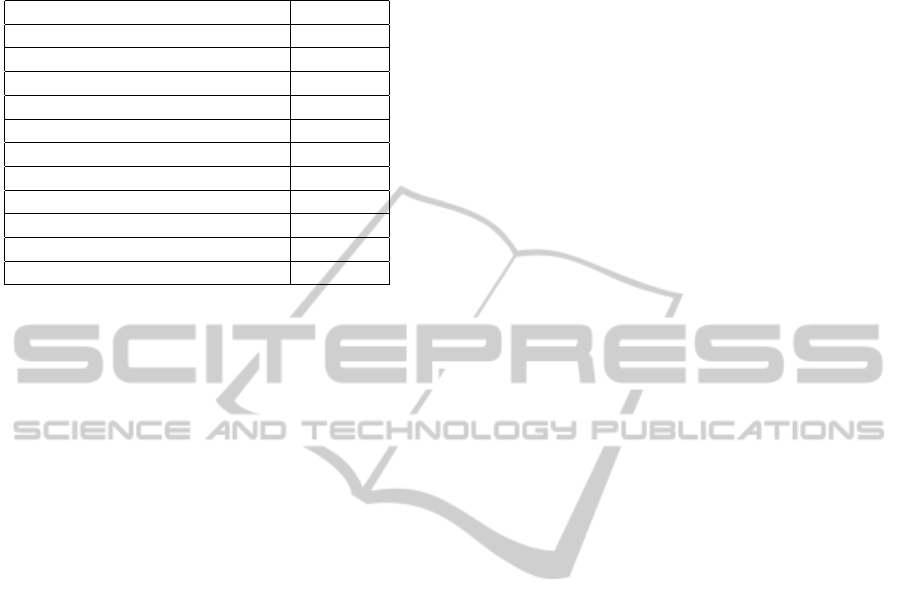
Table 4: Results for the MacKey-Glass time series. Re-
gression scores from (Jang, 1997), (Kim and Kim, 1997),
(Wang, 1992) and (Lee and Kim, 1994).
System RMSE
Linear regression model 0.55
Auto regressive model 0.19
Sixth order polynomial 0.04
Back propagation NN 0.02
GA and fuzzy system (5 MFs) 0.049206
GA and fuzzy system (7 MFs) 0.042275
GA and fuzzy system (9 MFs) 0.037873
Wang Product T-norm 0.907
Wang Min T-norm 0.904
ANFIS 0.007
P2-TSK-GP (3 MFs) - this paper 0.036548
several advantages over past related research. Firstly,
the system is capable to automatically produce arbi-
trarily large and complex TSK fuzzy systems, accord-
ing to the needs of a specific problem. Secondly, it
provides flexibility in the selection of non-linear func-
tions fired per rule. Finally, the output model is inter-
pretable by humans in contrast to some other models
like MLPs, since it is in the form of fuzzy rules. In this
paper we have presented preliminary results of the ex-
periments which while focusing on certain character-
istics and capabilities of the GP algorithms produced
encouraging results warranting further investigations.
Further research will be primarily focused on an
advanced grammar design for efficient combinations
of polynomials. Increasing the number of the mem-
bership functions is also expected to improve the ac-
curacy of the system. Integration with ensemble sys-
tems will also be considered.
ACKNOWLEDGEMENTS
The research leading to these results has received
funding from the European Commission within the
Marie Curie Industry and Academia Partnerships and
Pathways (IAPP) programme under grant agreement
n. 251617.
REFERENCES
Alba E., Cotta C., Troya J. M., Evolutionary Design of
Fuzzy Logic Controllers Using Strongly-Typed GP, In
Proc. 1996 IEEE Int’l Symposium on Intelligent Con-
trol New York, NY., 127-132, 1996.
Chen Y., Yang B., Abraham A., Peng L., Automatic Design
of Hierarchical Takagi-Sugeno Type Fuzzy Systems
Using Evolutionary Algorithms IEEE Trans. on Fuzzy
Systems , 15:3, 2007.
Delgado M. R., Zuben F. V., Gomide F. , Coevolutionary
genetic fuzzy systems: a hierarchical collaborative ap-
proach Fuzzy Sets and Systems , Elsevier, 141, 89-106,
2004.
Fern´andez F., Tommassini M.,Vanneschi L., An Empiri-
cal Study of Multipopulation Genetic Programming
Genetic Programming and Evolvable Machines 4:1,
Kluwer, 2003.
Hoffman F., Nelles O., Genetic Programming for Model Se-
lection of TSK-Fuzzy Systems Information Sciences,
Elsevier, 136, 7-28, 2001.
Jang J-S. R., Sun C. T., Mizutani E.,Neuro-Fuzzy and Soft
Computing , Prentice Hall, NJ, 1997.
Jang J-S. R., Neuro-fuzzy modeling for nonlinear dynamic
system identification in: Ruspini E. H. , Bonissone P.
P. , Pedrycz W., eds., Handbook of fuzzy computation ,
Institute of Physics Publishing, Dirak House, Temple
Back, Bristol, UK 1998.
Kim D., Kim C., Forecasting time series with genetic fuzzy
predictor ensemble,IEEE Trans. Fuzzy Syst., 5:4, 523-
535, 1997.
Koza J. R. Genetic programming - On the programming of
computers by means of natural selection The MIT
Press, Cambridge, Massachussets, USA, 1992.
Lee S.-H., Kim I., Time series analysis using fuzzy learn-
ing, inProc. Int’l Conf. Neural Inform. Process., Vol.
6, Seoul, Korea, 1577-1582, 1994.
Montana D. J., Strongly Typed Genetic Programming, Evo-
lutionary Computation, 3:2, 1995.
Wang L. X., Mendel J. M.,Generating fuzzy rules by learn-
ing from examples,IEEE Trans. Systems, Man and Cy-
bernetics, 22:6, 1414-1427, 1992.
Yeh, I-C., Modeling slump of concrete with fly ash and su-
perplasticizer, Computers and Concrete, 5:6, 559-572,
2008.
EVOLVING TAKAGI-SUGENO-KANG FUZZY SYSTEMS USING MULTI POPULATION GRAMMAR-GUIDED
GENETIC PROGRAMMING
281