. 7,
No. 2, pp. 221-241.
Amasaka. K., 2010a. Chapter 4.Product Design, Quality
Assurance Guidebook, New ed,. JSOC, pp. 87-101.
Amasaka, K., 2010b, Proposal and Effectiveness of a High
Quality Assurance CAE Analysis Model, Current
Development in Theory and Applications of Computer
Science, Engineering and Technology, Vol. 2, No. 1/2,
August/November Issue, pp. 23-48.
Amasaka, K., (Ed), 2007a, Establishment of a Needed
Design Quality Assurance Framework for Numerical
Simulation in Automobile Production, Working Group
No. 4 Studies in JSQC.
Amasaka, K., (Ed)., 2007b, New Japan Model - Science
TQM, Study Group on the Ideal Situation of Quality
Management in the Manufacturing Industry, Maruzen.
Ito, T., Sato, M., Yamaji, M., and Amasaka, K., 2010a, An
Analysis of Bottleneck Technology by Using
Experiments and CAE, International Business &
Economics Research Journal, Vol. 9, No. 12, pp. 123-
129.
Ito, T., Matsuura, S., and Amasaka, K., 2010b, Optimized
Design using High Quality Assurance CAE, The
Japanese Society for Quality Control, The 40th
Annual Technical Conference, Seijyo University,
Japan, pp.189-192.
Dong, Y, Fan, X,, and Liu, B., 2011, Numerical
Simulation and Technology Optimization of Rear Oil
Seal Bearing Die Casting Based on ProCast,
Advanced Materials Research, Volx, 89-193, pp. 189-
193.
Leo, J. D. V., Annos, N., and Oscarsson, 2004, Simulation
Based Decision Support for Manufacturing System
Life Cycle Management, Journal of Advanced
Manufacturing Systems, Vol. 3, No. 2, pp. 115-128.
Kameike, M., Ono, S., and Nakamura, K., 2000, The
Helical Seal: Sealing Concept and Rib Design Sealing
Technology, International 77 pp. 7-11.
Kume, H., 1999, Quality Management in Design
Development, JUSE.
Lopez, A.M., Nakamura, K., and Seki, K., 1997, A Study
on the Sealing Characteristics of lip Seals with Helical
Ribs, in Proc. 15th Int. Conf. British Hydromechanics
Research Group Ltd Fluid Sealing, pp. 1-11.
A NEW DEVELOPMENT DESIGN CAE EMPLOYMENT MODEL - Applying Numerical Simulation to Automobile
Bottleneck Technology
123