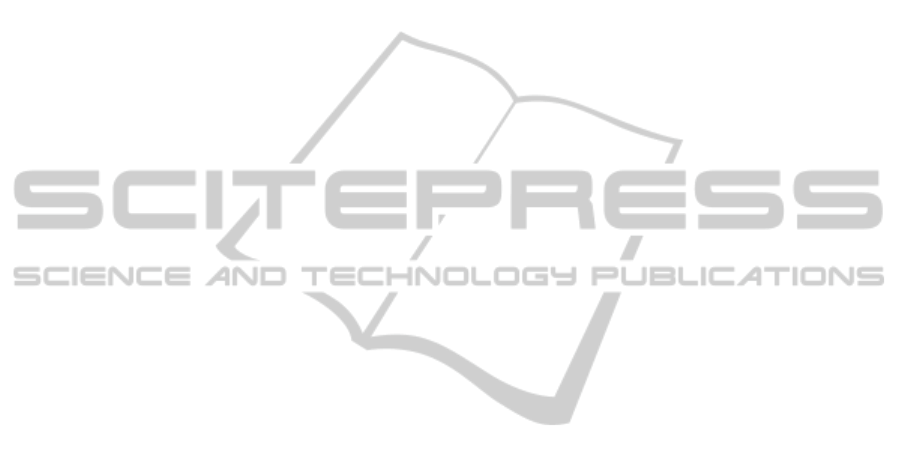
representation of Data
obs
. The second dataset,
Data
expert
, representing the expertise, was used to
decode the SeaWiFS images. The principle of the
method is to compare the ten-component vectors of
Data
expert
whose associated parameters are known,
with those of the neurons of SOM-A-S according to
a distance. At the end of the labeling, each neuron of
SOM-A-S map has captured a set of
ertexp
ρ
and
takes a label, which is extracted from that set
according to the procedure described in Niang et al.,
(2006). The only difference between the two
versions being that the old one uses a first map to
determine 10 different classes of angles, each one
giving rise to a dedicated SOM map for the
classification of the reflectance spectra, while SOM-
A-S uses a unique map doing a data fusion between
the viewing angles and the spectra. By using a
unique map, we avoided the threshold effect that is
induced by the two steps classification (angle and
then reflectance) and the eleven SOM maps
described in Niang et al., (2003).
Each neuron is therefore associated with an
atmospheric and ocean physical parameters (
τ,
C)
and an aerosol type. The SOM-A-S map being
labelled, we are able to analyze a satellite image by
projecting the ten component vector (reflectances
and viewing angles) associated with each pixel on
the SOM-A-S map. Pixels captured by a neuron are
assigned to the aerosol type and optical thickness
associated with this neuron. For monthly
climatology images, the aerosol type is estimated as
the median of the types of the images considered.
The second statistical model improves the
retrieval of the optical thickness. We used a neuro-
variational algorithm, called NeuroVaria, that is able
to provide accurate atmospheric corrections for
inverting satellite ocean-colour measurements. The
algorithm minimizes a weighted quadratic cost
function, J, by adjusting control parameters
(atmospheric and oceanic) such as
τ
and C (Brajard
et al., 2008). J describes the difference between the
satellite measurement
ρ
obs
and a simulated
reflectance
sim
computed using radiative transfer
codes modelled by supervised neural networks (the
so called Multi-Layer-Perceptrons, MLP). The
minimization implies the computation of the
gradient of J with respect to the control parameters
and consequently of the derivatives of the MLPs,
which is done by the classical gradient back-
propagation algorithm (Bishop, 1995). The novelty
of the version of NeuroVaria developed in this work
is that the MLPs modelling the radiative transfer
codes were specially designed to take African dusts
into account. Moreover we used the atmospheric
parameter values given by SOM-A-S and validated
using in situ data (see section 4), as first guesses of
the NeuroVaria algorithm minimization. Since the
efficiency of a minimizing procedure depends on the
first guesses of the control parameters, we expect to
improve the accuracy of the retrieved parameters.
Using these two statistical models sequentially is
indeed a mixed neuro variational method. We
denoted it in the following by SOM-NV.
4 VALIDATIONS OF THE
AEROSOL PARAMETERS
USING SOM-A-S
As SOM-A-S takes into account Saharan dusts, the
number of pixels processed is an order of magnitude
higher than that processed by the standard SeaWiFS
algorithm. As an example, on October 07 2003,
SOM-A-S processed 29,083 pixels while SeaWiFS
processed 16,193 pixels only; on October 12 SOM-
A-S processed 30,300 pixels and SeaWiFS 3,338
only. Besides a statistical comparison between the
SOM-A-S and SeaWiFS algorithms was made for
values of
<0.35. The Mean Relative Error (MRE)
remains low (22.88% for October 07 2003 and
16.16% for October 12 2003) and the Root Mean
Square Error (RMSE) was less than 0.04 for both
days. As a preliminary conclusion, the values
retrieved by SOM-A-S seem consistent with and
very close to those retrieved by the classical
algorithm of SeaWiFS for
<0.35.
The Angström exponent
α
(500,870) provided by
AERONET, allows us to attempt to validate the dust
aerosol type provided by SOM-A-S. Since the sun
photometer does not give the aerosol type, it is
thought possible to validate the dusts by studying the
behavior of
α
(500,870). The low
α
(500,870)
values (
α
<0.5) result from the presence of large
particles typical of desert dusts (Nobileau et al.,
2005). In Figure 1 we show the distribution of the
α
(500,870) of the Dakar-M’Bour AERONET
measurements for the dusty and the non-dusty days
determined by SOM-A-S on the SeaWiFs collocated
pixels. The confidence interval of the average value
of
α
(500,870) calculated by SOM-A-S from
SeaWiFs measurements for the dusty days was
between 0.40 and 0.47, whereas it was between
0.61-0.79 for non-dusty days. This means that the
dust classification provided by SOM-A-S is in
agreement with the AERONET measurements,
which permits us to distinguish the dust absorbing-
aerosols from the non-absorbing ones by processing
RETRIEVING AEROSOL CHARACTERISTICS FROM SATELLITE OCEAN COLOR MULTI-SPECTRAL SENSORS
USING A NEURAL-VARIATIONAL METHOD
299