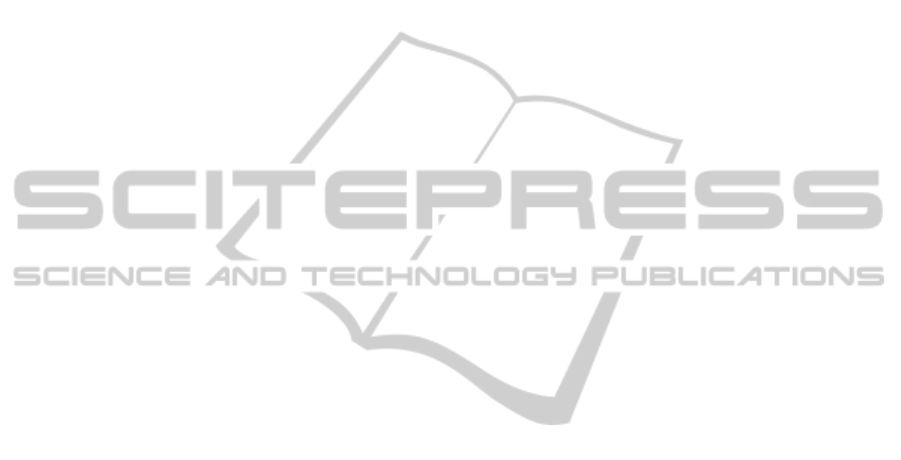
These modifications permit the Viterbi algorithm
to circumvent the problems of impossible
transitions, or emissions due to insufficient data in
the training sequences that resulted in nul
probabilities in the estimated parameters.
4 APPLICATION FOR THE
RESTITUTION OF THE
VERTICAL CHLOROPHYLL-
A CONCENTRATION
THROUGH SEA SURFACE
DATA
The bio-geochemical activity of the oceans and the
carbon cycle are two parts of a complex feedback
system. A change in climate and an increase of the
amount of available carbon can affect the primary
oceanic production, and in return a change in the
bio-geochemical activity affects, by modifying the
albedo and carbon fixation rates, the climate and
carbon concentration. It is therefore important to be
able to determine the oceanic primary production. In
recent years, many algorithms have been developed
that infer the Chlorophyll-A concentration in ocean
surface layers through satellite imaging (Brajard et
al., 2008). It has also been proved that the vertical
Chlorophyll-A distribution, is correlated with sea
surface data (Uitz et al., 2006). Therefore, the
determination of the vertical distribution of
Chlorophyll-A from sea surface data is a problem
that can be solved by the methodology we propose.
One cannot determine the vertical distribution of
Chlorophyll-A without first understanding the
parameters that influence the development of
phytoplankton. It is generally accepted that
phytoplankton growth depends on 5 parameters:
available radiation, available nutriments, predators
and biology, water temperature, water turbidity.
These parameters cannot easily be monitored
through a direct approach. Satellite imaging,
however, can give us proxy information, which can
be used in an empirical approach for determining the
vertical distribution of Chlorophyll-A. Specifically,
in this study we used: Sea Surface Chlorophyll-A
concentration (SCHL), Sea Surface Temperature
(SST), Sea Surface Elevation (SSH), Shortwave
Radiation (SR) and Wind-speed Intensity (WS).
Since our objective is to validate the theoretical
methodology, we used simulated data in order to test
the validity of our approach. We therefore
approximated the satellite values of the previous
parameters by using the input and output values
provided by the NEMO oceanic circulation model
coupled to the PISCES bio-geochemical model (C.
Moulin, 2008). In order to better simulate the noise
and errors inherent to satellite images we added a
white noise z ~ N(0,ê), ê=1/2 * (σ
schl
, σ
sst
, σ
ssh
, σ
ws
,
σ
sr
) to the parameters that could be gathered from
satellite imaging. σ represents the standard
derivation of each corresponding surface parameter,
as computed on the training data. The application
was set at the site of BATS.
The unobserved states that were classified, were
the output data vectors containing the average
vertical Chlorophyll-A distribution at 17 depth
levels (from 5 meters to 217) and temperature
distribution at 9 depth levels. These vertical
distribution profiles were 5-day averages spanning
the period from 1991 to 2007 located in a 2°x2°
square centred on BATS. Therefore M
dis
belongs to
R
26
(17 levels of Chlorophyll-A + 9 levels of
Temperature). M
obs
belongs to R
5
.
We trained M
obs
and M
dis
by taking into account
all available profiles at BATS, as well as any
adjacent points included in the model. This gave us
9*73*17=11169 profiles for the construction of the
maps. The optimum map sizes, N
obs
and N
dis
were
determined to be 21*14=294 classes.
For the estimation of the HHM parameters on
the other hand, we take a total of 14 years (1991-
2004) for the training, each including seventy-three
5-day steps. Therefore T
training
=1022. We maintained
3 years (2005-2007), or 219 5-day steps, to validate
our approach.
The results shown in Figure 1 present the
temporary evolution of Chlorophyll-A profiles in ten
5-day steps sequence, from 04-04-2005 to 19-05-
2005, at BATS. In green we see the Chlorophyll-A
distribution profiles, taken from the referents r
dis
of
M
dis
, corresponding to the indexes of the
reconstructed time series S
dis-rec
. In blue we can see
the vertical distribution of Chlorophyll-A according
to the NEMO-PISCES model at the same 5-day
steps.
In Figure 1 we also have, preceded by the
acronym Rec, the indexes that constitute time series
S
dis-rec
and, preceeded by Mod, the indexes we obtain
by projecting the corresponding profiles of the
NEMO-PISCES model on M
dis
. In order to avoid
confusion, the profiles corresponding to the indexes
after Mod are not displayed. When the vertical
distribution of Chlorophyll-A is know, these indexes
would correspond to the optimum reconstruction we
could get with M
dis
. We note this optimum time
series as S
dis-opt
. It is interesting to notice that even
NCTA 2011 - International Conference on Neural Computation Theory and Applications
320