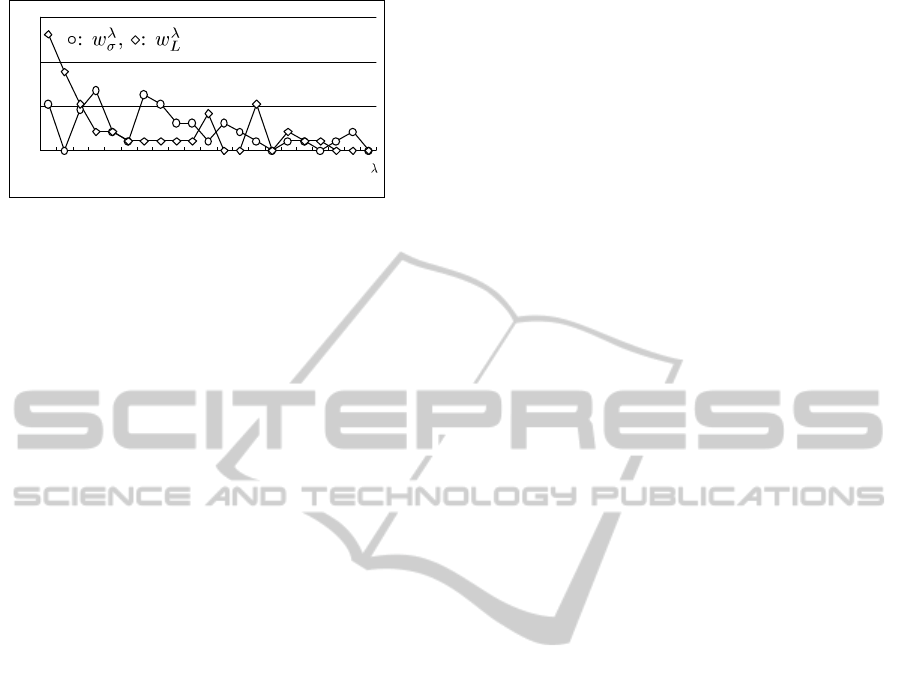
0
0.1
0.2
0.3
0
1
0
0.1
0.2
0.3
0
1
Figure 4: Winning rates of different λs in NMI.
of community discovery. Thus, we can confirm that
the appropriate parameter setting heavily depends on
application domain.
4 RELATED WORK
Estimation of user influence attracts much attention
in the area of social network analysis, and many so-
phisticated models are proposed, e.g. (Goyal et al.,
2010; Kimura et al., 2009). However, it is difficult to
apply them directly to proxy logs not having precise
information to construct accurate user networks.
Several methods for estimating user influence
without explicit network information have been de-
veloped recently. In (Gomez Rodriguez et al., 2010),
an algorithm named ‘netinf’ is proposed which esti-
mates hidden network structures from a set of infor-
mation cascades obtained from (proxy) log data. Net-
inf estimates directed unweighted networks of users
by adopting the exponential waiting time model on
information diffusion while it assumes that the de-
gree of user influences are the same among any user
pairs. As an extension of netinf, a convex program-
ming based method for inferring directed weighted
network structures from cascade data has been pro-
posed in (Myers and Leskovec, 2010). While these
two methods employ the exponential waiting time
model for reflecting information on time difference,
they do not consider the importance of contents at all.
A probabilistic model for user adoption behaviors
has been proposed in (Au Yeung and Iwata, 2010).
By using the model, user influence as well as influ-
ences of popularity and recency of contents are esti-
mated from log data. The model requires a parameter
specifying the length of period in which a user affects
others. In other words, behaviors outside of the pe-
riod are regarded to give no effect. On the other hand,
the effects of behaviors decrease gradually with time
in our proposal.
5 CONCLUSIONS
In this paper, we propose a framework for estimat-
ing implicit user influence from proxy logs. We
model user interactions as vectors by taking account
of the difference of access time and importance of
web pages, and use the vectors to estimate the influ-
ence. The proposed methods are evaluated empiri-
cally by using three real datasets in the tasks of web
page recommendation and community discovery.
For future work, detailed assessments of obtained
user influences are necessary. In addition, we plan to
investigate further experiments with large-scale proxy
logs having different characteristics as well as pre-
cise comparisons with related techniques on estimat-
ing user influence.
REFERENCES
Au Yeung, C.-m. and Iwata, T. (2010). Capturing implicit
user influence in online social sharing. In Proceedings
of the 21st ACM Conference on Hypertext and Hyper-
media, pages 245–254.
Chang, C.-C. and Lin, C.-J. (2001). LIBSVM: a library
for support vector machines. Software available at
http://www.csie.ntu.edu.tw/˜cjlin/libsvm
.
Csardi, G. and Nepusz, T. (2006). The igraph software
package for complex network research. InterJournal,
Complex Systems:1695.
Danon, L., D´ıaz-Guilera, A., Duch, J., and Arenas, A.
(2005). Comparing community structure identifica-
tion. Journal of Statistical Mechanics: Theory and
Experiment, 2005(9):P09008.
Gomez Rodriguez, M., Leskovec, J., and Krause, A. (2010).
Inferring networks of diffusion and influence. In
Proceedings of the 16th ACM SIGKDD International
Conference on Knowledge Discovery and Data Min-
ing, pages 1019–1028.
Goyal, A., Bonchi, F., and Lakshmanan, L. V. (2010).
Learning influence probabilities in social networks. In
Proceedings of the third ACM International Confer-
ence on Web Search and Data Mining, pages 241–250.
Kimura, M., Saito, K., and Motoda, H. (2009). Efficient
estimation of influence functions for sis model on so-
cial networks. In Proceedings of the 21st International
Joint Conference Artificial Intelligence, pages 2046–
2051.
McPherson, M., Lovin, L. S., and Cook, J. M. (2001). Birds
of a Feather: Homophily in Social Networks. Annual
Review of Sociology, 27(1):415–444.
Myers, S. and Leskovec, J. (2010). On the convexity of
latent social network inference. In Advances in Neu-
ral Information Processing Systems 23, NIPS, pages
1741–1749.
Newman, M. E. J. and Girvan, M. (2004). Finding and eval-
uating community structure in networks. Physical Re-
view E, 69(2):026113.
ESTIMATION OF IMPLICIT USER INFLUENCE FROM PROXY LOGS - An Empirical Study on the Effects of Time
Difference and Popularity
255