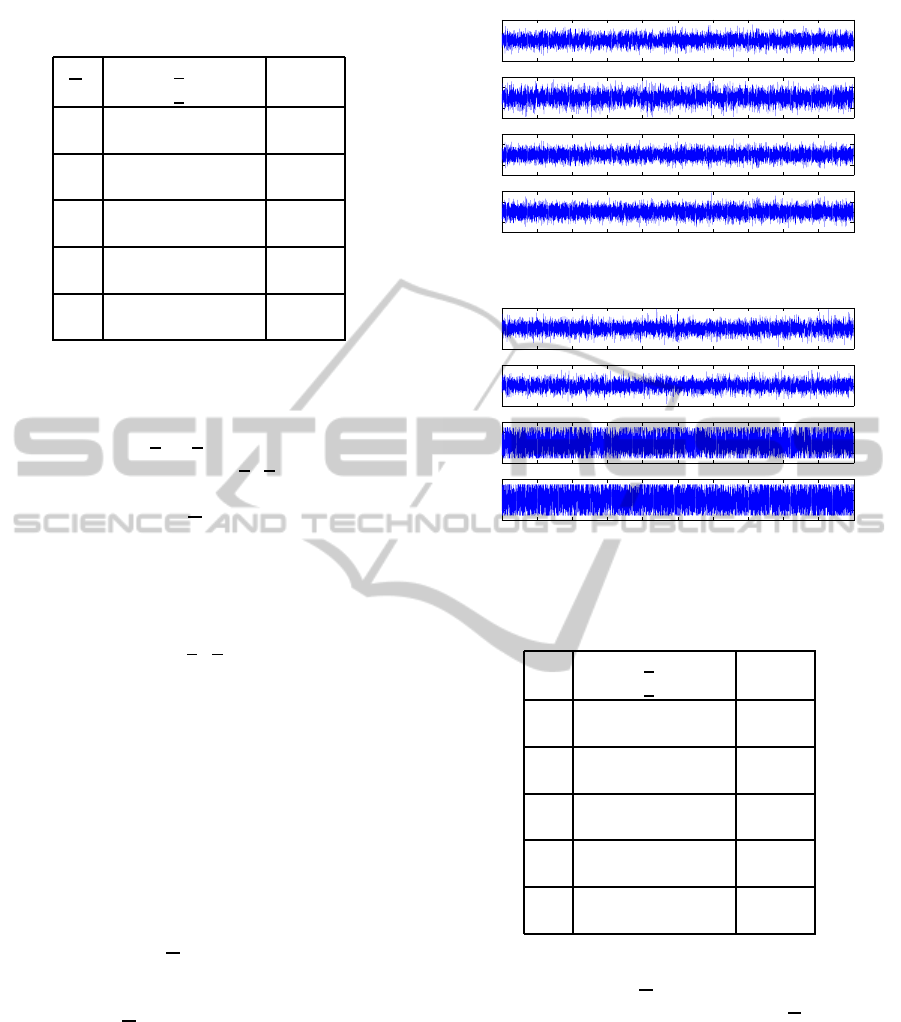
Table 1: Feedback gains and loss function values; failure
(3).
α
i
k
T
1
−
¯
α
i
k
T
2
¯
J
0.9 –0.9004, –0.3319 –
1.1 –0.1572, –1.0898 2.2794
0.7 –0.7492, –0.0924 –
1.3 0.0342, –0.7964 2.4626
0.5 –0.6357, 0.0989 –
1.5 0.1967, –0.5417 2.7634
0.3 –0.6141, 0.1750 –
1.7 0.3034, –0.3381 3.1764
0.1 –0.9880, 0.1943 –
1.9 0.5037, –0.2898 4.6344
Σ
w
=
1 0
0 1
,
Q = I, R = 0.1I and x
0
= 0.
The feedback gains K
T
= (k
1
, k
2
) and the corre-
sponding values of the loss function for few configu-
rations of system failure α
i
,
¯
α
i
are shown in Table 1.
The values of the loss function
¯
J were averaged over
10000 runs.
The feedback gains and the corresponding values
of the loss function for few constraints β
1
, β
2
for fail-
ure (23) are shown in Table 2. In this case, the sub-
optimal feedback gains k
1
, k
2
were calculated with
iterative procedure given in (Toivonen, 1983; Kro-
likowski, 2004) for given constraints β
1
, β
2
.
The optimal value of the loss function (also seen
from Table 2 for β
1
= β
2
= ∞) is J
opt
= 2.256. Fi-
nally, the feedback gains and the corresponding val-
ues of the loss function for failure (24) and different
constraints β
1
, β
2
are shown in Tables 3, 4, 5, 6. It
should be noticed that in this case the amplitude con-
straint β
i
in (24) is realized as a simple cut-off that
is obviously not an optimization approach like in the
previous case.
The exemplary run of inputs and outputs with ac-
tuator failure (3) with α
i
= 0.75,
¯
α
i
= 1.25, i = 1, 2
is shown in Fig.2 where the corresponding loss is
¯
J = 2.4018, and the corresponding run under actuator
failure (24) with α
i
= 0.75,
¯
α
i
= 1.25, β
i
= 1.5, i =
1, 2 is shown in Fig.3 where the corresponding loss is
¯
J = 2.5440.
Analyzing the values of the loss function given
in Tables 3, 4, 5, 6 one can observe a phenomenon
like the short-term behaviour phenomenon described
in (Chen et al., 1993; Chen et al., 1994) which takes
place when the minimum variance control is consid-
ered and the cutoff method is used to constrain the
control signal. This means that even though more
control effort is applied to the system, the closed-loop
system performance does not improve. The effect of
0 1000 2000 3000 4000 5000 6000 7000 8000 9000 10000
−5
0
5
x
1
0 1000 2000 3000 4000 5000 6000 7000 8000 9000 10000
−4
−2
0
2
4
x
2
0 1000 2000 3000 4000 5000 6000 7000 8000 9000 10000
−4
−2
0
2
4
u
1
0 1000 2000 3000 4000 5000 6000 7000 8000 9000 10000
−4
−2
0
2
4
u
2
k
Figure 2: Input and output signals for failure (3).
0 1000 2000 3000 4000 5000 6000 7000 8000 9000 10000
−5
0
5
x
1
(t)
0 1000 2000 3000 4000 5000 6000 7000 8000 9000 10000
−5
0
5
x
2
(t)
0 1000 2000 3000 4000 5000 6000 7000 8000 9000 10000
−2
−1
0
1
2
u
1
(t)
0 1000 2000 3000 4000 5000 6000 7000 8000 9000 10000
−2
−1
0
1
2
u
2
(t)
k
Figure 3: Input and output signals for failure (24).
Table 2: Feedback gains and loss function values; failure
(23).
β
1
k
T
1
−
β
2
k
T
2
¯
J
∞ –0.9322, –0.3821 –
∞ –0.1975, –1.1526 2.2567
1.5 –0.8594, –0.2113 –
1.5 –0.0544, –1.0186 2.4879
1.0 –0.7465, –0.0614 –
1.0 0.1423, –0.8302 2.7932
0.5 –0.5987, 0.1166 –
0.5 0.4141, –0.4282 4.3363
0.3 0.6192, 0.0785 –
0.3 0.5146, –0.2542 9.1401
that kind happens for α
i
= 0.0, 0.1,
¯
α
i
= 2.0, 1.9 as
illustrated in Fig.4, where the notation α
i
= 1 − δ,
¯
α
i
= 1 + δ is used. In Fig.5 where δ = 0.2, 0.3 the
effect is not seen. It can be concluded that the bigger
δ the stronger is the effect.
A similar phenomenon can be observed for given
β
i
and variable δ, for example for β
i
= 1.0, β
i
= 1.5
that is illustrated by Fig.6. Fig.7 shows the case β
i
=
0.3, β
i
= 0.5 where the effect is not seen.
DISCRETE-TIME LQG CONTROL WITH ACTUATOR FAILURE
519