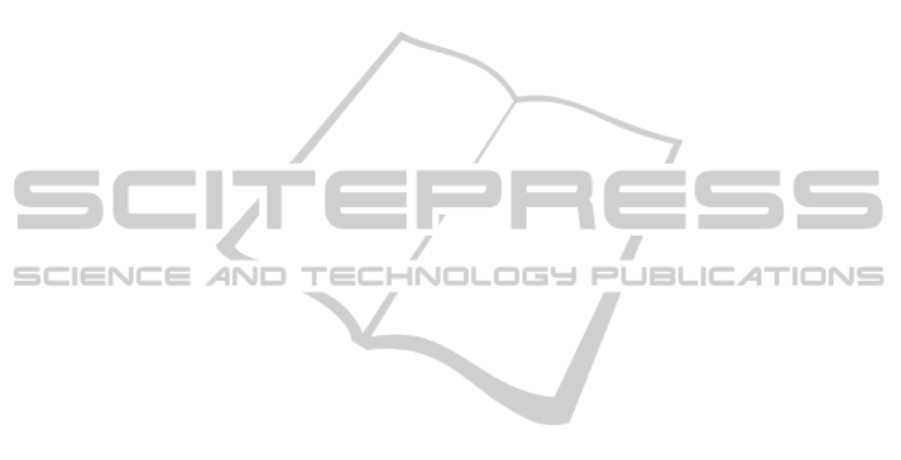
uations, it is likely that null is the most co-occurring
concept and the other concepts because they are too
much scattered are not associated to enough topics.
So they appear in the utterance but not on enough
words to be retained by the segmentation process.
From the high-level to fine-level concept evalua-
tion, results globally decrease of 10%. A loss of 12%
is observed from the generation to the alignment eval-
uation. In the fine-level evaluation, a maximum F-
measure of 52.5% is observed for the generation of
75 topics (Figure 1), corresponding to 54.9% in pre-
cision and 50.3% in recall whereas the F-measure de-
creases to 41% (precision=46.7% and recall=36.7%)
in the alignment evaluation (Figure 2).
To conclude on the [LDA] system, we can see that
it generates topics having a good correlation with the
high-level concepts, seemingly the best representation
level between topics and concepts. It is obvious that
an additional step is needed to obtain a more accurate
segmental annotation, what is expected with the use
of ILP.
[LDA + ILP] performs better whatever the level of
evaluation. For instance, an F-measure of 66% is ob-
served considering the high-level concept generation
for 75 topics (Figure 2). As for [LDA], the same losses
are observed between high-level and fine-level con-
cepts and generation and alignment paradigms. Nev-
ertheless, an F-measure of 54.8% is observed at the
high-level concept in alignment evaluation (Figure 2)
that corresponds to a precision of 56.2% and a recall
of 53.5%, which is not so low considering a fully-
automatic high-level annotation system.
4 CONCLUSIONS
In this paper an approach has been presented for con-
cept discovery and segmental semantic annotation of
user’s turns in an information-query dialogue system.
An evaluation based on an automatic association be-
tween generated topics and expected concepts has
been shown that topics induced by LDA are close to
high-level task-dependent concepts. The segmental
annotation process increases performance both for the
generation and alignment evaluations. On the whole
these results confirm the applicability of the technique
to practical tasks with expected gain in data produc-
tion.
Future work will investigate the use of n-grams to
extend LDA and to increase its accuracy for provid-
ing better hypotheses to the following segmentation
techniques. Also another technique for automatic re-
alignment, based on IBM models used in stochastic
machine translation, will be examined.
REFERENCES
Blei, D., Ng, A., and Jordan, M. (2003). Latent Dirichlet al-
location. The Journal of Machine Learning Research,
3:993–1022.
Bonneau-Maynard, H., Rosset, S., Ayache, C., Kuhn, A.,
and Mostefa, D. (2005). Semantic annotation of the
French MEDIA dialog corpus. In Proceedings of Eu-
rospeech.
Celikyilmaz, A., Hakkani-Tur, D., and Tur, G. (2010). LDA
based similarity modeling for question answering. In
Proceedings of the NAACL HLT 2010 Workshop on
Semantic Search, pages 1–9. Association for Compu-
tational Linguistics.
Chen, D., Batson, R. G., and Dang, Y. (2010). Applied
Integer Programming: Modeling and Solution. Wiley.
Iosif, E., Tegos, A., Pangos, A., Fosler-Lussier, E., and
Potamianos, A. (2006). Unsupervised combination of
metrics for semantic class induction. In Proceedings
of the IEEE Spoken Language Technology Workshop,
pages 86–89.
Pargellis, A., Fosler-Lussier, E., Potamianos, A., and Lee,
C. (2001). Metrics for measuring domain indepen-
dence of semantic classes. In Proceedings of Eu-
rospeech.
Phan, X., Nguyen, L., and Horiguchi, S. (2008). Learning
to classify short and sparse text & web with hidden
topics from large-scale data collections. In Proceeding
of the 17th international conference on World Wide
Web, pages 91–100. ACM.
ReVelle, C. S. and Eiselt, H. A. (2005). Location analysis:
A synthesis and survey. European Journal of Opera-
tional Research, 165(1):1–19.
Siu, K. and Meng, H. (1999). Semi-automatic acquisition of
domain-specific semantic structures. In Proceedings
of Eurospeech.
Tam, Y. and Schultz, T. (2006). Unsupervised language
model adaptation using latent semantic marginals. In
Proceedings of Interspeech, pages 2206–2209.
CONCEPT DISCOVERY FOR LANGUAGE UNDERSTANDING IN AN INFORMATION-QUERY DIALOGUE
SYSTEM
29