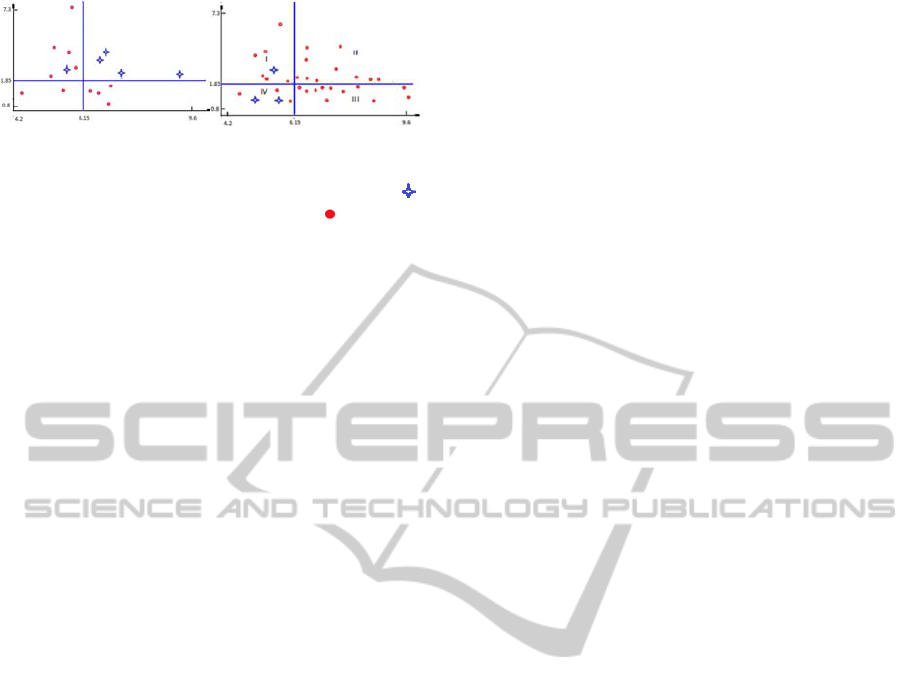
Figure 1: At the left part of figure regularity for Axis X
corresponds containment of cholesterol in blood
(mmmol/l), Y- containment of thyrocsyn (mmol/l) -
case with calculated third stage of severity,
- case with
calculated first stage of severity.
At the left side of figure regularity is represented
that ties calculated DE severity and two
abovementioned explanatory variables in group
dd
S
and at the right side
id
S
group empirical distribution
is represented for the same pair of explanatory
variables. It is seen that quadrant II at left part of
figure contains 4 cases with calculated third severity
stage and the same quadrant II at right part of figure
contains 10 cases with calculated first severity stage.
Statistical validity of difference between
distributions represented al left and right parts of
figure was evaluated at p<0.01 with the help of
permutation test using functional
Q
F
Δ
.
5 CONCLUSIONS
Thus new techniques were represented that are
aimed at improving performance of OVP method.
The represented methods allow to asses structure of
regularities systems in high-dimensional tasks and to
estimate contribution of each single variable. Also
variant of OVP method was discussed that allows to
compare effects of explanatory variables on outcome
in different groups of objects. An example
concerning using of this technique in clinical and
genetic researches was considered. The represented
methods may be used in various data analysis tasks.
ACKNOWLEDGEMENTS
The research was supported by Russian Fond of
Basic Researches grant 11-07-0715. We thanks Irina
Kostomarova and Natalia Malygina for biomedical
problems statement and useful discussions.
REFERENCES
Abdolell, M., LeBlanc, M., Stephens, D., Harrison, R. V.,
2002. Binary partitioning for continuous longitudinal
data: categorizing a prognostic variable. In Statistics in
Medicine, 21:3395-3409.
Breiman, L., Friedman, J. H., Olshen, R. A. and Stone, C.
J., 1984. Classification and Regression Trees,
Chapman & Hall, New York
Dedovets M, Senko O., 2010. The Algorithm Based on
Metric Regularities. In International Journal
“Information Theories and Applications”, Vol. 17,
Number 1, 27-29.
Ernst, M. J., 2004. Permutation methods: A basis for exact
inference. In Statistical Science, 19: 676-685.
Kim, H., Loh, W. Y., 2003. Classification Trees with
Bivariate Linear Discriminant Node Models, In
Journal of Computational and Graphical Statistics,
12: 512–530.
Kostomarova, I., Kuznetsova, A., Malygina, N., Senko,
O., 2010. Methods for evaluating of regularities
systems structure. In New Trends in Classification and
Data Mining, ITHEA, Sofia, Bulgaria, 40-46.
Kostomarova, I., Kuznetsova, A., Malygina, N., Senko,
O., 2011. Method for evaluating discrepancy between
regularities systems in different groups. In
International Journal "Information Technologies &
Knowledge" Vol.5, Number 1, 46-53
Kovshov, V. V., Moiseev, V. L., Ryazanov, V. V., 2008.
Algorithms for finding Logical Regularities in Pattern
Recognition. In Computational mathematics and
Mathematical Physics, 48: 314-328.
Ryazanov, V. V., 2007. Logical Regularities in Pattern
Recognition (parametric approach). In Computational
mathematics and Mathematical Physics, 47: 1793-
1808.
Sen’ko, O. V., Kuznetsova, A. V., 1998. The use of
partitions constructions for stochastic dependencies
approximation. In Proceedings of the International
conference on systems and signals in intelligent
technologies. Minsk (Belarus), 291-297.
Sen’ko, O. V., Kuznetsova, A. V., 2006. The Optimal
Valid Partitioning Procedures. In Statistics on the
Internet http://statjournals.net/
Senko, O. V., Kuznetsova A. V. 2009. Methods of
Regularities Searching Based on Optimal Partitioning.
In International Book Series “Information Science and
Computing”, N 8, Classification, Forecasting, Data
Mining, ITHEA, Sofia, 136-141
KDIR 2011 - International Conference on Knowledge Discovery and Information Retrieval
426