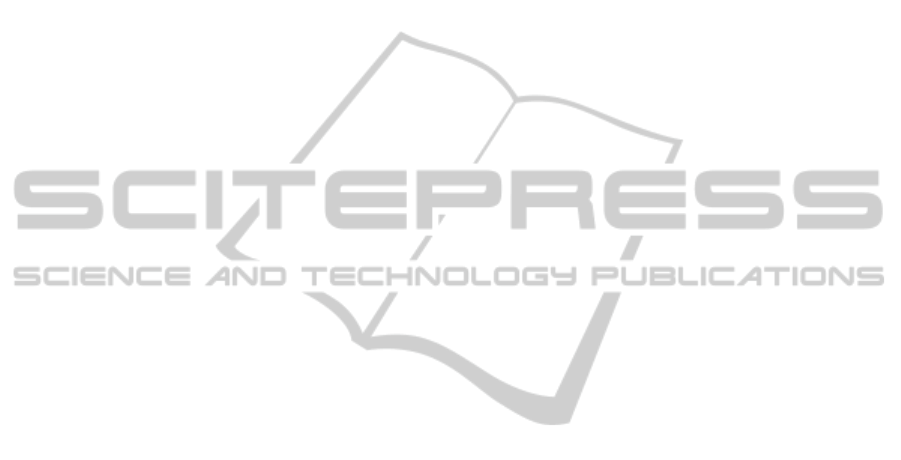
sults on KBP 2010 have shown that the overall perfor-
mance could be improved without such complemen-
tary resource and that the effect of such process on
final results were lower compared to KBP 2009 (we
even observe a negative impact).
5 CONCLUSIONS AND
PERSPECTIVES
In this article, we present an information extraction
system designed for the large-scale extraction of at-
tribute relations between named entities. The “large-
scale” qualification is meant for both the integration
of a large number of types of relations and the search
of these relations in a large corpus. This system is
based on a weakly supervised approach in which the
examples are limited to pairs of entities in relation.
The extraction of relations is performed by the ap-
plication of lexico-syntactic patterns that are learned
from occurrences of relations automatically selected
from the entity pairs of the examples and used to rep-
resent the relation types. We evaluate our approach
using the evaluation framework from the Slot Fill-
ing task of the KBP evaluation campaign, concentrat-
ing on the problem of relation extraction itself (we
did not consider the case where the relation is not
present in the target corpus). The results obtained in
this context are comparable to the results obtained by
the participants of 2010 campaign, which we consider
promising for our system, since it is designed to be
generic and is not tuned to deal with the specificities
of the types of relations used in this campaign. We
also show that specific techniques used to deal with
the large-scale aspect of the task, such as the filter-
ing of the examples with the APSS technique, do not
decrease the performance and can even contribute to
improve it.
We are currently working on the improvement of
our system, trying to keep the idea of a generic sys-
tem with respect to the type of relation considered. In
particular, we focus on the pattern learning step: we
are considering both the use of a more important num-
ber of examples to learn the pattens and the improve-
ment of the quality of the examples. These two points
are connected because, usually, in order to get more
examples, we need to relax a constraint on the selec-
tion of the examples, which will generally increase
the number of false examples. To avoid this draw-
back, we will explore the use of a relation filtering
module which is capable of determining if a sentence
contains a relation between two entities or not with-
out any consideration on the nature of the relation (as
in (Banko and Etzioni, 2008)).
ACKNOWLEDGEMENTS
This work was partly supported by the FP7 Virtuoso
project.
REFERENCES
Agichtein, E. and Gravano, L. (2000). Snowball: Extracting
Relations from Large Plain-Text Collections. In 5
th
ACM International Conference on Digital Libraries,
pages 85–94, San Antonio, Texas, USA.
Agirre, E., Chang, A., Jurafsky, D., Manning, C.,
Spitkovsky, V., and Yeh, E. (2009). Stanford-UBC at
TAC-KBP. In Second Text Analysis Conference (TAC
2009), Gaithersburg, Maryland, USA.
Banko, M. and Etzioni, O. (2008). The Tradeoffs Between
Open and Traditional Relation Extraction. In ACL-08:
HLT, pages 28–36, Columbus, Ohio.
Bayardo, R., Ma, Y., and Srikant, R. (2007). Scaling Up All
Pairs Similarity Search. In 16
th
International Confer-
ence on World Wide Web (WWW’07), pages 131–140,
Banff, Alberta, Canada.
Bikel, D., Castelli, V., Radu, F., and jung Han, D. (2009).
Entity Linking and Slot Filling through Statistical Pro-
cessing and Inference Rules. In Second Text Analysis
Conference (TAC 2009), Gaithersburg, USA.
Bizer, C., Lehmann, J., Kobilarov, G., Auer, S., Becker, C.,
Cyganiak, R., and Hellmann, S. (2009). DBpedia - A
crystallization point for the Web of Data. Journal of
Web Semantics, 7:154–165.
Byrne, L. and Dunnion, J. (2010). UCD IIRG at TAC 2010
KBP Slot Filling Task. In Third Text Analysis Confer-
ence (TAC 2010), Gaithersburg, Maryland, USA.
Chada, D., Aranha, C., and Monte, C. (2010). An Anal-
ysis of The Cortex Method at TAC 2010 KBP Slot-
Filling. In Third Text Analysis Conference (TAC
2010), Gaithersburg, Maryland, USA.
Chen, Z., Tamang, S., Lee, A., Li, X., Passantino, M., and
Ji, H. (2010a). Top-down and Bottom-up: A Com-
bined Approach to Slot Filling. In 6
th
Asia Infor-
mation Retrieval Symposium on Information Retrieval
Technology, pages 300–309, Taipei, Taiwan.
Chen, Z., Tamang, S., Lee, A., Li, X., Snover, M., Pas-
santino, M., Lin, W.-P., and Ji, H. (2010b). CUNY-
BLENDER TAC-KBP2010 Slot Filling System De-
scription. In Text Analysis Conference (TAC 2010),
Gaithersburg, Maryland, USA.
Embarek, M. and Ferret, O. (2008). Learning patterns
for building resources about semantic relations in the
medical domain. In 6
th
Conference on Language Re-
sources and Evaluation (LREC’08), Marrakech, Mo-
rocco.
Gionis, A., Indyk, P., and Motwani, R. (1999). Simi-
larity Search in High Dimensions via Hashing. In
25
th
International Conference on Very Large Data
Bases (VLDB’99), pages 518–529, Edinburgh, Scot-
land, UK.
KDIR 2011 - International Conference on Knowledge Discovery and Information Retrieval
102