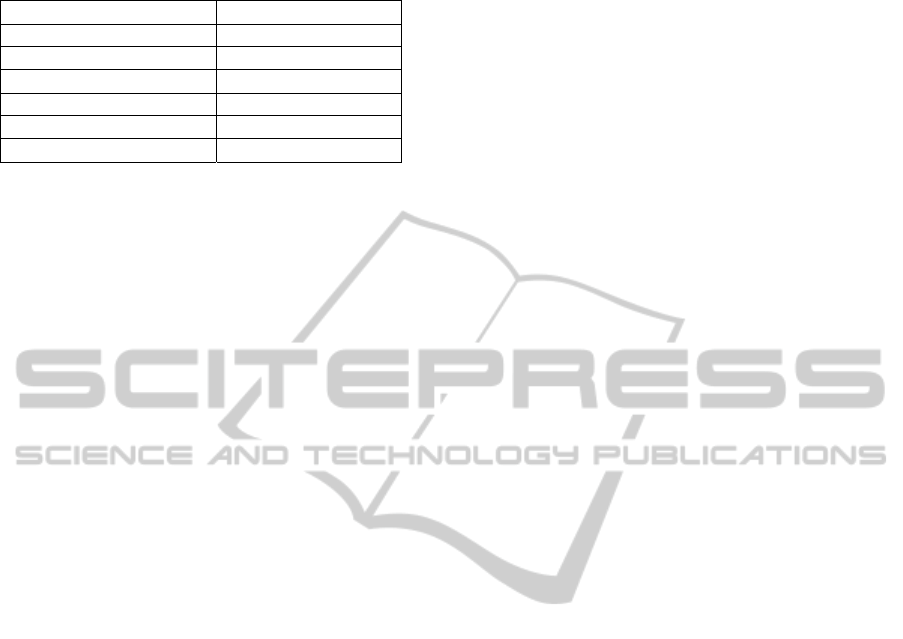
Table 3: Classification Results for Adult Male Sequence
Starting at Epoch with Extended Wrong Classification.
Method Correct Classification
Raw Data .6291
Discounted Dempster-Shafer .7549
Kalman (High Gain) .6725
Kalman (Medium Gain) .6920
Kalman (Low Gain) .7961
Kalman (Ultra-low Gain) .8568
In summary, the proposed Kalman filter-based
temporal evidence accumulation algorithm
outperformed the traditional Dempster-Shafer
algorithm on all three of the datasets in this real-
world application from an automotive airbag
suppression system.
7 CONCLUSIONS AND FUTURE
WORK
We have introduced the notion that when integrating
evidence from a temporal stream of sensor inputs, an
approach based on estimation theory and human
reasoning provides superior performance to a
traditional evidential reasoning approach based on
Dempster-Shafer. We posited that this is due to the
fact that the Dempster-Shafer approach is based on
the concept of evidential independence which
mandates the data be derived from different sensors
(as originally envisioned by Dempster) and that for a
single sensor a weaker statistical independence is all
that can be assured.
We reviewed various approaches for evidence
accumulation. We then developed an alternative
Kalman filter representation from first principles and
identified the key uncertainty terms as being: the
estimate uncertainty:
k
E
2
ˆ
, the measurement
uncertainty:
2
M
, and the system uncertainty:
k
h
2
.
We proposed that the concept of conflict in the
incoming evidential states can be used as a means of
estimating the system uncertainty. The approach was
tested on a real-world automotive airbag suppression
application which consisted of a high resolution
camera providing real-time classification inputs to
our evidence accumulation system. An ultra-low
gain Kalman filter out-performed the traditional
Dempster-Shafer algorithm, which parallels the
findings from human cognition where long term
accumulation of evidence is best considered an
estimation technique and recent evidence is highly
discounted in favour of the historical accumulation
of evidence.
REFERENCES
Baratgin, J., & Politzer, G. (2007). The psychology of
dynamic probability judgment: order effect, normative
theories, and experimental methodology. Mind &
Society , 53–66.
Benferhet, S., Dubois, D., & Prade, H. (2000). Kalman-
like filtering in a qualitative setting. INRIA.
Dubois, D., & Prade, H. (1997). A synthetic view of belief
revision with uncertain inputs in a framework of
possibility theory. International Journal of
Approximate Reasoning , 295-324.
Farmer, M. E. (2006). Integrating temporal streams of
image classifications using evidential reasoning. PRoc.
of Conference on Electro-information Technology (pp.
360-365). IEEE.
Farmer, M. E., & Reiman, J. (2006). Fusion of motion
information with static classifications of occupant
images for smart airbag applications. Proc. Intl.
Conference on Information Fusion (pp. 1-8). IEEE .
Gelb, A. (1974). Applied Optimal Estimation. Cambridge:
MIT Press.
Hogarth, R. M., & Einhorn, H. J. (1992). Order effects in
belief updating: The belief-adjustment model.
Cognitive Psychology , 1-55.
Liu, W., & Hong, J. (2000). Re-investigating Dempster’s
idea on evidence combination. Knowledge and
Information Systems , 223-241.
McKenzie, C. R., Lee, S. M., & Chen, K. K. (2002). When
negative evidence increases confidence: Change in
belief after hearing two sides of a dispute. Journal of
Behavioral Decision Making , 1-18.
Premaratne, K., Dewasurendra, D. A., & Bauer, P. H.
(2007). Evidence combination in an environment with
heterogeneous sources. IEEE. Trans on Systems, Man,
and Cybernetics-Part A: Systems and Humans , 298-
309.
Schubert, J. (2008). Conflict management in Dempster-
Shafer theory by sequential discounting using the
degree of falsity. Procs. of Intl. Conf. on Information
Processing and Management of Uncertainty in
Knowledge-based Systems, (pp. 298-305).
Shafer, G. (1976). A Mathematical Theory of Evidence.
Princeton: Princeton University Press.
Wang, H., Zhang, J., & Johnson, T. R. (1999). Order
effects in human belief revision. Proceedings of the
1999 Cognitive Science Society Conference.
Wu, H., Siegel, M., & Ablay, S. (2003). Sensor fusion
using Dempster-Shafer theory II: Static weighting and
Kalman filter-like dynamic weighting. Procs. of
Instrumentation and Measurement Technology
Conference (pp. 907-912). IEEE.
ICINCO 2011 - 8th International Conference on Informatics in Control, Automation and Robotics
476