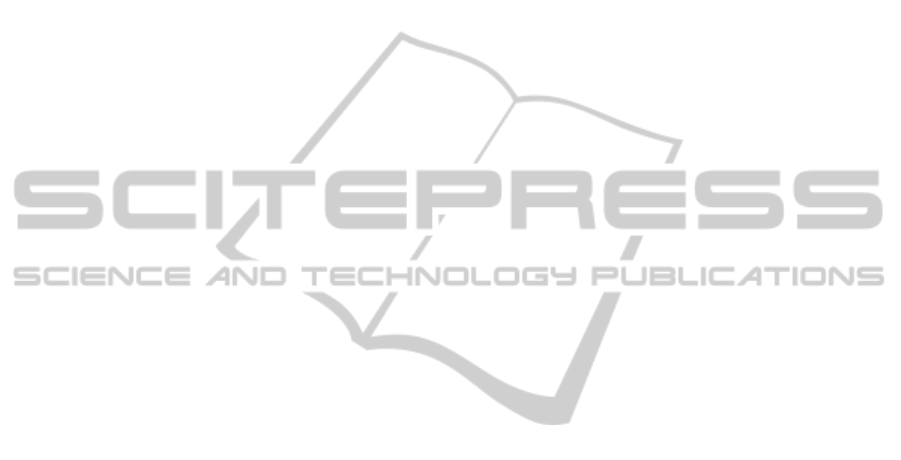
require a finer-grained analysis and additional itera-
tions of the conceptualisation process, but most of the
related terms occur in the contexts of the mentions of
existing concepts.
Both use-cases show that named entities help the
detection of relevant domain concepts. Since the list
of named entities extracted is much smaller than the
list of terms (more than 10 times smaller in our use-
cases), it is interesting to rely on named entities as
a starting step, even if the list of textual units must
then be further explored to enrich the draft ontologies
based on named entities.
6 CONCLUSIONS
This paper shows how text-based ontology building
methods can be enriched by taking specific textual
units into account – named entities as well as terms
– and explains how named entities can be used in the
conceptualisation task. We show that they can be used
either to enrich an ontology (building new concepts,
their properties, re-structuring and populating exist-
ing concepts) or for bootstrapping the conceptualisa-
tion step and identifying relevant domain terms.
This combined approach, which is implemented
in the new TERMINAE tool, is illustrated on two use-
cases. Even if named entities are not as numerous in
policies as in press articles for instance, they are im-
portant to take into account in the conceptualisation
process because they point out critical domain ele-
ments that are important to integrate in a conceptual
model.
ACKNOWLEDGEMENTS
This work was realised as part of the FP7 231875 ON-
TORULE project (http://ontorule-project.eu). We are
grateful to American Airline who is the owner of one
of our working corpora.
REFERENCES
Aussenac-Gilles, N., Bourigault, D., Condamines, A., and
Gros, C. (1995). How can knowledge acquisition ben-
efit from terminology? In Proceedings of the 9th
Knowledge Acquisition Workshop.
Aussenac-Gilles, N., Despr/’es, S., and Szulman, S. (2008).
The terminae method and platform for ontology engi-
neering from texts. In Buitelaar, P. and Cimiano, P.,
editors, Bridging the Gap between Text and Knowl-
edge, pages 199–223. IOS Press.
Cimiano, P. and V
¨
olker, J. (2005). Text2onto - a framework
for ontology learning and data-driven change discov-
ery. In Proc. of the 10th Int. Conf. on Applications
of Natural Language to Information Systems, pages
227–238.
Faure, D. and N
´
edellec, C. (1999). Knowledge acquisi-
tion of predicate argument structures from technical
texts using machine learning: the system asium. In
et R. Stude, D. F., editor, Proc. of the 11th Int. Conf.
on Knowledge Engineering and Knowledge Manage-
ment (EKAW’99), pages 329–334. Springer-Verlag.
Giuliano, C. and Gliozzo, A. (2008). Instance-based ontol-
ogy population exploiting named-entity substitution.
In Proc. of the 22nd Int. Conf. on Computational Lin-
guistics (Coling 2008), pages 265–272, Manchester.
LDC (2004). Ace (automatic content extraction) english an-
notation guidelines for entities. Livrable version 5.6.1
2005.05.23, Linguistic Data Consortium.
Lopes, L. and Vieira, R. (2009). Automatic extraction of
composite terms for construction of ontologies: an
experiment in the health care area. Electronic Jour-
nal of Communication, Information and Innovation in
Health, 3(1):72–84.
Magnini, B., Pianta, E., Popescu, O., and Speranza, M.
(2006). Ontology population from textual mentions:
Task definition and benchmark. In Proc. of the OLP2
workshop on Ontology Population and Learning.
Maynard, D., Li, Y., and Peters, W. (2008). NLP tech-
niques for term extraction and ontology population.
In Buitelaar, P. and Cimiano, P., editors, Bridging the
Gap between Text and Knowledge, pages 199–223.
IOS Press.
Meyer, I., Skuce, D., Bowker, L., and Eck, K. (1992). To-
wards a new generation of terminological resources
: an experiment in building a terminological knowl-
edge base. In Proc. of the 15th Int. Conf. on Com-
putational Linguistics (COLING’92), pages 956–960,
Nantes, France.
Morita, T., Fukuta, N., Izumi, N., and Yamaguchi, T.
(2008). Doddle-owl: Interactive domain ontology de-
velopment with open source software in java. IEICE
Transactions on Information and Systems, 4:945–958.
Nadeau, D. and Sekine, S. (2007). A survey of named entity
recognition and classification. Linguisticae Investiga-
ciones, 30(1):3–26.
Studer, R., Benjamins, V. R., and Fensel, D. (1998). Knowl-
edge engineering: Principles and methods. Data
Knowl. Eng., 25:161–197.
Wang, Y., Volker, J., and Haase, P. (2006). Towards semi-
automatic ontology building supported by large-scale
knowledge acquisition. In AAAI Fall Symposium On
Semantic Web for Collaborative Knowledge Acquisi-
tion, volume FS-06-06, pages 70–77, Arlington, VA,
USA. AAAI, AAAI Press.
KEOD 2011 - International Conference on Knowledge Engineering and Ontology Development
254