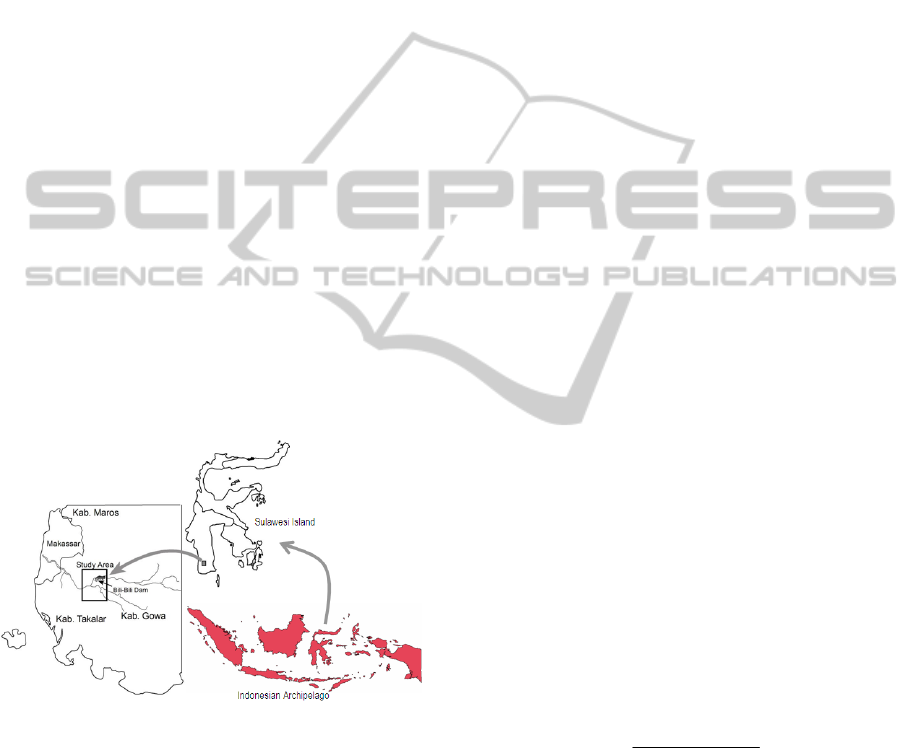
characteristics and crop yields on specified farmland
management in a given study region. The primary
aim of this study is to implement available fuzzy
modeling approaches in a spatial context, and to
assess and map the spatial distribution of corn (Zea
mays L.) yields in the field in relation to land
suitability indices. Geographic information Systems
(GIS) technology, was employed in synchrony with
global positioning system (GPS).
2 METODOLOGY
2.1 Study Area
The area selected for this study includes some parts
of the lower Jeneberang River catchment covering
an area of approximately 37.000 ha, located about
30 km Southeast of Makassar City, South Sulawesi,
Indonesia (Figure 1). According to existing land use
map, agriculture is the predominant land use in the
study region consisting of paddy field 16,725 ha
(45%), followed by shrubs 9,335 ha (25%), mixed
farms 5,071 ha (14%), forest 4,087 ha (11%), water
body (Bili-Bili Dam) 1,766 ha (5%), and residential
379 ha (1%). It was found in the study area that in
addition to rice, rainfed paddy field is also cultivated
with corn.
Figure 1: Location of study area.
2.2 Identification of Land under Corn
Cultivation
Identification of land under traditional corn
cultivation in the study area was undertaken during
cultivation period (March to April 2009). As many
of 31 farmers of corn cultivars from different
villages were involved in this study. These farms
were taken from different land units and identified
as having different land characteristics. At the same
time, soil samples with precise GPS records were
taken from different units for laboratory analysis. An
informal agreement was made between our
surveyors with these farmers to harvest the crops
together (in May and June), in order the corn yields
can be further weighted in kg/ha.
2.3 Calculating Land Suitability
Indices
A fuzzy set is most commonly used for
classifications of objects or phenomena in
continuous values, where the classes do not have
sharply defined boundaries. It deals with a class with
a continuum of grades of memberships (Zadeh,
1965). A fuzzy set A may be defined as follows:
A = {x,
A
(x)} x X
(1)
Where X = {x} is a finite set (or space) of objects or
phenomena,
A
(x) is a membership function of X for
subset A.
Therefore, a fuzzy subset is defined by the
membership function (MF) that defines the
membership grades of fuzzy objects or phenomena
in the ordered pairs, consisting of the objects and
their membership grades. The MF of a fuzzy subset
determines the degree of membership of x in A
(Burrough et al., 1992).
Calculation procedure implemented in this study
utilizes an a priori membership function (MF) for
individual variables under consideration, where the
technique is called “a Semantic Import” (SI) model
(Burrough and McDonnel, 1998). Examples can be
seen in Baja et al. (2002a) and Davidson et al.
(1994). With this approach, the attribute values
considered are converted to common membership
grades (from 0 to 1.0), according to the class limits
specified by the analysts based on experience or
conventionally imposed definitions.
If MF(x
i
) represents individual MF values for i
th
land property x, then, the basic SI model function
take the following form in the computation process:
}]/)[(1{
1
)(
2
dbx
xMF
i
i
(2)
In the computation, it is crucial to examine an
appropriate fuzzy model parameter to suit each
decision criterion. The choice depends on the ‘trend
of performance’ of the respective land attribute in
accommodating a favorable condition for a selected
land use type (Baja et al., 2002b). Model parameters
include LCP (lower crossover point), b (central
concept), UCP (upper crossover point), and d (width
of transition zone).
Land and climate characteristics used for
FCTA 2011 - International Conference on Fuzzy Computation Theory and Applications
436