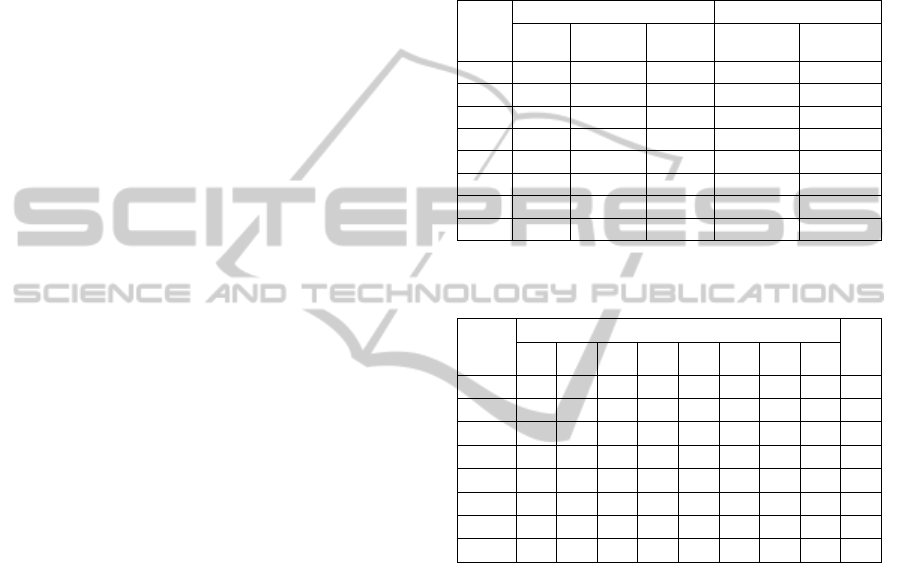
efficiency values associated to the f-th supplier for
f=1,2,…,F are evaluated by averaging the overall
cross-efficiency index
CE
f
computed for all the
iterations, so that a ranking of the suppliers is
established by the descending order of such values.
Summing up, the proposed method integrates
both the advantages of traditional DEA (evaluating
the supply efficiency and avoiding data
normalization) and cross-efficiency DEA
(optimizing the weight set so as to shift from self-
evaluation to peer-evaluation) with respect to
alternative supplier selection techniques. In addition,
the technique is able to deal with uncertain data in
the supply, typical of the real context.
4 USING THE STOCHASTIC DEA
MODEL FOR THE SUPPLIER
SELECTION OF AN SME
We apply the described model for the cross-
efficiency evaluation of suppliers to the supplier
selection process of a small enterprise of southern
Italy, operating in the areas of sale, set-up, and
maintenance of hydraulic plants. Before the
subsequently reported investigation, the case study
SME used the classical lowest price approach to
choose suppliers. On the contrary, thanks to the
proposed supplier selection tool, it was able to
compare the performance of suppliers that it
employed in the past with that of other potential
partners, based not only on price but also
considering additional uncertain data. The
considered supply refers to a specific component, a
cast iron mainspring check valve. While for the
current suppliers the available data are deterministic,
the uncertainty referring to some data of the
performance of potential partners was modelled
using Monte Carlo simulation and the previously
introduced Beta-PERT distribution. In particular, we
consider two currently existing suppliers (denoted
by E1 and E2) for the product supply. Their
performance is compared to that of six potential
additional suppliers (P1, P2, P3, P4, P5, and P6).
The considered efficiency indicators are five. In
particular, three are input indices, i.e., refer to
resources that are required to the SME in the supply
process: 1) component purchasing price (in
€); 2) lead time for the order execution, i.e., time
elapsing between order emission and product
delivery (in days, d); and 3) geographical distance
between supplier and SME (in Km). In addition, we
consider two output indices: 1) quality of the
provided component, defined as a percentage ratio
between working components and overall number of
delivered components (%); and 2) delivery
reliability, expressed as a percentage ratio between
orders that are dealt with on time and overall number
of orders (%).
Table 1: Input and output data for current and potential
suppliers.
Suppl.
Input criteria Output criteria
Price
[€]
Lead Time
[d]
Distance
[Km]
Quality [%]
Reliability
[%]
E1
110 40 1000 99.3 50
E2
91 50 900 99 37.5
P1
95 (30,35,45) 970 (90,95,99) (30,50,75)
P2
78 (42,45,50) 20 (90,92,95) (30,50,75)
P3
132 (32,40,50) 833 (96,99,100) (40,60,85)
P4
130.67 (32,40,50) 897 (90,95,99) (40,60,75)
P5
114.50 (35,45,50) 813 (90,95,99) (35,55,77)
P6
133.10 (32,40,50) 898 (90,95,99) (50,60,80)
Table 2: Cross-efficiency matrix and average cross-
efficiency index of suppliers.
Suppl.
CE
fi
- Efficiency with respect to
CE
f
Cross
Eff.
E1 E2 P1 P2 P3 P4 P5 P6
E1
0.95 0.87 0.84 0.02 0.83 0.87 0.89 0.85 0.77
E2
0.82 0.94 0.70 0.02 0.62 0.66 0.74 0.63 0.64
P1
0.99 0.95 1.00 0.02 0.90 0.93 0.96 0.92 0.83
P2
0.97 1.00 0.82 1.00 0.85 0.90 0.99 0.87 0.92
P3
0.94 0.75 0.84 0.03 0.97 0.92 0.92 0.91 0.79
P4
0.90 0.73 0.82 0.02 0.88 0.94 0.89 0.89 0.76
P5
0.87 0.79 0.78 0.02 0.80 0.84 0.91 0.82 0.73
P6
0.92 0.74 0.84 0.02 0.91 0.93 0.92 0.96 0.78
With reference to the data, we remark that
purchasing prices and geographical distances are
deterministic values. Indeed, prices may be
determined using the price list of the suppliers,
neglecting price variations during the year. On the
contrary, the remaining indices, i.e., lead time,
quality, and reliability, are deterministically defined
for the current suppliers E1 and E2, using historical
data of the SME, while they can be only
stochastically defined for potential suppliers using
the previously described the Beta-PERT approach.
Table 1 summarizes the problem data. For each of
the eight considered suppliers, we evaluate its cross-
efficiency value according to the presented method.
Due to the presence of stochastic elements among
the problem data, we solve the problem using the
described Monte Carlo simulation, thanks to 1000
iterations that are executed in the well-known
MATLAB software environment. The results of the
procedure are in Table 2. The last column of the
KMIS 2011 - International Conference on Knowledge Management and Information Sharing
156