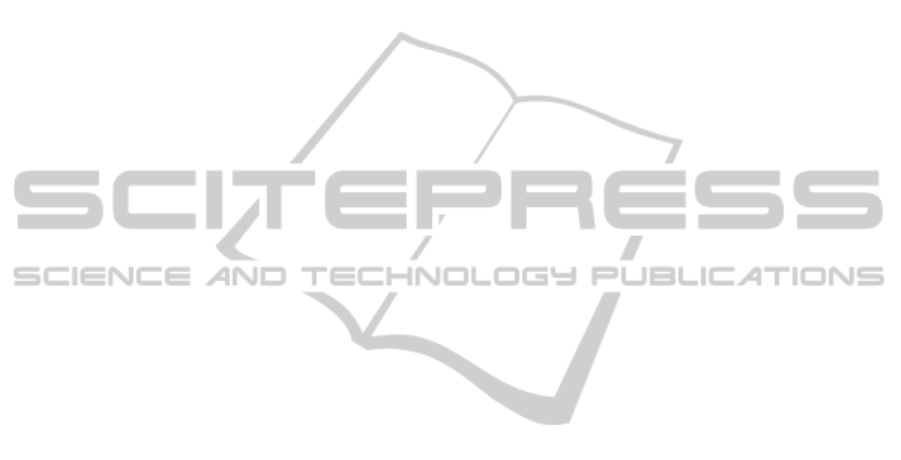
tivation for continuing its exploration stands in the
search of a ground state which takes into account the
agent mobility as a further facility of the neurons. Ac-
tually, artificial neural networks paradigm borns ex-
actly in view of emulating an analogous paradigm
which proved to be very efficient in nature. Nowadays
we may envisage in social networks an extension of
this paradigm as a social phenomenon which roots a
great part of the ethological system evolution (Easley
and Kleinberg, 2010). In turn, this may be consid-
ered a macro-scale companion of the neuromorphol-
ogy process ruling the early stage of our live. Thus,
we try to transfer one of the main features of both phe-
nomena, namely the agents’ mobility (either actual or
virtual within the web) as a complement to the infor-
mation piping capability of the network connecting
them.
There is a lot of issues related to the task of com-
bining motion with cognitive phenomena. On the one
hand, in a very ambitious perspective we could con-
sider learning as another form of mobility in a proper
subspace, so as to state a link in terms of potential
fields of the same order scientists stated in the past
between thermodynamic and informative entropy. On
the other hand, we may draw from the granular com-
puting province (Apolloni et al., 2008) the analogous
of the Boltzmann constant (Fermi, 1956) in terms of
links between physical and cognitive aspects. In this
paper, besides the notion of neuron cognitive masses,
we stated this link through the λ coefficients. In turn,
they play a clear role of membership function of the
downward-layer neurons to the cognitive basin of the
upward-layer neurons. We will further elaborate on
these aspects in a future work.
REFERENCES
Apolloni, B., Bassis, S., and Brega, A. (2009). Feature se-
lection via Boolean Independent Component analysis.
Information Science, 179(22):3815–3831.
Apolloni, B., Bassis, S., Malchiodi, D., and Pedrycz, W.
(2008). The Puzzle of Granular Computing, volume
138. Springer Verlag.
Carpenter, G. A. and Grossberg, S. (2003). Adaptive reso-
nance theory. In The Handbook of Brain Theory and
Neural Networks, pages 87–90. MIT Press.
Ciresan, D. C., Meier, U., Gambardella, L. M., and Schmid-
huber, J. (2010). Deep big simple neural nets for
handwritten digit recognition. Neural Computation,
22(12):3207–3220.
Corke, P. I. (1996). A robotics toolbox for Matlab. IEEE
Rob. and Aut. Mag., 3(1):24–32.
Danafar, S., Gretton, A., and Schmidhuber, J. (2010).
Characteristic kernels on structured domains excel in
robotics and human action recognition. In Machine
Learning and Knowledge Discovery in Databases,
volume 6321, pages 264–279. Springer, Berlin.
Dirac, P. A. M. (1982). The Principles of Quantum Mechan-
ics. Oxford University Press, USA.
Easley, D. and Kleinberg, J. (2010). Networks, Crowds,
and Markets: Reasoning About a Highly Connected
World. Cambridge University Press, Cambridge, MA.
Ezhov, A. and Ventura, D. (2000). Quantum neural net-
works. Future Directions for Intelligent Systems and
Information Science 2000.
Fermi, E. (1956). Thermodynamics. Dover Publications.
Feynman, R., Leighton, R., and Sands, M. (1963). The
Feynman Lectures on Physics, volume 3. Addison-
Wesley, Boston.
Hinton, G. E., Osindero, S., and Teh, Y. W. (2006). A fast
learning algorithm for deep belief nets. Neural Com-
putation, 18:1527–1554.
Kreutz-Delgado, K. and Rao, B. D. (1998). Application
of concave/Schur-concave functions to the learning of
overcomplete dictionaries and sparse representations.
In 32th Asilomar Conference on Signals, Systems &
Computers, volume 1, pages 546–550.
Larochelle, H., Bengio, Y., Louradour, J., and Lamblin, P.
(2009). Exploring strategies for training deep neural
networks. Jour. Machine Learning Research, 10:1–40.
LeCun, Y. (1988). A theoretical framework for back-
propagation. In Proc. of the 1988 Connectionist Mod-
els Summer School, pages 21–28. Morgan Kaufmann.
LeCun, Y., Bottou, L., Bengio, Y., and Haffner, P. (1998).
Gradient-based learning applied to document recogni-
tion. Proceedings of the IEEE, 86(11):2278–2324.
Mar´ın, O. and Lopez-Bendito, G. (2007). Neuronal migra-
tion. In Evolution of Nervous Systems: a Comprehen-
sive Reference, chapter 1.1. Academic Press.
Mar´ın, O. and Rubenstein, J. L. (2003). Cell migration in
the forebrain. Review in Neurosciences, 26:441–483.
NVIDIA Corporation (2010). Nvidia Tesla c2050 and
c2070 computing processors.
Rasmussen, C. E., Neal, R. M., Hinton, G. E., van Camp,
D., Revow, M., Ghaharamani, Z., Kustra, R., and
Tibshirani, R. (1996). The Delve manual. Techni-
cal report, Dept. Computer Science, Univ. of Toronto,
Canada. Ver. 1.1.
Stanley, K. O. and Miikkulainen, R. (2002). Evolving neu-
ral networks through augmenting topologies. Evolu-
tionary Computation, 10(2):99–127.
KINETIC MORPHOGENESIS OF A MULTILAYER PERCEPTRON
105