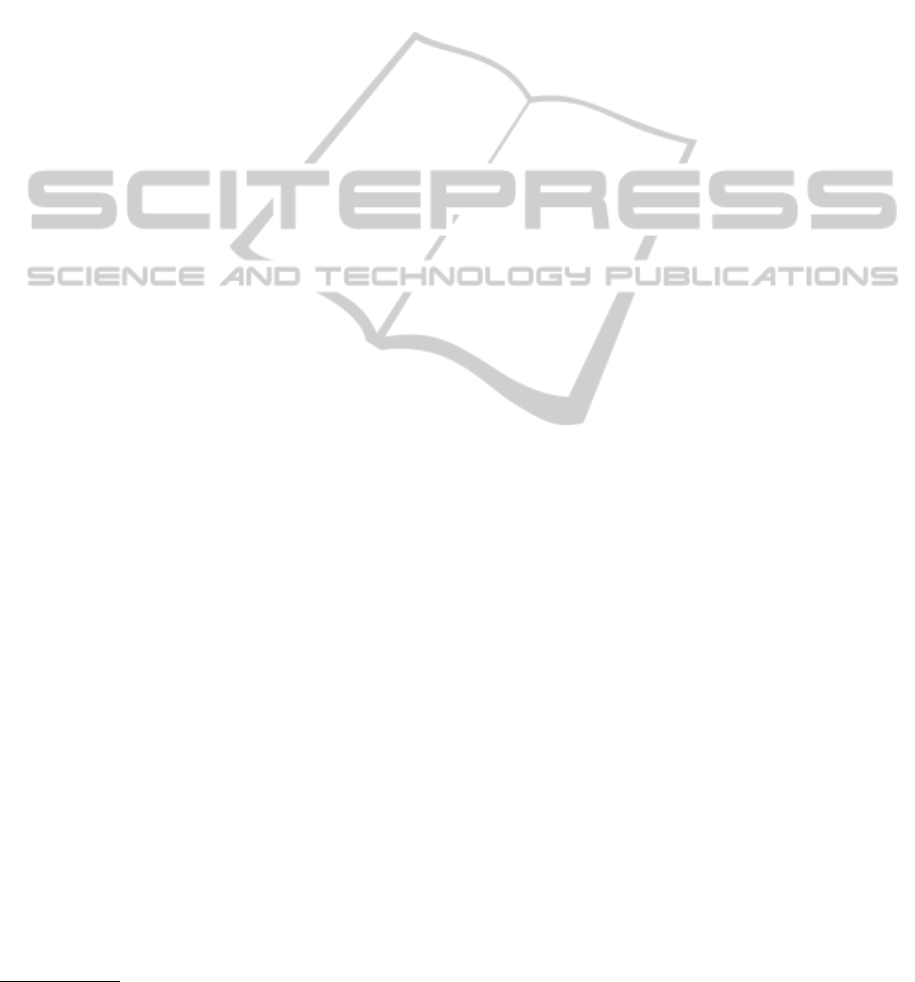
clustering algorithm (as implemented in the Phylip
Package
2
). In Fig. 3 two trees are reported, namely
the ground truth one and the one obtained with the -1
map.
Rather than looking at the global similarity be-
tween trees, a perceptive comparison should focus
mostly on the local matches. This is because, above a
defined cutoff, distances between entries tend to be
less consistent. Indeed, the map-driven tree repro-
duced nicely some of the trends recorded by the dock-
ing ranks. For instance, entries 1GNG, 1I09, 2O5K
and 1H8F were singletons, according to the ground
truth. As illustrated in Fig. 3, the oxygen map-
driven tree put 5 entries distant from the rest. Four
of those entries were indeed the ground truth single-
tons, while the remaining entry (i.e. 1QW3) was er-
roneously recognized as close to 1H8F. From a bio-
logical perspective, 3 out of those 4 entries were pe-
culiar cases, being the only proteins of the set whose
crystals lacked a molecule bound. Another very inter-
esting achievement is the pairing of 1J1B and 1J1C.
Those Xray structures in fact showed very similar
molecules bound, which in turn yielded highly com-
parable conformational rearrangements. Another no-
table result was found for the cluster composed of
entries 3L1S, 1UV5, 1Q41, 1Q5K and 314B, which
perfectly matched the one found in the ground truth.
Entries 3F88, 3DU8 and 1PYX clustered together in
the map-driven tree. The same trend was found in the
ground truth, with the exception of entry 3F7Z, which
was missing in the former. Overall, the remarkable
resemblance between the ground truth and the map-
driven trees speaks to the accuracy of the proposed
methodology in finding hidden relevant chemical pat-
terns in protein structures. Nonetheless, there is room
for improvement. For instance, in a less reductionist
approach, more atom probes could be used.
5 CONCLUSIONS
In this paper, we proposed a novel computational ap-
proach to comparing two or more proteins, starting
from a physico-chemical description of their binding
site (atomic grid maps). These maps were prepro-
cessed via a chemically plausible procedure that sim-
plified the data while retaining the relevant informa-
tion. Different alignment-based similarity measures
were proposed based on a rigid registration algorithm.
The proposed approach was tested on a real dataset in-
volving 22 proteins. Retrospective evaluations, both
2
All information on software and models could be found
at http://evolution.gs.washington.edu/phylip.html.
qualitative and quantitative, proved the feasibility of
the method.
ACKNOWLEDGEMENTS
We kindly acknowledge the IIT computational plat-
form initiative for providing computer time. We
thank Grace Fox for editing and proofreading the
manuscript.
REFERENCES
Berman, H., Henrick, K., and Nakamura, H. (2003). An-
nouncing the worldwide protein data bank. Nat Struct
Biol, 10:980.
Besl, P. and McKay, N. (1992). A method for registration
of 3d shapes. IEEE Trans. on Pattern Analysis and
Machine Intelligence, 14:239–256.
Bicego, M., Dellaglio, F., and Felis, G. (2007). Multimodal
phylogeny for taxonomy: Integrating information
from nucleotide and amino acid sequences. J. Bioin-
formatics and Computational Biology, 5(5):1069–
1085.
Bottegoni, G., Rocchia, W., Rueda, M., Abagyan, R., and
Cavalli, A. (2011). Systematic exploitation of multi-
ple receptor conformations for virtual ligand screen-
ing. Plos One. in press.
Chen, Y. and Crippen, G. (2005). A novel approach to struc-
tural alignment using realistic structural and environ-
mental information. Protein Sci, 14:2935–2946.
Chen, Y. and Medioni, G. (1992). Object modeling by reg-
istration of multiple range images. Image Vision Com-
puting, 10:145155.
Duin, R. and Tax, D. (2000). Experiments with classifier
combining rules. In Proc. Workshop on Multiple Clas-
sifier Systems, pages 16–29.
Favia, A. (2011). Theoretical and computational ap-
proaches to ligand-based drug discovery. Frontiers in
Bioscience, 16:1276–1290.
Fred, A. and Jain, A. (2005). Combining multiple
clusterings using evidence accumulation. IEEE
Trans. on Pattern Analysis and Machine Intelligence,
27(6):835–850.
Fumera, G. and Roli, F. (2005). A theoretical and experi-
mental analysis of linear combiners for multiple clas-
sifier systems. IEEE Trans. on Pattern Analysis and
Machine Intelligence, 27(6):942–956.
Hernandez, F., De Barreda, E., Fuster-Matanzo, A., Goni-
Oliver, P., Lucas, J., and Avila, J. (2009). The role of
gsk3 in alzheimer disease. Brain Res Bull, 80:248–
250.
Ho, T., Hull, J., and Stihari, S. (1994). Decision combi-
nation in multiple classifier systems. IEEE Trans. on
Pattern Analysis and Machine Intelligence, 16(1):66–
75.
AN INNOVATIVE PROTOCOL FOR COMPARING PROTEIN BINDING SITES VIA ATOMIC GRID MAPS
421