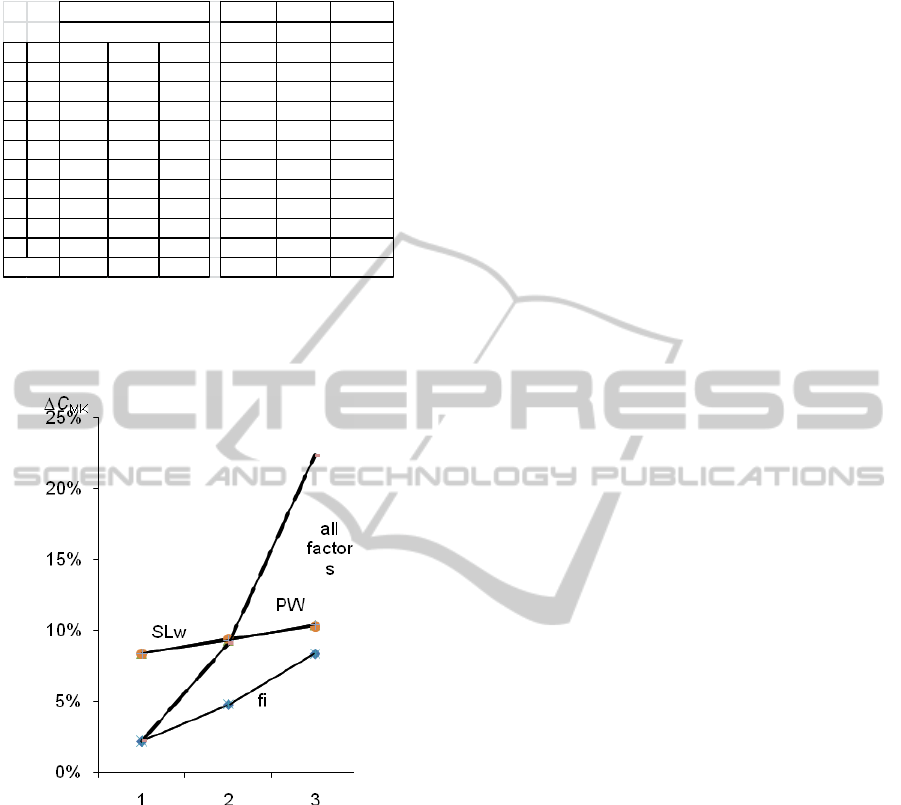
Table 3a: variable f
i
3b: all factors are variable.
fi=4 fi=2 fi=1
PW=10% PW=3 0% PW=50%
ig 4 2 1
Slw=1.1SLw=1.3 SLw=1.5
3 3 1,2% 2,1% 3,3% 1,2% 3,4% 13,5%
4 3 0,3% 1,5% 3,5% 0,3% 4,8% 15,7%
4 4 1,5% 3,3% 5,9% 1,5% 7,0% 18,1%
5 5 1,4% 3,6% 6,2% 1,4% 6,6% 17,0%
5 6 1,6% 2,6% 6,2% 1,6% 5,8% 17,6%
6 5 1,9% 4,8% 8,2% 1,9% 8,0% 18,8%
6 6 2,3% 4,7% 8,0% 2,3% 8,8% 20,9%
8 8 2,3% 4,5% 8,6% 2,3% 8,8% 20,7%
8 10 3,2% 6,3% 10,8% 3,2% 12,4% 28,5%
10 10 2,7% 6,6% 10,7% 2,7% 11,9% 28,2%
averag
2,2% 4,8% 8,4% 2,2% 9,1% 22,3%
PW=10% SLw=1.1
fi
the three factors is significantly higher and ranges
between 2.2% and 22.3%. The averaged results
presented Tables 2 and 3 are graphically shown in
Figure 2.
Figure 2: Influence of factors.
6 CONCLUSIONS
A permutational flowshop group scheduling problem
(GSP) with sequence dependent set-up times, limited
interoperational buffer capacity, workers with
different skills and different mix of the working
crew has been taken into account. In the model, the
set-up times depend on both the sequence of groups
and the worker skill level; the working times have
been considered independent by the skill of the
operator because the working operations are
completely automated. A Genetic Algorithm has
been proposed as an efficient tool to solve the
investigated problem with respect to the
minimization of the total completion time. A
sensitivity analysis has been carried out on a
benchmark of problems to show the relevant
influence of all factors considered in the model. A
future development of this research will treat the
scheduling of jobs as well as the workers assignment
strategy to each machine as independent variables of
the optimization problem.
REFERENCES
Diginesi S.; Kock A., Mummolo G., Rooda J., (2009). The
effect of dynamic worker behavior on flow line
performance. International Journal of Production
Economics, 120 (2), 368-377.
Fowler J., Wirojanagud P., Gel E., (2008). Heuristics for
workforce planning with worker differences.
European Journal of Operational Research, 190 (3),
724-740.
França, P. M., Gupta, J. N. D., Mendes, A. S., Moscato,
P., Veltink, K. J., (2005). Evolutionary algorithms for
scheduling a flowshop manufacturing cell with
sequence dependent family set-ups. Computers &
Industrial Engineering, 48 (3), 491-506.
McDonald T., Ellisb K., Van Akenb E., Koellingb C.,
(2009). Development and application of a worker
assignment model to evaluate a lean manufacturing
cell. International Journal of Production Research, 47
(9), 2427–2447.
Nowicki, E., (1999). The permutation flow shop with
buffers. A tabu search approach. European Journal of
Operational Research, 116, 205-219.
Qian, B., Wang, L., Huang, D., Wang, W., Wang, X.,
(2009). An effective hybrid DE-based algorithm for
multi-objective flow shop scheduling with limited
buffers. Computers & Operations Research, 36, 209-
233.
Schaller, J. E., (2000). A comparison of heuristics for
family and job scheduling in a flow-line
manufacturing cell. International Journal of
Production Research, 38(2), 287-308.
Shridar, J., Rajendran, C., (1994). A genetic algorithm for
family and job scheduling in a flow-line
manufacturing cell. Computers and Industrial
Engineering, 27, 469-472.
Vakharia, A. J., Chang, Y. L, (1990). A simulated
annealing approach to scheduling a manufacturing
cell. Naval Research Logistics, 37, 559-577.
Wang, L., Zhang, L., Zheng, D. Z., (2006). An effective
hybrid genetic algorithm for flow shop scheduling
with limited buffers. Computers & Operations
Research, 33, 2960-2971.
Wemmerlov, U., Vakharia, A. J., (1991). Job and family
scheduling of a flow-line manufacturing cell: a
simulation study. IIE Transactions, 23(4), 383-392.
FLOW SHOP GROUP SCHEDULING WITH LIMITED BUFFER CAPACITY AND DIFFERENT WORKFORCE
491