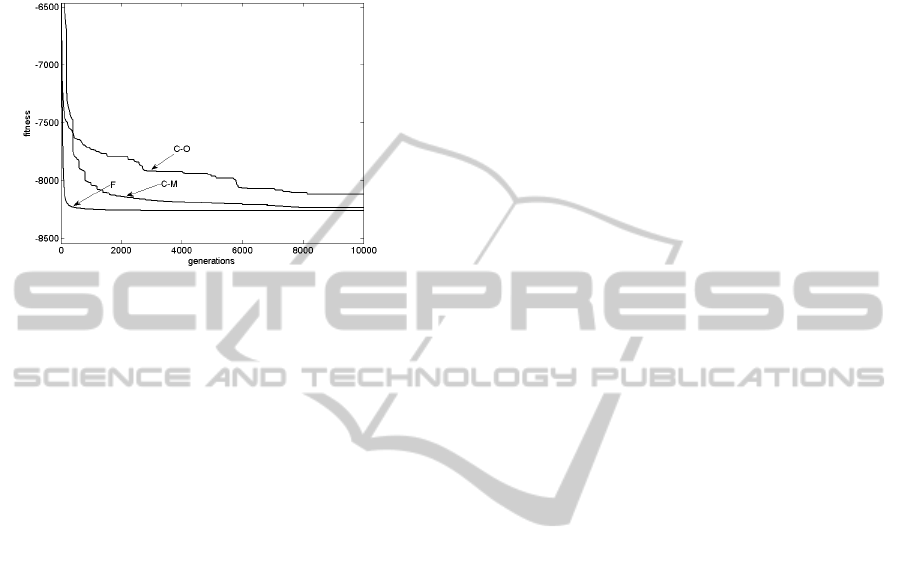
The last figure (Fig.18) depicts the comparison of
the best representatives of all PGA architectures.
The fastest convergence in case of the Eggholder
function has been achieved by the fine-grained
(cellular) PGA.
Figure 18: Comparison of various PGA types: Coarse-
grained migration-based PGA (C-M), Coarse-grained
overlapping-based PGA (C-O), Fine-grained PGA (F).
5 CONCLUSIONS
Selected types of parallel genetic algorithms have
been experimentally compared and the influence of
some of their parameters analyzed. We have
considered various architectures, various topologies
of migration connections, influence of changing
migration period, population size and number of
islands. In our experiments using the Eggholder
function the fine-grained PGA performance
outperforms the coarse-grained PGA. However, the
performance of all PGA types depends on their
parameters as well as on the problem to be solved.
In our comparison in each island of the PGA the
genetic algorithm with equal parameters has been
considered. Such "homogenous" algorithms have the
same diversity and selection pressure in all
subpopulations. Based on our experiments for such
type of PGA (PEA) we can make following
conclusions. Changing of migration topology has a
small influence on the PGA performance. The main
influence on the performance has the migration
period, subpopulation size and number of islands.
These factors affect the ability of all subpopulations
of the PGA to evolve perspective genes and building
blocs and effectively to explore the search space.
For each problem solved it is important to find a
balance between the "independence" of each
subpopulation for their evolution and diversity in
each subpopulation on the one side and the
communication and exchange of perspective genetic
information between subpopulations or individuals
on the other side.
The presented experimental study is a part of a
project, which should help to generalise the
properties and behaviour of various types of
parallel evolutionary algorithms and help to design
algorithms for solving hard search/optimisation
problems like modelling of bio-medicine processes,
optimisation of large technological and construction
tasks, solving of economical and financial problems
etc.
ACKNOWLEDGEMENTS
This work was supported by the Slovak Research
and Development Agency under the contract No.
APVV-0523-10.
REFERENCES
Alba E., Tomassini M., 2002. Parallelism and
Evolutionary Algorithms. In IEEE Trans. on
Evolutionary Computation, Vol. 6, NO.5.
Cantú-Paz E., 1995. A Summary of Research on Parallel
Genetic Algorithms. In IlliGAL Report No. 95007.
University of Illinois at Urbana-Champaign.
Cantú-Paz E., 1999. Migration Policies, Selection
Pressure, and Parallel Evolutionary Algorithms. In
IlliGAL Report 99015, University of Illinois at
Urbana-Champaign.
Giacobini M., Tomassini M., Tettamanzi A.G.B., Alba E.,
2005. Selection intensity in cellular evolutionary
algorithms for regular lattices. In IEEE Transactions
on Evolutionary Computation.
Lin S. Ch., Punch W., Goodman E., 1994. Coarse-grain
parallel genetic algorithms: Categorization and new
approach. In IEEE Symposium on Parallel and
Distributed Processing.
Nowostawski M., Poli R., 1999. Parallel Genetic
Algorithm Taxonomy. In KES’99.
Sekaj I., 2004. Robust Parallel Genetic Algorithms with
Re-Initialisation. In PPSN VIII, September 18-22,
Birmingham.
Sekaj I., Perkacz J., 2007. Some Aspects of Parallel
Genetic Algorithms with Population Re-initialization.
In CEC, Singapore.
Sekaj, I., Oravec, M., 2009. Selected Population
Characteristics of Fine-grained Parallel Genetic
Algorithms with Re-initialisation. In Proceedings of
the GEC 2009, Shanghai.
Skolicki Z., DeJong K., 2005. The influence of migration
sizes and intervals on island models. In GECCO,
Washington, USA.
Whitley D., Rana S., Heckendorn R. B.,1999. The island
model genetic algorithm: On separability, population
size and convergence. In Journal of Computing and
Information Technology, 7(1), pp.33-47.
ECTA 2011 - International Conference on Evolutionary Computation Theory and Applications
302