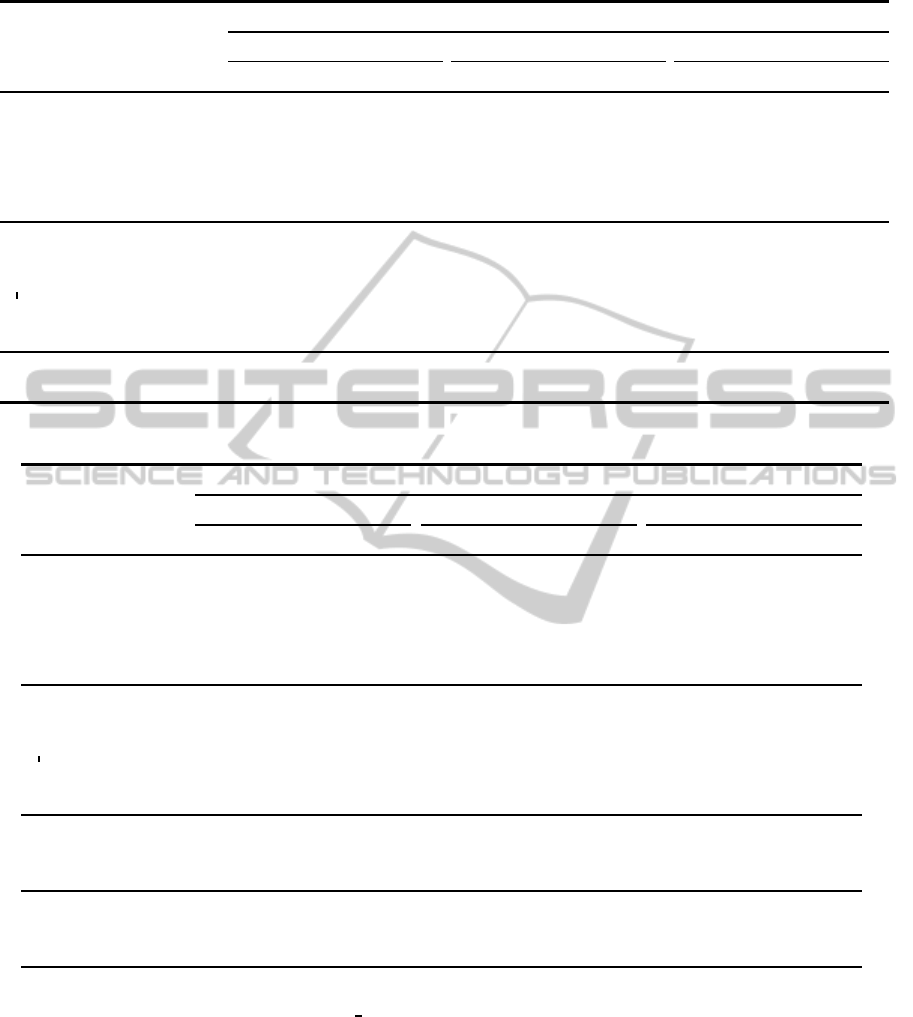
Table 2: Comparative results for datasets of high performance athletics.
Dataset
100ml-4-I 100ml-4-P Long-4
Technique Train Test Train Test Train Test
EOFP
Fuzzy partition
FFR
SM1
[0.107,0.305] [0.130,0.323] [0.043,0.235] [0.093,0.290] [0.191,0.484] [0.083,0.349]
FRF
SM2
[0.110,0.306] [0.150,0.343] [0.045,0.237] [0.110,0.307] [0.165,0.449] [0.083,0.349]
FRF
MWL1
[0.070,0.265] [0.073,0.267] [0.032,0.224] [0.060,0.257] [0.085,0.364] [0.033,0.299]
FRF
MWL2
[0.060,0.254] [0.113,0.306] [0.043,0.235] [0.060,0.257] [0.111,0.391] [0.083,0.349]
FRF
MWLT1
[0.070,0.267] [0.073,0.267] [0.032,0.224] [0.060,0.257] [0.085,0.364] [0.033,0.299]
FRF
MWLT2
[0.060,0.252] [0.093,0.286] [0.038,0.231] [0.060,0.257] [0.107,0.386] [0,083,0.349]
OFP CLASS
Fuzzy partition
FFR
SM1
[0.139,0.331] [0.150,0.343] [0.098,0.291] [0.133,0.310] [0.120,0.404] [0.200,0.467]
FRF
SM2
[0.141,0.333] [0.150,0.343] [0.096,0.288] [0.093,0.290] [0.115,0.391] [0.200,0.467]
FRF
MWL1
[0.077,0.269] [0.093,0.287] [0.075,0.269] [0.073,0.270] [0.116,0.396] [0.100,0.417]
FRF
MWL2
[0.060,0.252] [0.093,0.287] [0.077,0.269] [0.073,0.270] [0.102,0.382] [0.100,0.367]
FRF
MWLT1
[0.077,0.269] [0.093,0.287] [0.075,0.267] [0.073,0.270] [0.107,0.387] [0.150,0.417]
FRF
MWLT2
[0.062,0.254] [0.093,0.287] [0.077,0.269] [0.073,0.270] [0.094,0.373] [0.067,0.333]
Crisp (Palacios et al., 2009) 0.259 0.384 0.288 0.419 0.327 0.544
GGFS (Palacios et al., 2009) [0.089,0.346] [0.189,0.476] [0.076,0.320] [0.170,0.406] [0.000,0.279] [0.349,0.616]
Table 3: Comparative results for datasets of dyslexia.
Dataset
Dyslexic-12 Dyslexic-12-01 Dyslexic-12-12
Technique Train Test Train Test Train Test
EOFP
Fuzzy partition
FRF
SM1
[0.000,0.238] [0.000,0.398] [0.022,0.223] [0.039,0.377] [0.001,0.263] [0.035,0.422]
FRF
SM2
[0.000,0.228] [0.000,0.399] [0.008,0.184] [0.022,0.332] [0.009,0.245] [0.032,0.411]
FRF
MWL1
[0.000,0.270] [0.000,0.406] [0.017,0.231] [0.045,0.383] [0.001,0.273] [0.019,0.430]
FRF
MWL2
[0.000,0.270] [0.000,0.407] [0.020,0.241] [0.056,0.385] [0.001,0.267] [0.026,0.406]
FRF
MWLT1
[0.000,0.263] [0.000,0.402] [0.012,0.216] [0.038,0.365] [0.000,0.265] [0.019,0.427]
FRF
MWLT2
[0.000,0.266] [0.000,0.404] [0.015,0.221] [0.049,0.373] [0.000,0.262] [0.024,0.422]
OFP CLASS
Fuzzy partition
FRF
SM1
[0.000,0.320] [0.002,0.511] [0.000,0.282] [0.000,0.413] [0.000,0.405] [0.000,0.477]
FRF
SM2
[0.000,0.327] [0.001,0.515] [0.000,0.253] [0.000,0.389] [0.000,0.402] [0.000,0.469]
FRF
MWL1
[0.000,0.261] [0.003,0.419] [0.000,0.264] [0.000,0.400] [0.000,0.335] [0.000,0.422]
FRF
MWL2
[0.000,0.270] [0.003,0.423] [0.000,0.276] [0.000,0.407] [0.000,0.343] [0.000,0.414]
FRF
MWLT1
[0.000,0.264] [0.004,0.419] [0.000,0.243] [0.000,0.386] [0.000,0.331] [0.000,0.422]
FRF
MWLT2
[0.000,0.267] [0.003,0.417] [0.000,0.259] [0.000,0.394] [0.000,0.343] [0.000,0.418]
Crisp CF
0
(*) 0.444 [0.572,0.694] 0.336 [0.452,0.533] 0.390 [0.511,0.664]
GGFS (*) – [0.421,0.558] – [0.219,0.759] – [0.199,0.757]
GGFS CF
0
(*) [0.003,0.237] [0.405,0.548] [0.005,0.193] [0.330,0.440] [0.003,0.243] [0.325,0.509]
Crisp CF
0
(**) 0.556 [0.614,0.731] 0.460 [0.508,0.605] 0.485 [0.539,0.692]
GGFS (**) – [0.490,0.609] – [0.323,0.797] – [0.211,0.700]
GGFS CF
0
(**) [0.038,0.233] [0.480,0.621] [0.000,0.187] [0.394,0.522] [0.000,0.239] [0.393,0.591]
better results in test than FRF with OFP CLASS
fuzzy partitions. FRF ensemble is a significant im-
provement over the crisp GFS. In these experiments
we can see that when the partitions are obtained with
the original data using the EOFP algorithm, the ac-
curacy is higher (the intervals of error are closer to
0 and they are less imprecise). As also discussed in
(Palacios et al., 2010) is preferable to use an algo-
rithm which is capable of learning with low quality
data than removing the imperfect information and us-
ing a conventional algorithm.
4 CONCLUSIONS
In this paper we have presented the EOFP Algorithm
for fuzzy discretization of continuous attributes. This
algorithm is able to work with imperfect information.
CONSTRUCTING FUZZY PARTITIONS FROM IMPRECISE DATA
387