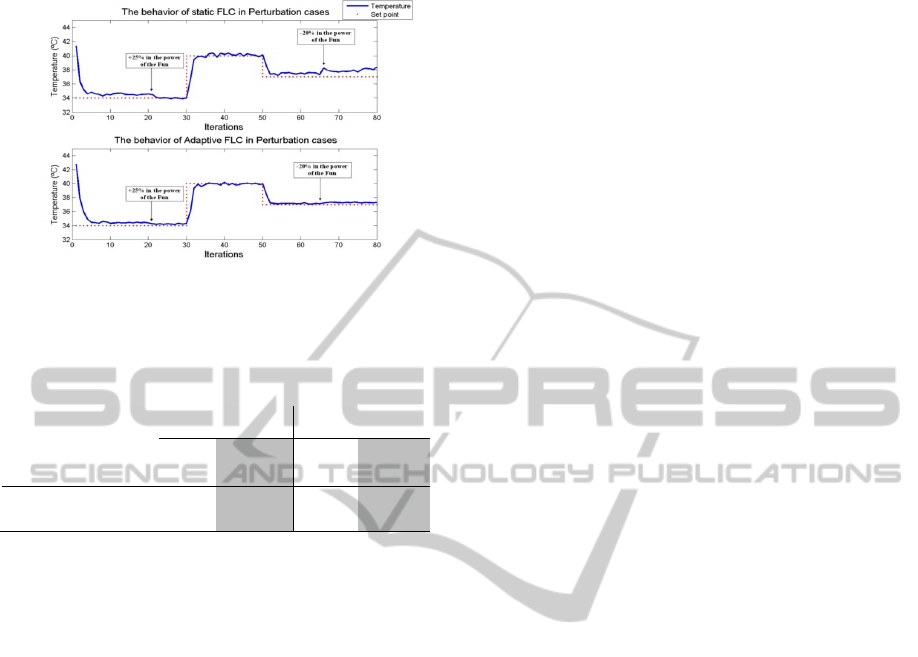
10 iterations with disturbance for each algorithm.
Figure 9: The perturbation effects on the control
performance.
Table 3: Comparative performance indices before and
after the perturbation.
MSE in 10 iterations
+ %25 in the
power of the Fan
- %20 in the power
of the Fan
FLC
Adapt
FLC
FLC
Adapt
FLC
Before perturbation
0.78 0.42 0.64 0.1
After perturbation
0.32 0.36 1.77 0.14
It is important to clear here that the error
reduction in the first case of perturbation doesn’t
mean a control improvement, it’s due to the new Fan
added by simulation, adding a Fan with power more
bigger signify big reduction in the error committed
and maybe the error will change the sign if this Fan
power was too much. The MSE presented in this
table proves that the perturbation effects on the
behavior of the adaptive algorithm are very small
when compared with their effects on the behavior of
the FLC algorithm.
5 CONCLUSIONS
This paper presents a comparative study between
several control algorithms and an adaptive fuzzy
logic controller. Both, the conventional fuzzy logic
controller and the P, I and D controller structure
show their capabilities to control the plant with a
reasonable error. The adaptive algorithm without
any off line pre-training and starting with no definite
rule base has been able to improve the committed
error during the control process. The adaptive
algorithm does not need any complex mathematical
models. It only needs a limited information from the
plant.The monotonicity and the delay of the plant,
were the only information used by the adaptive
algorithm to optimize the error at the plant output.
The results comparison can prove the superiority of
the proposed adaptive FLC controller against the
remaining of algorithms.
ACKNOWLEDGEMENTS
This work has been supported by the Genil Start-up
Project “Processing and Classification of Electro-
oculography (EOG) Data for Ataxia SCA-2
Diagnosis” (PYR-2010-23) from the CEI bioTIC
GENIL (CEB09-0010) of the CEI program from the
MICINN.
REFERENCES
Rojas, I., Pomares, H., González, J., Herrera, L. J., Guillen
,A., Rojas ,F., Valenzuela ,O., 2006. Adaptive fuzzy
controller: Application to the control of the tem-
perature of a dynamic room in real time, Fuzzy Sets
and Systems 157, 2241 – 2258.
Fan, X., Zhang, N., Teng, S., 2004. Trajectory planning
and tracking of ball and plate system using hierar-
chical fuzzy control scheme, Fuzzy Sets and Systems
144 (2) 297–312.
Hua, C., Guan, X., Duan, G., 2004. Variable structure ada-
ptive fuzzy control for a class of nonlinear time-delay
systems, Fuzzy Sets and Systems 148 (3) 453–468.
Lin, C. M., Hsu, C. F., 2004. Adaptive fuzzy sliding-mode
control for induction servomotor systems, IEEE Trans.
Energy Conversion 19 (2) 362–368.
Mamdani, E. H., Assilian, S., 1975. Experiment in
Linguistic Synthesis with a Fuzzy Logic Controller.
International Journal of Man-Machine Studies, 7, 1-
13.
Park, J.-H., Park, G.-T., Kim, S.-H., Moon, C.-J., 2005.
Output-feedback control of uncertain nonlinear
systems using a self-structuring adaptive fuzzy
observer, Fuzzy Sets and Systems 151 21–42.
Velagic, J., Vukic, Z., Omerdic, E., 2003. Adaptive fuzzy
ship autopilot for track-keeping, Control Eng. Practice
11 433–443.
Velez-Diaz, D., Tang, Y., 2004. Adaptive robust fuzzy
control of nonlinear systems, IEEE Trans. Systems,
Man Cybernetics, Part B 34 1596–1601.
Ziegler, J. G., Nichols, N. B., 1942. Optimum Settings for
Automatic Controllers, Trans. ASME, Vol. 64,759-
768.
Yi, J., Yubazaki, N., Hirota, K., 2002. A proposal of
SIRMs dynamically connected fuzzy inference model
for plural input fuzzy control, Fuzzy Sets and Systems
125 (1) 79–92.
Rojas, I., Pomares, H., Pelayo, F. J., Anguita, M., Ros, E.,
Prieto, A., 1999. New methodology for the develop-
pment of adaptive and self-learning fuzzy controllers
in real time, Int. J. Approx. Reason. 21, 109–136.
FCTA 2011 - International Conference on Fuzzy Computation Theory and Applications
474