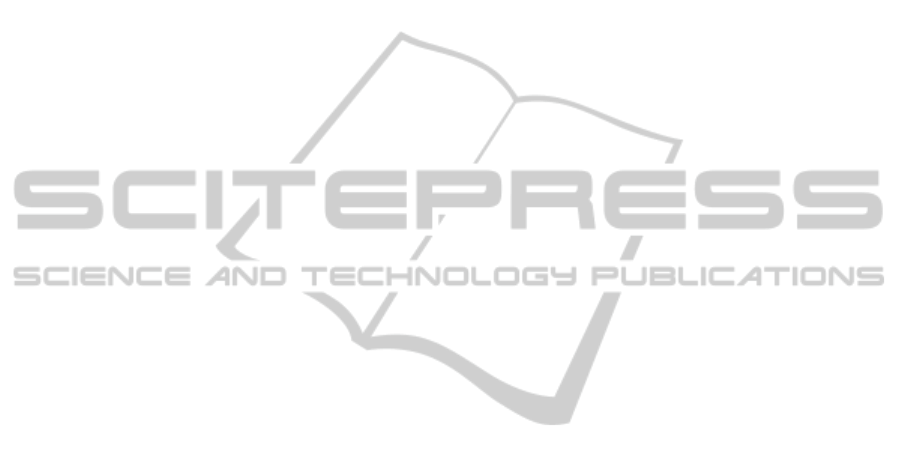
Foundation (DFG). We also acknowledge the DFG
for financing our computing cluster used for parts of
this work.
REFERENCES
Albornoz, E. M., Milone, D. H., and Rufiner, H. L. (2011).
Spoken emotion recognition using hierarchical clas-
sifiers. Computer Speech and Language, 25(3):556–
570.
Baum, L., Petrie, T., Soules, G., and Weiss, N. (1970).
A maximization technique occurring in the statistical
analysis of probabilistic functions of markov chains.
Ann. Math. Stat., 41:164–171.
Bengio, Y., Simard, P., and Frasconi, P. (1994). Learning
long-term dependencies with gradient descent is diffi-
cult. IEEE transactions on neural networks, 5(2):157–
66.
B¨ock, R., H¨ubner, D., and Wendemuth, A. (2010). De-
termining optimal signal features and parameters for
hmm-based emotion classification. In MELECON
2010 - 15th IEEE Mediterranean Electrotechnical
Conference, pages 1586–1590.
Boreczky, J. S. and Wilcox, L. D. (1998). Hidden markov
model framework for video segmentation using audio
and image features. In ICASSP, IEEE International
Conference on Acoustics, Speech and Signal Process-
ing, volume 6, pages 3741–3744.
Burkhardt, F., Paeschke, A., Rolfes, M., Sendlmeier, W.,
and Weiss, B. (2005). A database of german emo-
tional speech. In Proceedings of the 9th European
Conference on Speech Communication and Technol-
ogy; Lisbon, pages 1517–1520.
Chen, J. and Chaudhari, N. (2009). Segmented-memory
recurrent neural networks. Neural Networks, IEEE
Transactions, 20(8):1267–80.
Ekman, P. (July 1992). Are there basic emotions? Psycho-
logical Review, 99:550–553.
El Ayadi, M., Kamel, M., and Karray, F. (2007). Speech
emotion recognition using gaussian mixture vector au-
toregressive models. In Acoustics, Speech and Signal
Processing, 2007. ICASSP 2007. IEEE International
Conference on, volume 4, pages 957–960. IEEE.
El Ayadi, M., Kamel, M. S., and Karray, F. (2011). Sur-
vey on speech emotion recognition: Features, classi-
fication schemes, and databases. Pattern Recognition,
44(3):572–587.
Elman, J. L. (1990). Finding structure in time. Cognitive
Science, 14(2):179–211.
Fant, G. (1960). Acoustic theory of speech production.
Mounton, The Hague.
Ganchev, T., Fakotakis, N., and Kokkinakis, G. (2005).
Comparative evaluation of various mfcc implementa-
tions on the speaker verification task. In Proc. of the
SPECOM, pages 191–194.
Gl¨uge, S., B¨ock, R., and Wendemuth, A. (2010a). Im-
plicit sequence learning - a case study with a 4-2-4
encoder simple recurrent network. In Proceedings of
the International Conference on Fuzzy Computation
and 2nd International Conference on Neural Compu-
tation, pages 279–288.
Gl¨uge, S., Hamid, O. H., and Wendemuth, A. (2010b). A
simple recurrent network for implicit learning of tem-
poral sequences. Cognitive Computation, 2(4):265–
271.
Grimm, M., Kroschel, K., Mower, E., and Narayanan, S.
(2007). Primitives-based evaluation and estimation of
emotions in speech. Speech Communication, 49(10-
11):787–800.
Hermansky, H. (1990). Perceptual linear predictive (PLP)
analysis of speech. Journal of the Acoustical Society
of America, 87(4):1738–1752.
Hitch, G. J., Burgess, N., Towse, J. N., and Culpin, V.
(1996). Temporal grouping effects in immediate re-
call: A working memory analysis. Quarterly Journal
of Experimental Psychology Section A: Human Exper-
imental Psychology, 49(1):116–139.
Hochreiter, S. (1998). The vanishing gradient problem dur-
ing learning recurrent neural nets and problem solu-
tions. International Journal of Uncertainty, Fuzziness
and Knowledge-Based Systems, 6(2):107–116.
H¨ubner, D., Vlasenko, B., Grosser, T., and Wendemuth,
A. (2010). Determining optimal features for emo-
tion recognition from speech by applying an evolu-
tionary algorithm. In INTERSPEECH 2010, pages
2358–2361.
Inoue, T., Nakagawa, R., Kondou, M., Koga, T., and Shi-
nohara, K. (2011). Discrimination between mothers’
infant- and adult-directed speech using hidden markov
models. Neuroscience Research, 70(1):62–70.
Kim, W. and Hansen, J. (2010). Angry emotion detec-
tion from real-life conversational speech by leverag-
ing content structure. In Acoustics Speech and Signal
Processing (ICASSP), 2010 IEEE International Con-
ference on, pages 5166–5169.
Mehrabian, A. (1996). Pleasure-arousal-dominance: A gen-
eral framework for describing and measuring individ-
ual differences in temperament. Current Psychology,
14(4):261–292.
M¨uller, M. (2007). Information Retrieval for Music and
Motion. Springer Verlag.
Nicholson, J., Takahashi, K., and Nakatsu, R. (1999). Emo-
tion recognition in speech using neural networks. In
Neural Information Processing, 1999. Proceedings.
ICONIP ’99. 6th International Conference on, vol-
ume 2, pages 495–501.
Nwe, T. L., Foo, S. W., and Silva, L. C. D. (2003). Speech
emotion recognition using hidden markov models.
Speech Communication, 41(4):603–623.
Petrushin, V. A. (2000). Emotion recognition in speech
signal: experimental study, development, and appli-
cation. In Proceedings of the ICSLP 2000, volume 2,
pages 222–225.
Pierre-Yves, O. (2003). The production and recognition of
emotions in speech: features and algorithms. Inter-
national Journal of Human-Computer Studies, 59(1-
2):157 – 183. Applications of Affective Computing in
Human-Computer Interaction.
Rabiner, L. and Juang, B.-H. (1993). Fundamentals of
NCTA 2011 - International Conference on Neural Computation Theory and Applications
314