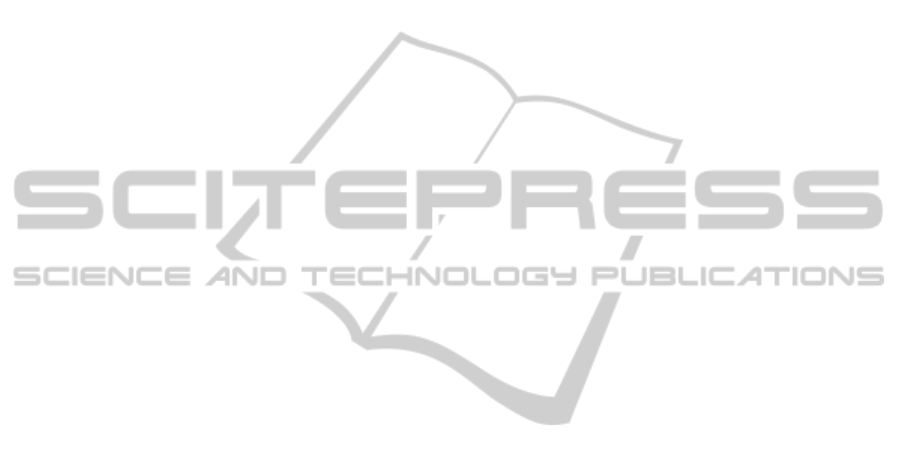
As can be seen on Figure 1, fuzzy system and
neural network have a full structure mapping.
,…,
are species related to input fuzzy variables,
where one species have a chromosome that contains
all nodes (i.e. membership functions parameters)
related to one input fuzzy variable.
,…,
are
species related to fuzzy rules. Antecedent parts of
rules with the same consequent part are encoded in
the same chromosome. Since the number of different
consequent parts is equal to the number of
membership functions in output fuzzy variable,
number of species related to fuzzy rules is equal to
the number of membership functions of the output
fuzzy variable. Chromosome for the species
is composed of nodes (i.e. membership functions
parameters) of the output fuzzy variable. The last of
species –
is related to active/inactive rules in
the model. This species is not marked on Figure 1
because it cannot be done in adequate way.
Chromosome size in each of species is variable
during the evolution of that species, because the
number of membership functions per fuzzy variable
and number of rules are changed dynamically.
From the rough description of the coevolving
species is possible to see that structure of the fuzzy-
neural system is split to coevolving species based on
logical interrelationships of elements. This is very
important for the coevolution efficiency, which is
shown in the tests results.
2.1 General Characteristics of the
Hybridisation
Hybridisation presented on Figure 1. uses only
triangular fuzzy sets. Fuzzy system is of Mamdani
type. Defuzzification type is centroid. The neural
network is five-layered with a clear mapping of the
fuzzy system into the neural network. The specific
backpropagation, not explained in details here, used
in this hybridisation can perform small adaptations
of the fuzzy-neural system and can be used after
system is built. Although the main optimization
mechanism is coevolutionary algorithm,
backpropagation is used partially during system
building. These two mechanisms interleave during
system’s model optimization as global and local
mechanism.
These characteristics are chosen to support
following goals:
Fuzzy presentation of knowledge learned, that
enables its easier interpretability;
Smaller size of the resulting fuzzy system’s model
comparing to Sugeno since “the number of the input
fuzzy sets and fuzzy rules needed by the Sugeno
fuzzy systems depend on the number and locations
of the extrema of the function to be approximated”
(Sivanandam et all, 2007);
The resulting system can be used as fuzzy system
with all its functionalities or as neural network with
all its functionalities including the backpropagation.
2.2 Coevolution Characteristics
From the previous explanation of the species that are
in coevolution it is obvious that complexity of the
optimization problem is high. Besides that,
implementation this hybridisation generates the
fuzzy-neural system from data automatically, which
additionally makes the problem more difficult. But
this coevolution organization has species with
relatively small chromosomes comparing to similar
cases where less species is used for fuzzy system’s
model optimization.
Each optimization algorithm has its mechanisms
to skip local optimums and search for better
solution. In the case of this hybridization, the
coevolutionary algorithm is implemented with
following mechanisms and with following
behaviours:
Coevolution of species. Coevolution of species is
implemented in the classical manner where each of
species has its own evolution up to the fitness
calculation. For fitness calculation, target member
and chosen cooperators from the rest of the species
are joined to form fuzzy-neural system. That fuzzy-
neural system (as neural network) is shortly trained
via the specific backpropagation to increase the
quality of the model and to measure its performance
with the training dataset. Behaviour of the
coevolution of species mainly depends on input
parameters, but also on additional mechanisms:
In the case of the very weak progress mechanism
of random generation of members is used along with
the basic mechanisms – crossover and mutation.
In the case of the weak progress, some of parameters
of the coevolution are change dynamically.
Coevolution of two groups of species. This type of
coevolution is based on the analogy with the
coevolution of two species in coevolutionary fuzzy
modelling (Pena-Reyes, 2002). While in
coevolutionary fuzzy modelling there are only two
species – one related to fuzzy variables and one
related to fuzzy rules, in the coevolution of two
groups of species one of groups contains species
related to fuzzy variables while the other one contain
species related to fuzzy rules.
Except the analogy, the rest of behaviour is
different. Figure 2 shows how it works. At the start
OPTIMIZATION OF STRUCTURE OF FUZZY-NEURAL SYSTEMS USING COEVOLUTIONARY ALGORITHM
127