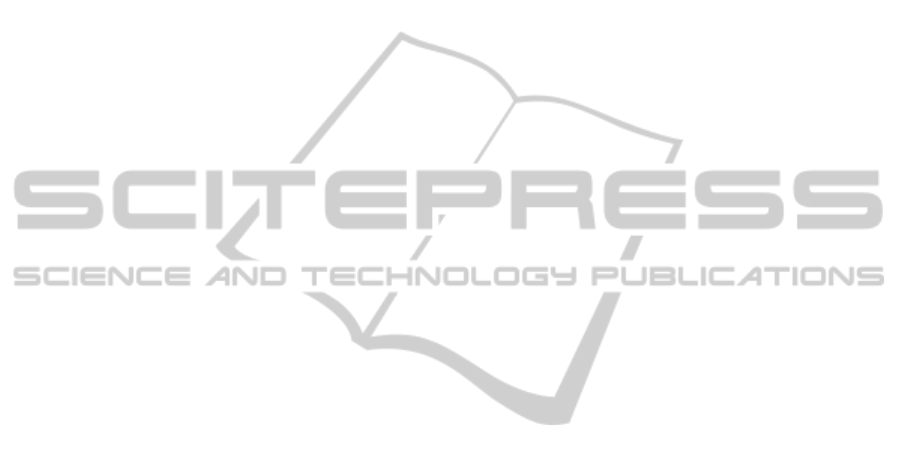
Dynamics in Government Extended Enterprises:
Essential Concepts,” Proceedings of the 2011 IEEE
International Systems Conference, IEEE.
John, L., P. McCormick, T. McCormick, G. McNeill, J,
Boardman (2011b). “Self-Organizing Cooperative
Dynamics in Government Extended Enterprises:
Experimental Methodology,” Proceedings of the 6th
IEEE International Systems Conference, IEEE.
Lopes, L. (1987). “Between Hope and Fear: The
Psychology of Risk.” Advances in Experimental Social
Psychology, vol. 20, pp. 255-295.
Mertikopoulos, P. and A. L. Moustakas (2010). “The
Emergence of Rational Behavior in the Presence of
Stochastic Perturbations,” The Annals of Applied
Probability, 20, pp. 1359-1388.
Miller, J. and S. Page (2007). Complex Adaptive Systems:
An introduction to Computational Models of Social
Life, Princeton MJ: Princeton University Press, 2007.
Miner, J. B. (2006). Organizational Behavior 2: Essential
Theories of Process and Structure, Armonk, NY: M. E.
Sharpe, Inc., pp.109-126.
Miner, J. B. (2005). Organizational Behavior 1: Essential
Theories of Motivation and Leadership, Armonk, NY:
M. E. Sharpe, Inc., pp. 94-113.
“need,” OED (2009). Oxford English Dictionary (2nd
Edition) Online, Oxford: Oxford University Press,
retrieved 7 October 2009.
Ostrom, E. (2005). Understanding Institutional Diversity,
Princeton: Princeton University Press.
Ostrom, E. (2007) “An Assessment of the Institutional
Analysis and Development Framework,” in Sabatier,
Paul A. (ed.) Theories of the Policy Process Second
Edition, Boulder, CO: Westview Press.
Ostrom, E (2010). Personal communication, “Request for
Opinion on Subtractability of Value as a replacement
for Subtractability of Flow in Information Exchanges,”
Fri 6/25/2010 8:06 AM.
Ostrom, E., R. Gardner, and J. Walker (1994). Rules,
Games, and Common-Pool Resources, Ann Arbor:
University of Michigan Press.
Ostrom, V. (2005). “Citizen Sovereigns: The Source of
Contestability, the Rule of Law, and the Conduct of
Public Entrepreneurship,” PS: Political Science and
Politics, (January), pp. 13-17.
Pacheco, J. M., F. C. Santos, M. O. Souze, B. Skyrms
(2009). “Evolutionary dynamics of collective action in
n-person stag hunt dilemmas,” Proceedings of the
Royal Society Biological Sciences, (January 22), 276
(1655), pp. 315-321.
Parsons, T. and E. A. Shils (2001). Toward a General
Theory of Action: Theoretical Foundations for the
Social Sciences, New Brunswick, NJ: Transactions
Publishers, p.134.
Poteete, A. R., M. A. Janssen and E. Ostrom (2010).
Working Together: Collective Action, the Commons,
and Multiple Methods in Practice, Princeton: Princeton
University Press.
Prange, G. W. (1981). At Dawn We Slept, New York:
McGraw-Hill.
Priest, D. and W. M. Arkin (2010). “Top Secret America:
A Washington Post Investigation,” accessed 10 Feb
2011 at http://projects.washingtonpost.com/top-secret-
america/articles/a-hidden-world-growing-beyond-
control.
Ridings, C. M., D. Gefen, and B. Arinze (2002). “Some
antecedents and effects of trust in virtual
communities,” Journal of Strategic Information
Systems, 11, pp. 271-295.
SEAS (2010). “What is SEAS?” http://teamseas.com/
content/view/55/71/, accessed 12 October 2010.
Shefrin, H. (2002). Beyond Greed and Fear, New York:
Oxford University Press, pp. 120-121.
Shor, M. (2010a). "Prisoners’ Dilemma," Dictionary of
Game Theory Terms, Game Theory.net, http://www.
gametheory.net/dictionary/games/PrisonersDilemma.ht
ml accessed: 12 October 2010.
Shor, M. (2010b). "Stag Hunt," Dictionary of Game
Theory Terms, Game Theory.net, www.gametheory.
net/dictionary/games/StagHunt.html accessed: 12
October 2010.
Simon, H. A. (1990). “Invariants of Human Behavior,”
Annual Review of Psychology, 41, pp. 1-19.
Simon, H. A. (1997 [1945]). Administrative Behavior: A
Study of Decision-Making Processes in Administrative
Organizations, Fourth Edition, New York: The Free
Press, pp 206-207.
Skinner, B. F. (1965). Science and Human Behavior, New
York: The Free Press, 1965, p. 361.
Skyrms, B. (2004). The Stag Hunt and the Evolution of
Social Structure, Cambridge: Cambridge University
Press.
Smith, A. (1790). The Theory of Moral Sentiments (6th
Edition), London: A. Millar, http://www.econlib.org/
library/Smith/smMS1.html.
SSCI (2010). Senate Select Committee on Intelligence,
“Unclassified Executive Summary of the Committee
Report on the Attempted Terrorist Attack on Norwest
Airlines Flight 253,” Senate Select Committee on
Intelligence, May 18, 2010.
Stamper, R., K. Liu, M. Hafkamp and Y. Ades (2000).
“Understanding the Roles of Signs and Norms in
Organizations – a Semiotic Approach to Information
Systems Design,” Behaviour and Information
Technology, 19(1), pp. 15-27.
“trust,” OED (2009). Oxford English Dictionary (2nd
Edition) Online, Oxford: Oxford University Press,
retrieved 7 October 2009.
“uncertainty,” OED (2009). Oxford English Dictionary
(2nd Edition) Online, Oxford: Oxford University Press,
retrieved 7 October 2009.
von Bertalanffy, L. (1950). “The Theory of Open Systems
in Physics and Biology,” Science, III, 2872, pp.23-29.
Wiener, N. (1948), Cybernetics. New York: Wiley.
Williams, J. (2002). In Cynthia Grabo, Anticipating
Surprise: Analysis for Strategic Warning, Washington
DC: Center for Strategic Intelligence Research, Joint
Military Intelligence College.
Windrum, P., G. Fagiolo, and A. Moneta (2007).
“Empirical Validation of Agent-Based Models:
Alternatives and Prospects,” Journal of Artificial
IMPACT OF BEHAVIORAL FORCES ON KNOWLEDGE SHARING IN AN EXTENDED ENTERPRISE SYSTEM OF
SYSTEMS
75